Many-task evolutionary algorithm with adaptive knowledge transfer via density-based clustering
KNOWLEDGE-BASED SYSTEMS(2023)
摘要
Evolutionary multitask optimization (EMTO) explores how to solve two or more related optimization tasks simultaneously by exploiting useful knowledge of other related tasks while solving one task, with the goal of enhancing the performance of the algorithm in solving each task. There has been a large body of work in the literature on knowledge transfer and reuse. However, when the number of tasks increases to a certain extent, the potential negative knowledge transfer between tasks becomes a great challenge for most multitask evolutionary algorithms. To allay this threat, this study proposes an adaptive knowledge transfer strategy for evolutionary many-task optimization via density-based clustering (AEMaTO-DC). AEMaTO-DC contains an adaptive mating selection mechanism, a correlation task evaluation and selection mechanism and a cluster-based knowledge interaction mechanism between tasks. Specifically, AEMaTO-DC regulates the probability of knowledge transfer by comparing the relative intensity of the intertask evolution rate and intratask evolution rate. In knowledge transfer, AEMaTO-DC selects related tasks by a maximum mean discrepancy metric, and it determines the intensity of intertask interaction by a density-based clustering method. We compare the performance of AEMaTO-DC with several state-of-the-art multitask evolutionary algorithms on a variety of synthetic benchmarks and a real-world multitask optimization problem. The experimental results demonstrate that AEMaTO-DC shows competitive success rates in solving the many-task problems used, and it takes advantage of synergistic relationships among solution processes for the tasks addressed.(c) 2023 Elsevier B.V. All rights reserved.
更多查看译文
关键词
Many-task optimization,Intertask synergies,Knowledge transfer,Evolutionary multitask optimization,Density-based clustering
AI 理解论文
溯源树
样例
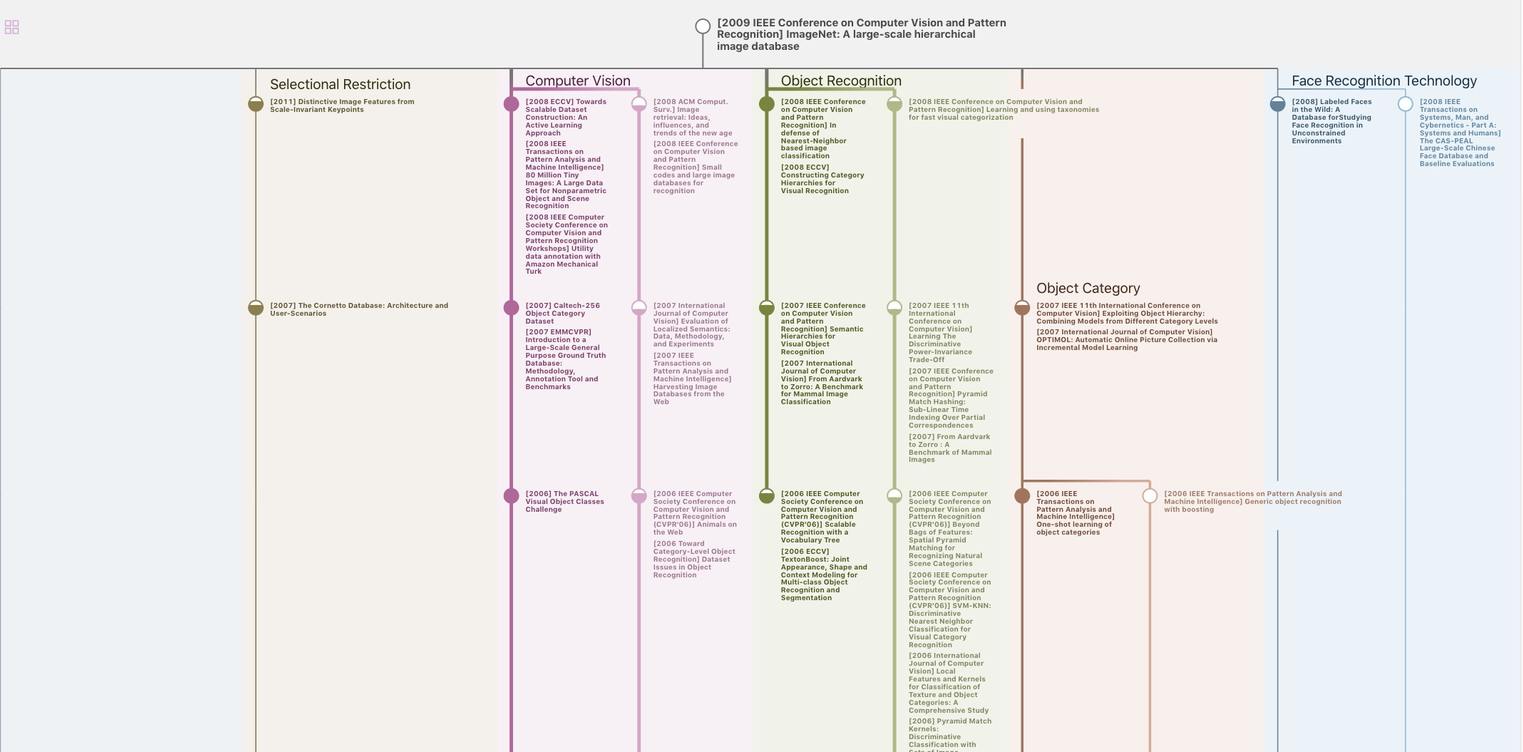
生成溯源树,研究论文发展脉络
Chat Paper
正在生成论文摘要