Multi-Frame Full-Rank Spatial Covariance Analysis for Underdetermined Blind Source Separation and Dereverberation.
IEEE ACM Trans. Audio Speech Lang. Process.(2023)
摘要
Full-rank spatial covariance analysis (FCA) is a technique for blind source separation (BSS), and can be applied to underdetermined situations where the sources outnumber the microphones. This paper proposes multi-frame FCA as an extension of FCA to improve the BSS performance when the room reverberations are not so short that multiple time frames are needed to cover the dominant parts of the reverberations. There has already been proposed an FCA model that considers delayed source components. However, the existing FCA model does not take the correlation between different time frames into account. In contrast, our new extension models multiple time frames with multivariate Gaussian distributions of larger dimensionality than the existing FCA models, aiming to better model the source components spanning multiple time frames. We derive an expectation-maximization (EM) algorithm to optimize the model parameters. Experimental results show that the proposed multi-frame FCA performed clearly better than the existing FCA techniques in BSS tasks and also joint BSS and blind dereverberation tasks.
更多查看译文
关键词
Sensors, Covariance matrices, Gaussian distribution, Speech processing, Reverberation, Analytical models, Probabilistic logic, Blind source separation (BSS), blind dereverberation (BD), full-rank spatial covariance analysis (FCA), expectation-maximization (EM) algorithm, multivariate complex Gaussian distribution, weighted prediction error (WPE)
AI 理解论文
溯源树
样例
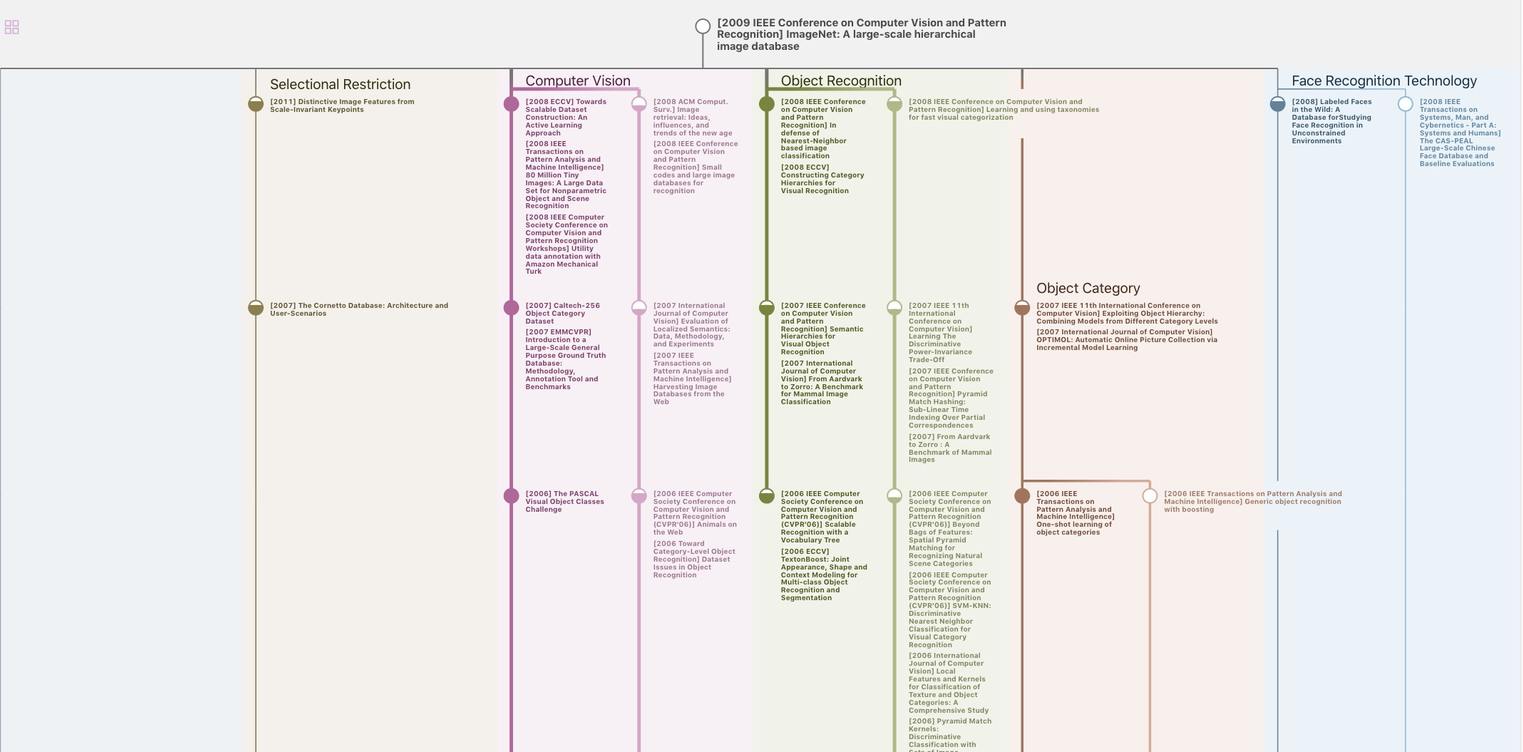
生成溯源树,研究论文发展脉络
Chat Paper
正在生成论文摘要