An improved k-NN anomaly detection framework based on locality sensitive hashing for edge computing environment
Intell. Data Anal.(2023)
摘要
Large deployment of wireless sensor networks in various fields bring great benefits. With the increasing volume of sensor data, traditional data collection and processing schemes gradually become unable to meet the requirements in actual scenarios. As data quality is vital to data mining and value extraction, this paper presents a distributed anomaly detection framework which combines cloud computing and edge computing. The framework consists of three major components: k-nearest neighbors, locality sensitive hashing, and cosine similarity. The traditional k-nearest neighbors algorithm is improved by locality sensitive hashing in terms of computation cost and processing time. An initial anomaly detection result is given by the combination of k-nearest neighbors and locality sensitive hashing. To further improve the accuracy of anomaly detection, a second test for anomaly is provided based on cosine similarity. Extensive experiments are conducted to evaluate the performance of our proposal. Six popular methods are used for comparison. Experimental results show that our model has advantages in the aspects of accuracy, delay, and energy consumption.
更多查看译文
关键词
Aanomaly detection,k-nearest neighbors,locality sensitive hashing,cosine similarity,edge computing
AI 理解论文
溯源树
样例
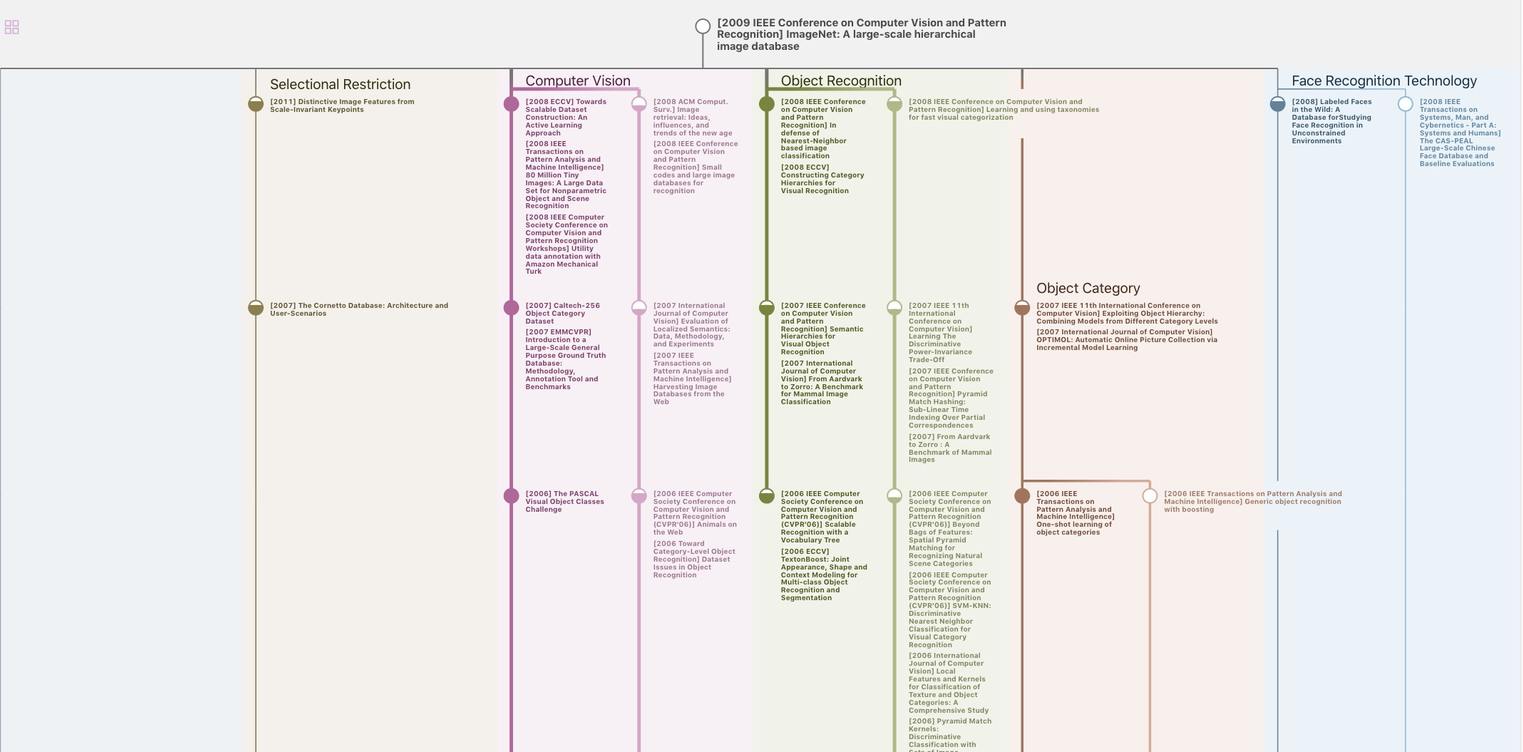
生成溯源树,研究论文发展脉络
Chat Paper
正在生成论文摘要