Consistency-based self-supervised visual tracking by using query-communication transformer
Knowl. Based Syst.(2023)
摘要
Self-supervised learning (SSL) performs remarkably in visual tracking since it enables the extraction of general representations from unlabeled data and alleviates the need for expensive human annotations. SSL models usually achieve frame-to-frame communications during training by predicting each object location of intermediate frames, however, the possible prediction errors may accumulate and mislead the forward-backward tracking procedure. A novel query-communication transformer (QCT) architecture is proposed in this work to enable more reliable frame-to-frame communications via propagating query information, avoiding the above-mentioned tracking errors on intermediate frames tactfully. Specifically, we introduce the transformer into self-supervised tracking to handle the object template and search frames, i.e., the encoder encodes spatio-temporal context of template and search frames, while the decoder takes the query embedding of previous frame to retrieve the template object information from the encoder output. To further enhance the query embedding, a query interaction module is devised to promote information passing between frames. Moreover, we employ inter-frame correspondence and intra-frame correspondence to construct different views and transformations for better learning the representation from palindromic sequences. We validate our method on the seven challenging benchmarks. The results demonstrate considerable improvements over recent self-supervised algorithms and even some fully-supervised deep trackers. & COPY; 2023 Elsevier B.V. All rights reserved.
更多查看译文
关键词
Self-supervised learning,Visual tracking,Cycle consistency,Transformer
AI 理解论文
溯源树
样例
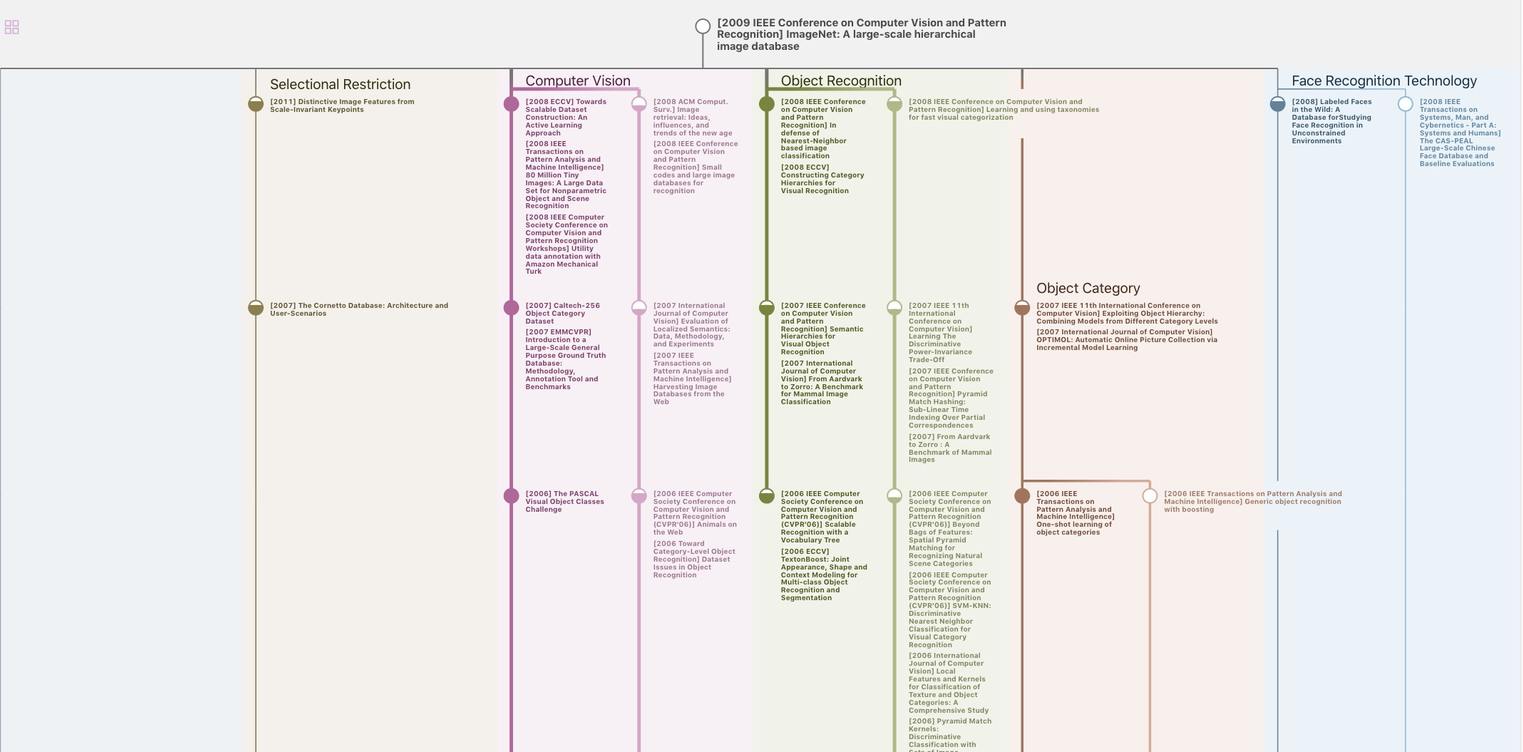
生成溯源树,研究论文发展脉络
Chat Paper
正在生成论文摘要