Influence of Feature Scaling and Number of Training Sessions on EEG Spectral-based Person Verification with Artificial Neural Networks
2023 Signal Processing Symposium (SPSympo)(2023)
摘要
Research on using EEG as a biometric began over 20 years ago. However, compared to more traditional modalities like fingerprints, EEG is still relatively novel. Further studies on more extensive databases are necessary to properly verify proposed methods, accounting for temporary fluctuations in the EEG signal. The study focuses on the impact of methods of feature scaling on the efficiency of EEG spectral-based person verification using artificial neural networks. For our research, we used signals collected from 29 individuals, with 20 recording sessions, each performed on different days. Having such a database, the influence of a number of training sessions on the verification results was also investigated. We tested various methods for scaling estimates of power spectral density coefficients, including power normalization, 5–95 percentile normalization, interquartile range normalization, and raw PSD estimate coefficients converted to the decibel scale. The best results were obtained after converting them to the decibel scale, which resulted in the accuracy of EEG-based people verification equals to 96.6 %.
更多查看译文
关键词
EEG,verification,biometry,PSD,neural networks
AI 理解论文
溯源树
样例
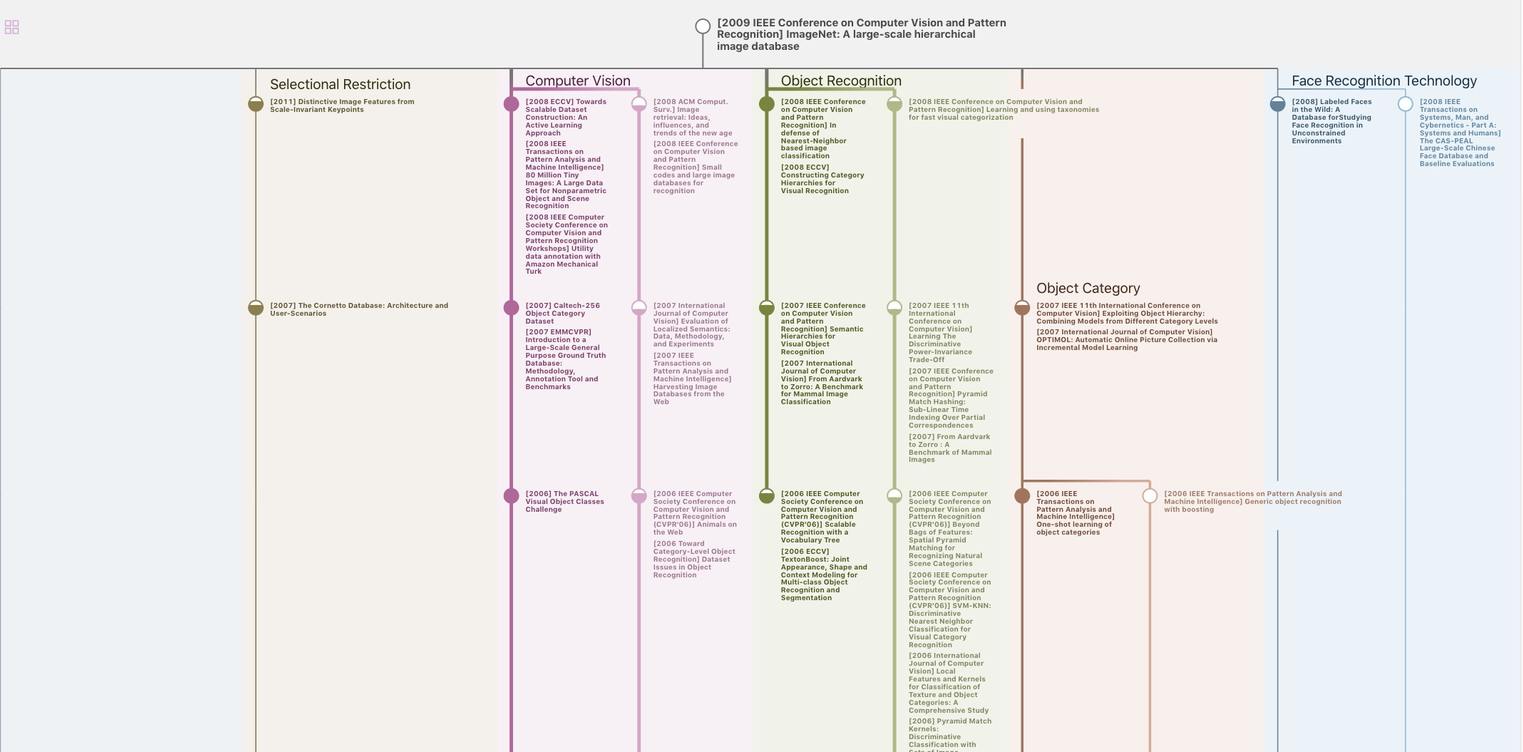
生成溯源树,研究论文发展脉络
Chat Paper
正在生成论文摘要