DAIL: Data Augmentation for In-Context Learning via Self-Paraphrase.
CoRR(2023)
摘要
In-Context Learning (ICL) combined with pre-trained large language models has achieved promising results on various NLP tasks. However, ICL requires high-quality annotated demonstrations which might not be available in real-world scenarios. To overcome this limitation, we propose \textbf{D}ata \textbf{A}ugmentation for \textbf{I}n-Context \textbf{L}earning (\textbf{DAIL}). DAIL leverages the intuition that large language models are more familiar with the content generated by themselves. It first utilizes the language model to generate paraphrases of the test sample and employs majority voting to determine the final result based on individual predictions. Our extensive empirical evaluation shows that DAIL outperforms the standard ICL method and other ensemble-based methods in the low-resource scenario. Additionally, we explore the use of voting consistency as a confidence score of the model when the logits of predictions are inaccessible. We believe our work will stimulate further research on ICL in low-resource settings.
更多查看译文
关键词
data augmentation,learning,in-context,self-paraphrase
AI 理解论文
溯源树
样例
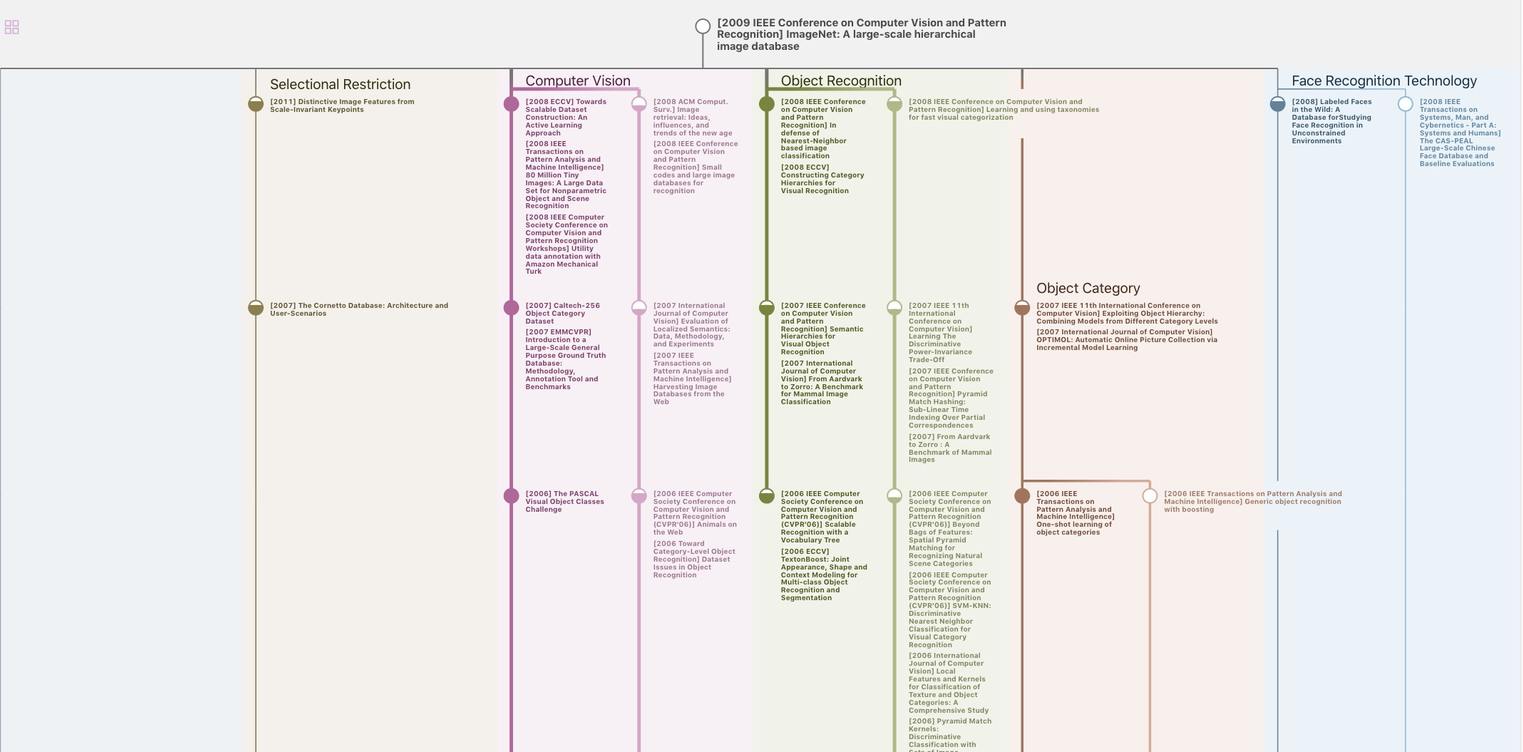
生成溯源树,研究论文发展脉络
Chat Paper
正在生成论文摘要