Narrowing the Gap between Zero- and Few-shot Machine Translation by Matching Styles.
CoRR(2023)
摘要
Large language models trained primarily in a monolingual setting have demonstrated their ability to generalize to machine translation using zero- and few-shot examples with in-context learning. However, even though zero-shot translations are relatively good, there remains a discernible gap comparing their performance with the few-shot setting. In this paper, we investigate the factors contributing to this gap and find that this gap can largely be closed (for about 70%) by matching the writing styles of the target corpus. Additionally, we explore potential approaches to enhance zero-shot baselines without the need for parallel demonstration examples, providing valuable insights into how these methods contribute to improving translation metrics.
更多查看译文
关键词
machine translation,few-shot
AI 理解论文
溯源树
样例
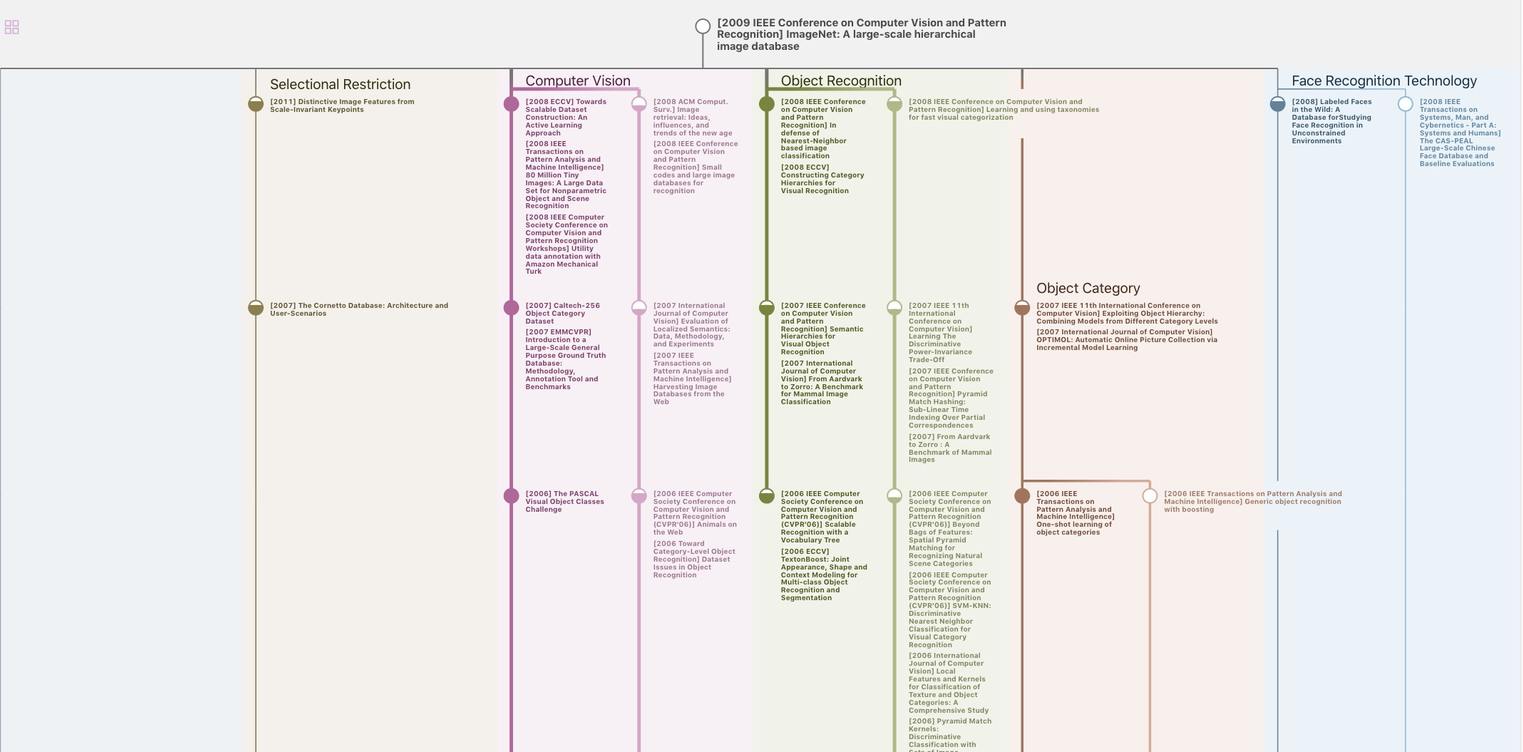
生成溯源树,研究论文发展脉络
Chat Paper
正在生成论文摘要