Neighborhood multigranulation rough sets for cost-sensitive feature selection on hybrid data
NEUROCOMPUTING(2024)
Abstract
Feature selection is a vital preprocessing step in real applications of data mining and machine learning. With the prevalence of high-dimensional hybrid data sets in real-world scenarios, along with the presence of test costs and misclassification costs, the need for effective feature selection methods has become more prominent. However, existing feature selection approaches mainly focus on cost-sensitive data from a single granularity perspective, and they are primarily applicable to single-typed data sets. To address these limitations, this paper presents a novel feature selection approach specifically designed for hybrid data, considering variable test costs and misclassification costs. The proposed method is based on neighborhood multigranulation rough sets (NMRS), which provides more comprehensive and multi-angle data analysis for cost-sensitive hybrid data. First, a novel multigranulation model is developed to effectively process cost-sensitive hybrid data. Building upon this model, a cost-based multi-criteria measure is proposed to evaluate the significance of features. This measure takes into account the comprehensive information of candidate features, including their power in algebraic view, information view, and associated costs. Furthermore, a heuristic feature selection algorithm based on NMRS is proposed to handle hybrid data with the test costs and misclassification costs. This algorithm leverages the benefits of the proposed multigranulation model and cost-based measure to identify the most discriminative features efficiently. Finally, the experimental results on twelve different datasets show that the proposed heuristic algorithm outperforms other compared algorithms, especially in total cost and classification accuracy.
MoreTranslated text
Key words
Feature selection,Rough sets,Hybrid data,Multi-granulation,Neighborhood,Cost-sensitive learning
AI Read Science
Must-Reading Tree
Example
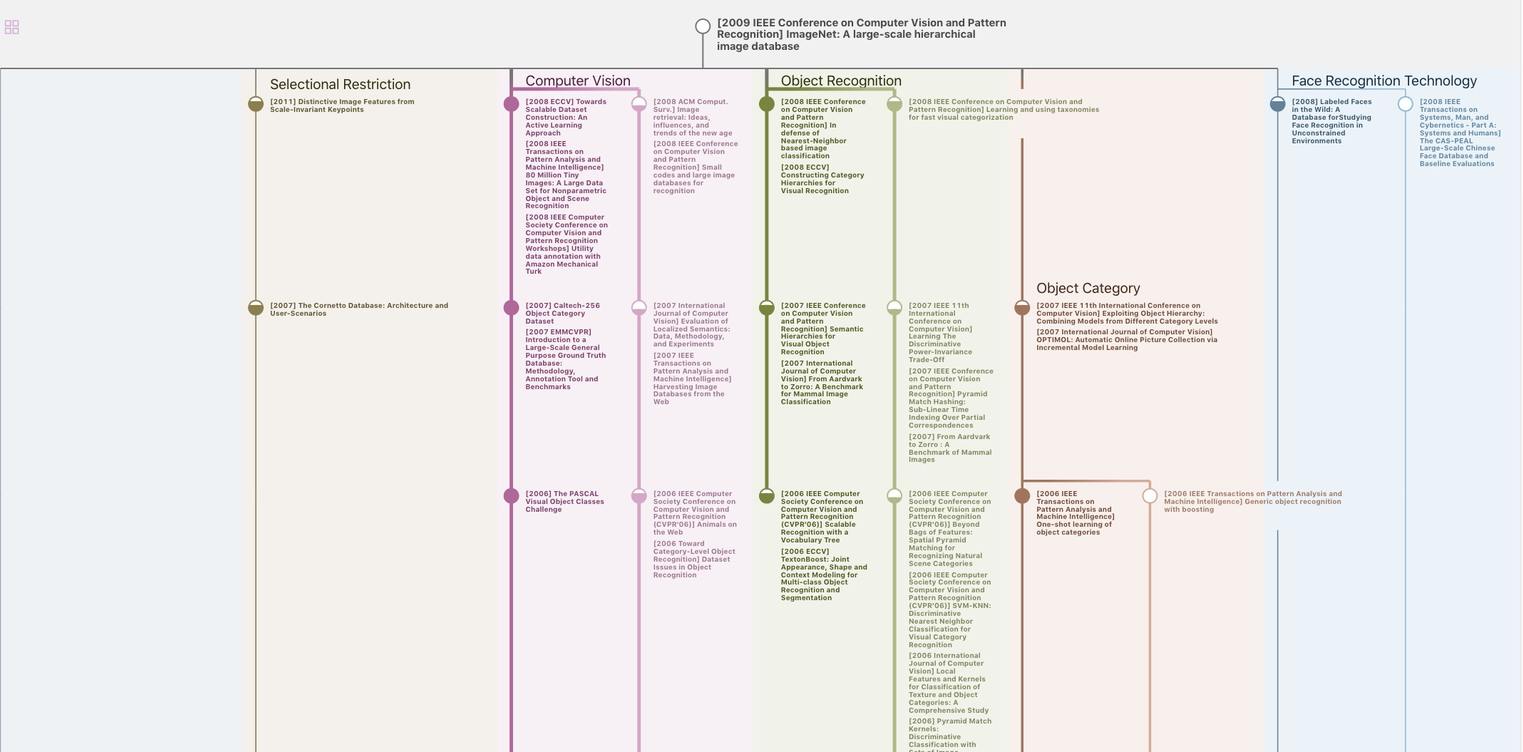
Generate MRT to find the research sequence of this paper
Chat Paper
Summary is being generated by the instructions you defined