Computational modelling for predicting rheological properties of composite modified asphalt binders
CASE STUDIES IN CONSTRUCTION MATERIALS(2023)
摘要
The complicated viscoelastic characteristics of asphalt binders make it a challenging task to precisely predict their rheological behavior. This study aims to investigate and compare the suitability of response surface methodology (RSM) and machine learning (ML) modeling approaches in predicting the complex modulus (G*), phase angle (8), and rutting parameter (G*/ sin8) of Nano Silica (NS) and/or waste denim fiber (WDF) modified asphalt binders before and after short-term aging. To achieve this, an experimental scheme was designed for RSM and ML modeling with three variables including NS contents (0-6%), WDF contents (0-6%), and testing temperature (40-76 degrees C) as the inputs, and provided the G*, 8 and G*/sin8 before and after shortterm aging as the outputs. A wide range of ML algorithms was evaluated to determine the optimum ML model that can be used to accurately predict the rheological properties of NS/WDFmodified asphalt binders. RSM analysis results indicated that the G*, 8, and G*/sin8 of NS/ WDF composite asphalt are significantly affected by the %NS, %WDF, and test temperatures. The RSM-developed models showed coefficient of determination (R2) values exceeding 0.97 for all responses, indicating adequate agreement between experimental results and models developed by RSM. From ML algorithms optimization and among all evaluated ML models, it was found that Gaussian process regression (GPR) exhibited the highest R2 with a value of (0.99) and the lowest Root Mean Square Error (RMSE) with a value of approximately 1%. The performance evaluation of the GPR model for predicting all responses showed a very small difference between the predicted and experimental results, highlighting the prediction accuracy of the developed ML models.
更多查看译文
关键词
Composite modified asphalt,Rheological properties,Complex modulus,Phase angle,Response surface methodology,Machine learning
AI 理解论文
溯源树
样例
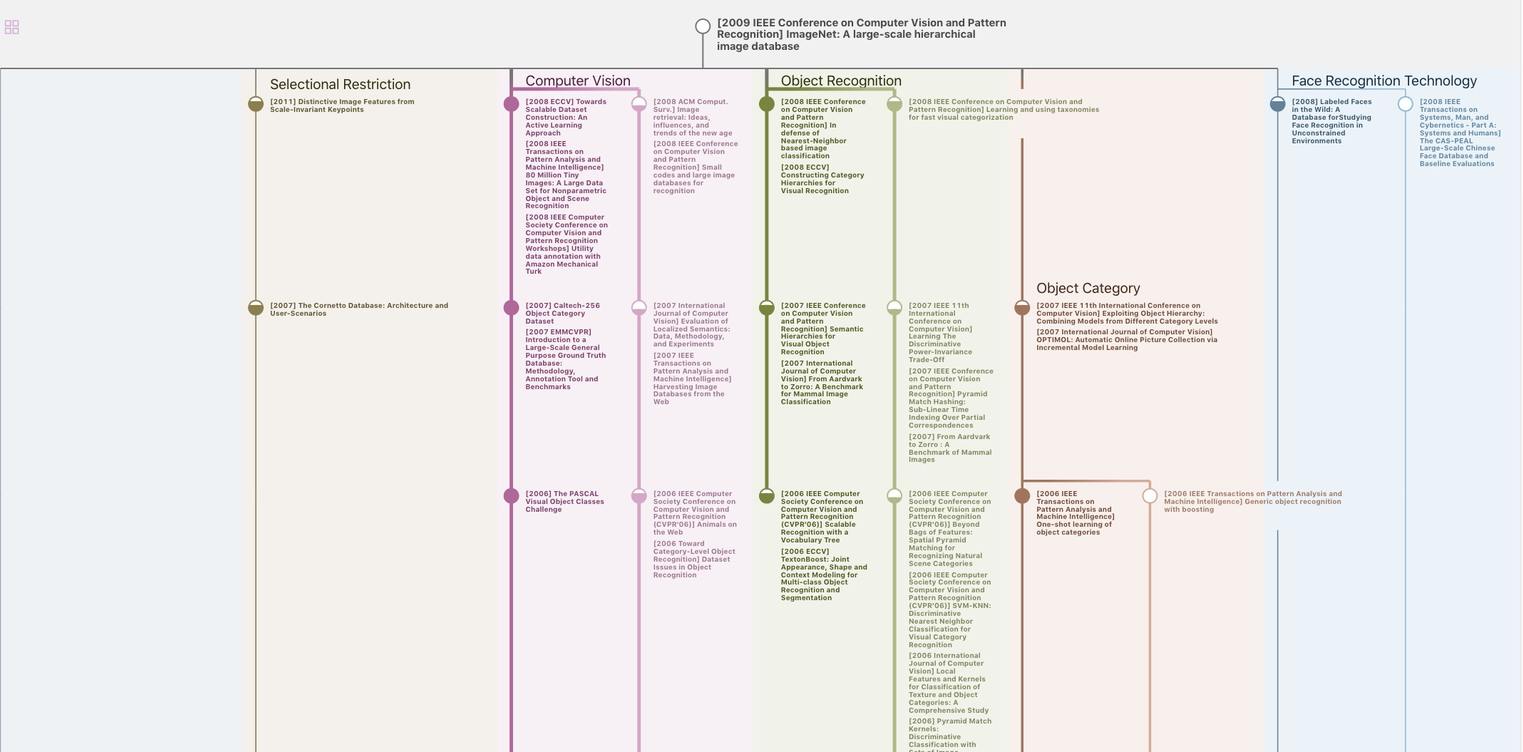
生成溯源树,研究论文发展脉络
Chat Paper
正在生成论文摘要