A deep learning method for the dynamics of classic and conservative Allen-Cahn equations based on fully-discrete operators
JOURNAL OF COMPUTATIONAL PHYSICS(2024)
摘要
The Allen-Cahn equation is a well-known stiff semilinear parabolic equation used to describe the process of phase separation and transition in phase field modeling of multi-component physical systems, while the conservative Allen-Cahn equation is a modified version of the classic Allen-Cahn equation that can additionally conserve the mass. As neural networks and deep learning techniques have achieved significant successes in recent years in scientific and engineering applications, there has been growing interest in developing deep learning algorithms for numerical solutions of partial differential equations. In this paper, we propose a novel deep learning method for predicting the dynamics of the classic and conservative Allen-Cahn equations. Specifically, we design two special convolutional neural network models, one for each of the two equations, to learn the fully-discrete operators between two adjacent time steps. The loss functions of the two models are defined using the residual of the fully-discrete systems, which result from applying the central finite difference discretization in space and the Crank- Nicolson approximation in time. This approach enables us to train the models without requiring any ground-truth data. Moreover, we introduce an effective training strategy that automatically generates useful samples along the time evolution to facilitate training of the models. Finally, we conduct extensive experiments in two and three dimensions to demonstrate outstanding performance of our proposed method, including its dynamics prediction and generalization ability under different scenarios.
更多查看译文
关键词
Allen-Cahn equations,Mass-conserving,Fully-discrete operator,Convolutional neural network,Bound limiter,Training strategy
AI 理解论文
溯源树
样例
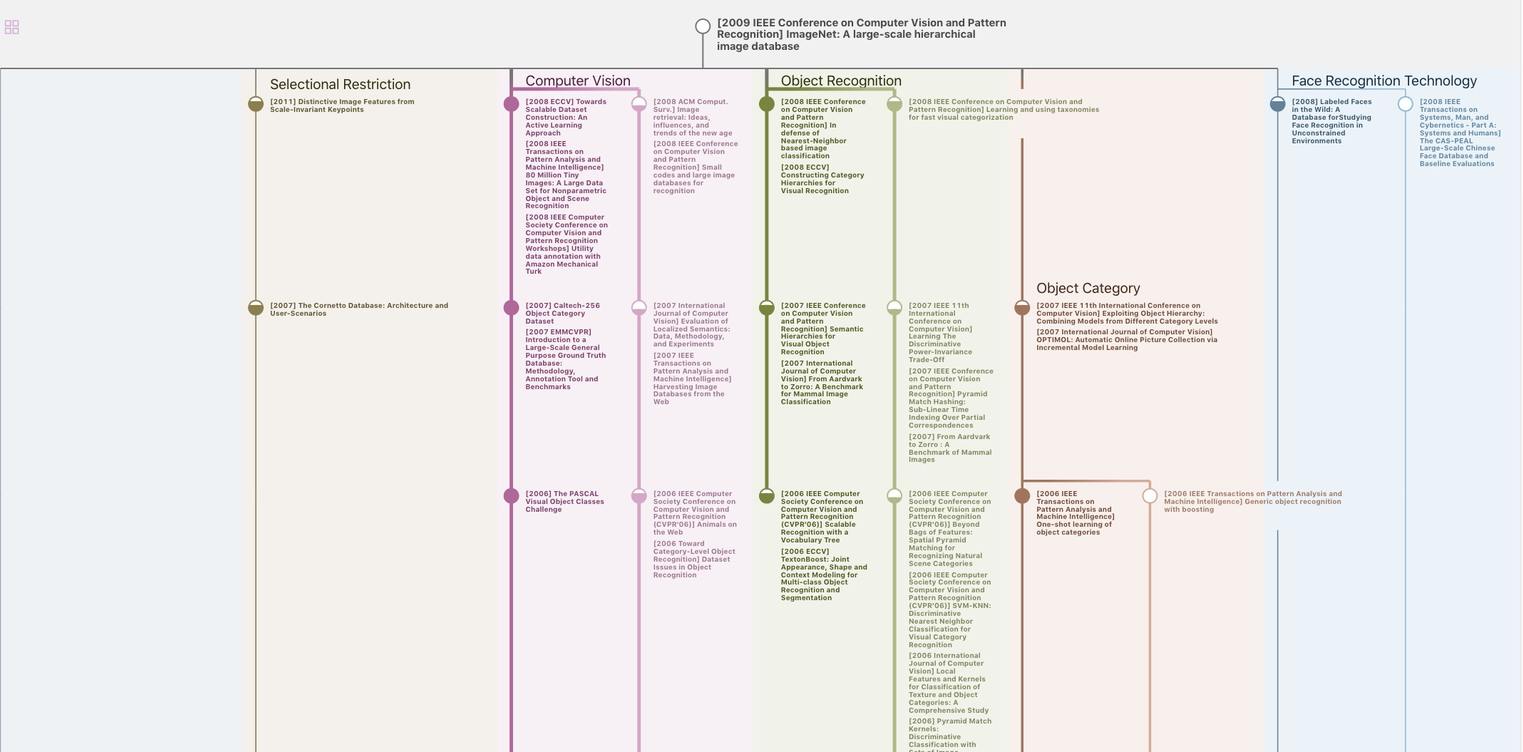
生成溯源树,研究论文发展脉络
Chat Paper
正在生成论文摘要