MiRGraph: A transformer-based feature learning approach to identify microRNA-target interactions by integrating heterogeneous graph network and sequence information
bioRxiv (Cold Spring Harbor Laboratory)(2024)
Abstract
MicroRNAs (miRNAs) play a crucial role in the regulation of gene expression by targeting specific mRNAs. They can function as both tumor suppressors and oncogenes depending on the specific miRNA and its target genes. Detecting miRNA-target interactions (MTIs) is critical for unraveling the complex mechanisms of gene regulation and identifying therapeutic targets and diagnostic markers. There is currently a lack of MTIs prediction method that simultaneously performs feature learning on heterogeneous graph network and sequence information. To improve the prediction performance of MTIs, we present a novel transformer-based multi-view feature learning method, named MiRGraph. It consists of two main modules for learning the sequence and heterogeneous graph network, respectively. For learning the sequence-based feaature embedding, we utilize the mature miRNA sequence and the complete 3’UTR sequence of the target mRNAs to encode sequence features. Specifically, a transformer-based CNN (TransCNN) module is designed for miRNAs and genes respectively to extract their personalized sequence features. For learning the network-based feature embedding, we utilize a heterogeneous graph transformer (HGT) module to extract the relational and structural information in a heterogeneous graph consisting of miRNA-miRNA, gene-gene and miRNA-target interactions. We learn the TransCNN and HGT modules end-to-end by utilizing a feedforward network, which takes the combined embedded features of the miRNA-gene pair to predict MTIs. Comparisons with other existing MTIs prediction methods illustrates the superiority of MiRGraph under standard criteria. In a case study on breast cancer, we identified plausible target genes of an oncomir hsa-MiR-122-5p and plausible miRNAs that regulate the oncogene BRCA1 .
### Competing Interest Statement
The authors have declared no competing interest.
MoreTranslated text
Key words
interactions,learning,feature,transformer-based,mirna-target
AI Read Science
Must-Reading Tree
Example
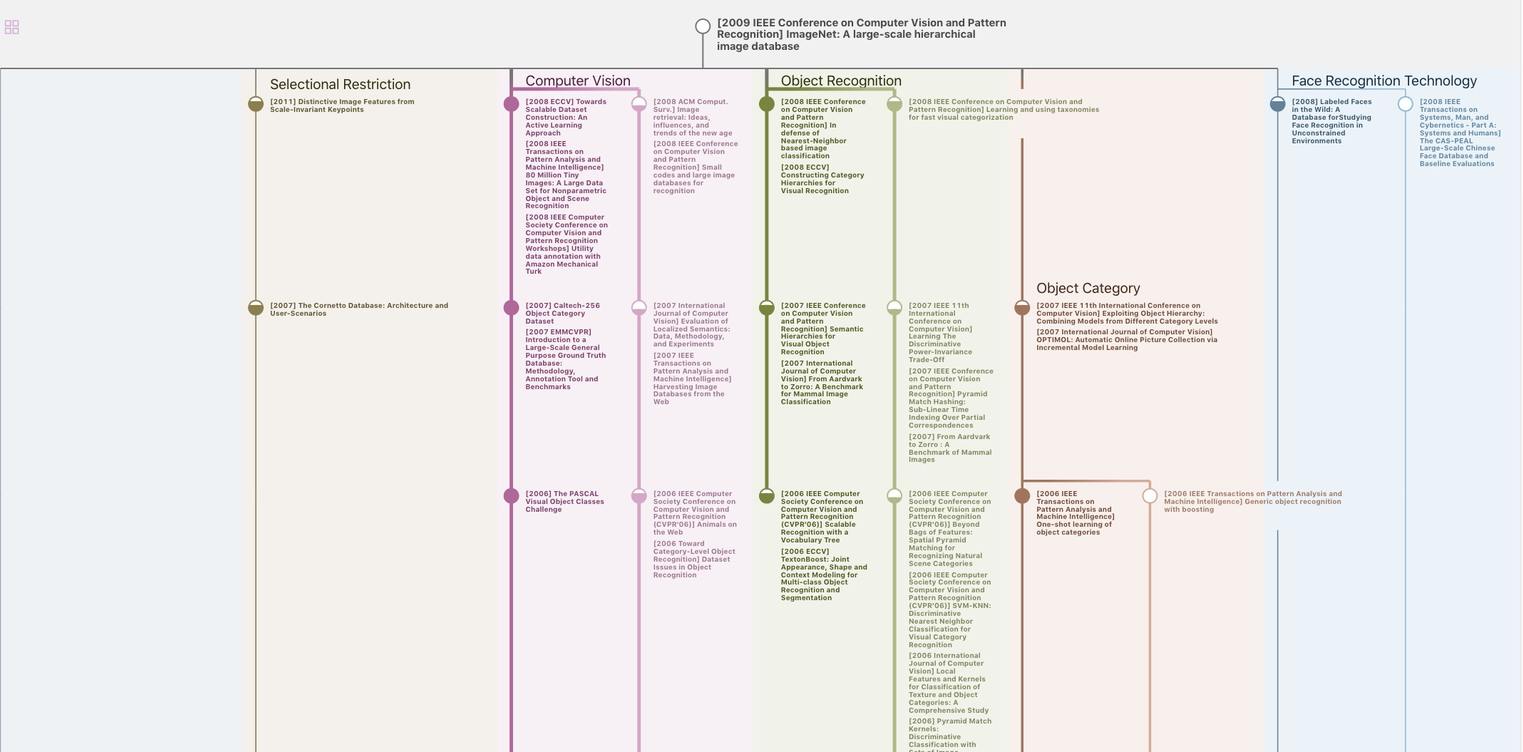
Generate MRT to find the research sequence of this paper
Chat Paper
Summary is being generated by the instructions you defined