GFNet: A pioneering approach for precisely estimating ash content in coal through the fusion of graph convolution and feedforward network
ENGINEERING APPLICATIONS OF ARTIFICIAL INTELLIGENCE(2024)
Abstract
Accurate determination of ash content in coal is paramount for quality control in the coal industry. However, conventional methods are time-consuming and ill-suited for real-time process control. To address this challenge, our study proposed a novel approach, GFNet, which integrated graph convolution and a feedforward network for ash content estimation. The image was divided into patches and transformed into a graph structure using a knearest Neighbor (k-NN) function. We introduced relative position encoding to each node feature to enhance feature representation. The GFNet architecture consisted of a stack of GF (graph convolution and FFN), facilitating feature extraction from the graph representation of image patches. Graph convolution captured intricate spatial relationships and interdependencies among patches, enabling effective information aggregation and updating within the graph. The FFN module, featuring two linear layers, facilitated node feature transformation. We developed pyramid (PGFNet) and isotropic (IGFNet) architectures by stacking GF blocks. Extensive experiments substantiated the superior performance of the proposed approach compared to traditional methods, emphasizing accuracy and efficiency. The GFNet achieved remarkable results, with PGFNet-S attaining a 96.36 accuracy and a mean absolute error (MAE) of 0.145%. IGFNet-Ti demonstrated a higher accuracy of 98.30% with an MAE of 0.093%. Visualization of the graph structure elucidated the model's proficiency in processing graph structure and node features, illustrating its capability to capture content-relevant nodes and gradually connect nodes with similar semantics. This pioneering work presented a transformative approach to ash content estimation in coal quality analysis, offering an invaluable tool for the coal industry's quality control needs.
MoreTranslated text
Key words
Coal quality analysis,Dynamic graph convolution,Deep learning,Machine vision,Coal quality control
AI Read Science
Must-Reading Tree
Example
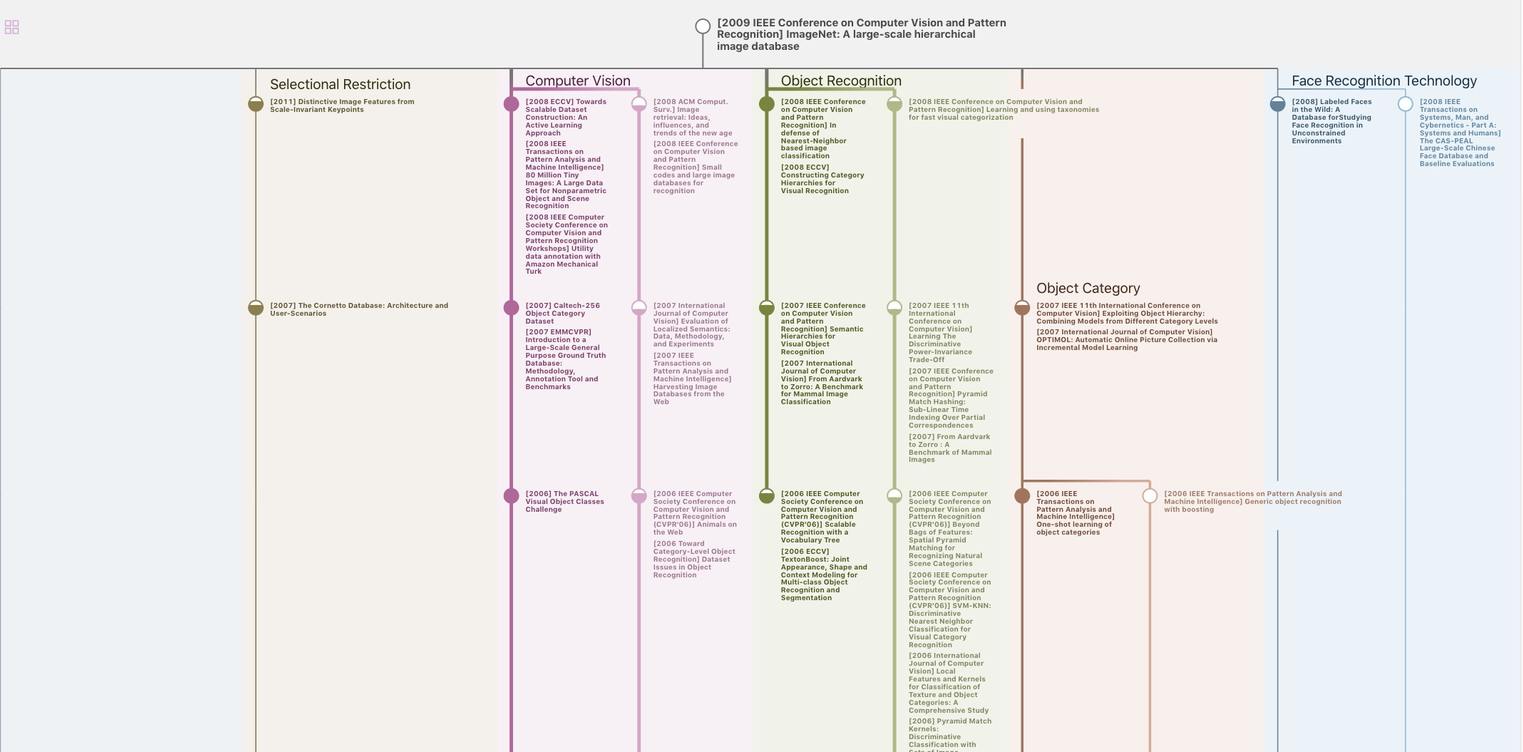
Generate MRT to find the research sequence of this paper
Chat Paper
Summary is being generated by the instructions you defined