Bi-Level-Based Inverse Stochastic Optimal Control
arXiv (Cornell University)(2023)
摘要
In this paper, we propose a new algorithm to solve the Inverse Stochastic Optimal Control (ISOC) problem of the linear-quadratic sensorimotor (LQS) control model. The LQS model represents the current state-of-the-art in describing goal-directed human movements. The ISOC problem aims at determining the cost function and noise scaling matrices of the LQS model from measurement data since both parameter types influence the statistical moments predicted by the model and are unknown in practice. We prove global convergence for our new algorithm and at a numerical example, validate the theoretical assumptions of our method. By comprehensive simulations, the influence of the tuning parameters of our algorithm on convergence behavior and computation time is analyzed. The new algorithm computes ISOC solutions nearly 33 times faster than the single previously existing ISOC algorithm.
更多查看译文
关键词
inverse stochastic optimal control,bi-level-based
AI 理解论文
溯源树
样例
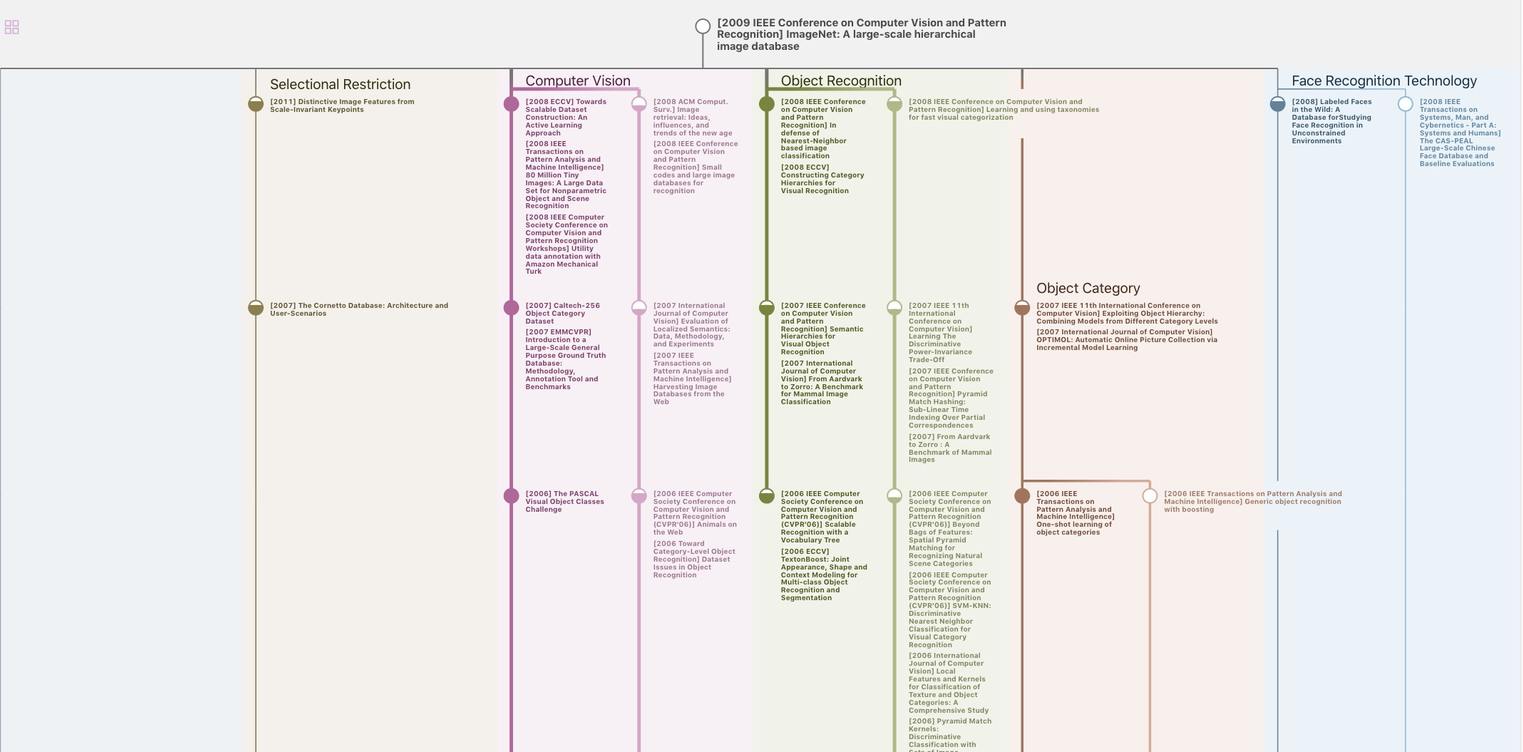
生成溯源树,研究论文发展脉络
Chat Paper
正在生成论文摘要