AntiViralDL: Computational Antiviral Drug Repurposing Using Graph Neural Network and Self-Supervised Learning
IEEE JOURNAL OF BIOMEDICAL AND HEALTH INFORMATICS(2024)
摘要
Viral infections have emerged as significant public health concerns for decades. Antiviral drugs, specifically designed to combat these infections, have the potential to reduce the disease burden substantially. However, traditional drug development methods, based on biological experiments, are resource-intensive, time-consuming, and low efficiency. Therefore, computational approaches for identifying antiviral drugs can enhance drug development efficiency. In this study, we introduce AntiViralDL, a computational framework for predicting virus-drug associations using self-supervised learning. Initially, we construct a reliable virus-drug association dataset by integrating the existing Drugvirus2 database and FDA-approved virus-drug associations. Utilizing these two datasets, we create a virus-drug association bipartite graph and employ the Light Graph Convolutional Network (LightGCN) to learn embedding representations of viruses and drugs. To address the sparsity of virus-drug association pairs, AntiViralDL incorporates contrastive learning to improve prediction accuracy. We implement data augmentation by adding random noise to the embedding representation space of virus and drug nodes, as opposed to traditional edge and node dropout. Finally, we calculate an inner product to predict virus-drug association relationships. Experimental results reveal that AntiViralDL achieves AUC and AUPR values of 0.8450 and 0.8494, respectively, outperforming four benchmarked virus-drug association prediction models. The case study further highlights the efficacy of AntiViralDL in predicting anti-COVID-19 drug candidates.
更多查看译文
关键词
Bipartite graph,contrastive learning,graph convolutional network,self-supervised learning,virus-drug associations
AI 理解论文
溯源树
样例
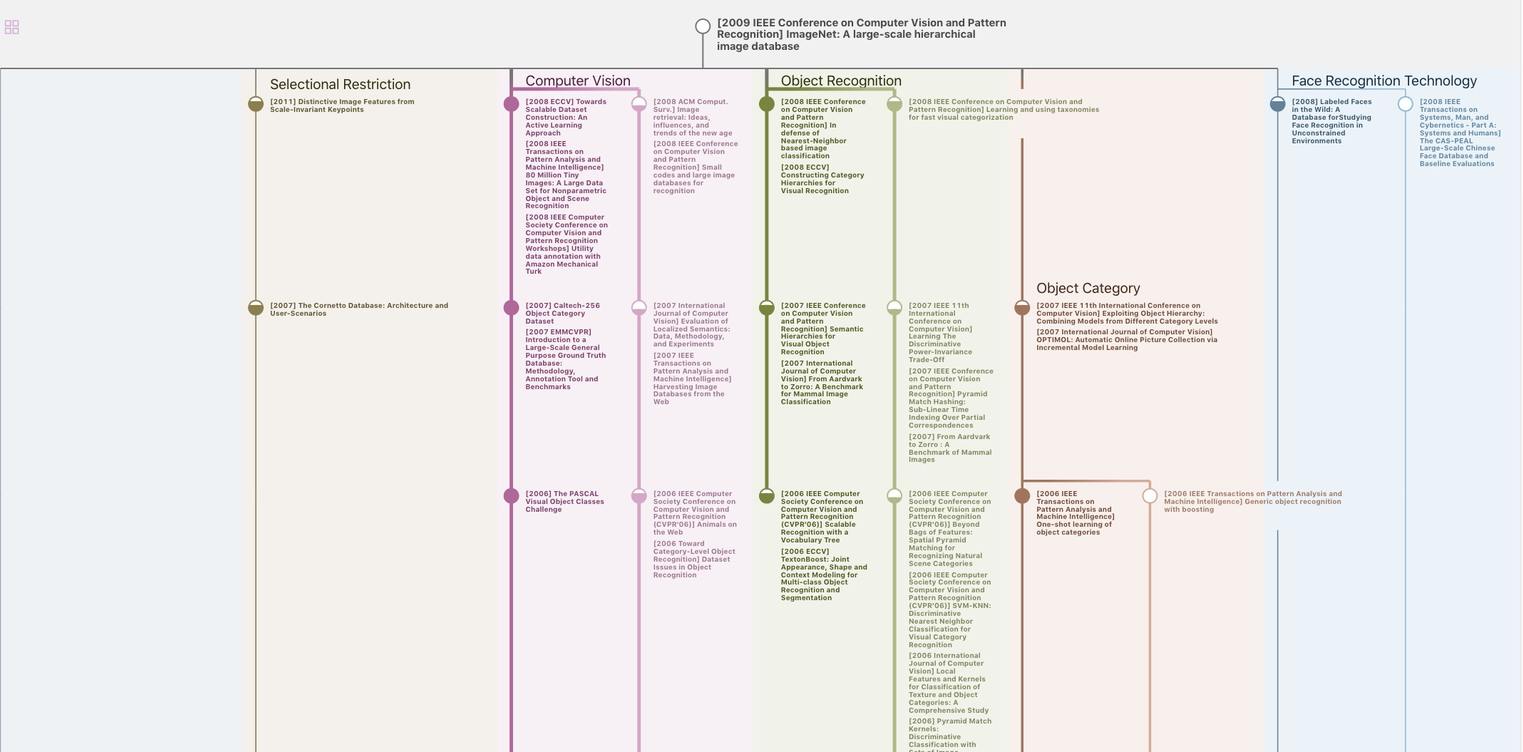
生成溯源树,研究论文发展脉络
Chat Paper
正在生成论文摘要