Deep neural network based tissue deconvolution of circulating tumor cell RNA
Journal of Translational Medicine(2023)
Abstract
Prior research has shown that the deconvolution of cell-free RNA can uncover the tissue origin. The conventional deconvolution approaches rely on constructing a reference tissue-specific gene panel, which cannot capture the inherent variation present in actual data. To address this, we have developed a novel method that utilizes a neural network framework to leverage the entire training dataset. Our approach involved training a model that incorporated 15 distinct tissue types. Through one semi-independent and two complete independent validations, including deconvolution using a semi in silico dataset, deconvolution with a custom normal tissue mixture RNA-seq data, and deconvolution of longitudinal circulating tumor cell RNA-seq (ctcRNA) data from a cancer patient with metastatic tumors, we demonstrate the efficacy and advantages of the deep-learning approach which were exerted by effectively capturing the inherent variability present in the dataset, thus leading to enhanced accuracy. Sensitivity analyses reveal that neural network models are less susceptible to the presence of missing data, making them more suitable for real-world applications. Moreover, by leveraging the concept of organotropism, we applied our approach to trace the migration of circulating tumor cell-derived RNA (ctcRNA) in a cancer patient with metastatic tumors, thereby highlighting the potential clinical significance of early detection of cancer metastasis.
MoreTranslated text
Key words
tissue deconvolution,tumor cell rna,cell rna,deep neural network
AI Read Science
Must-Reading Tree
Example
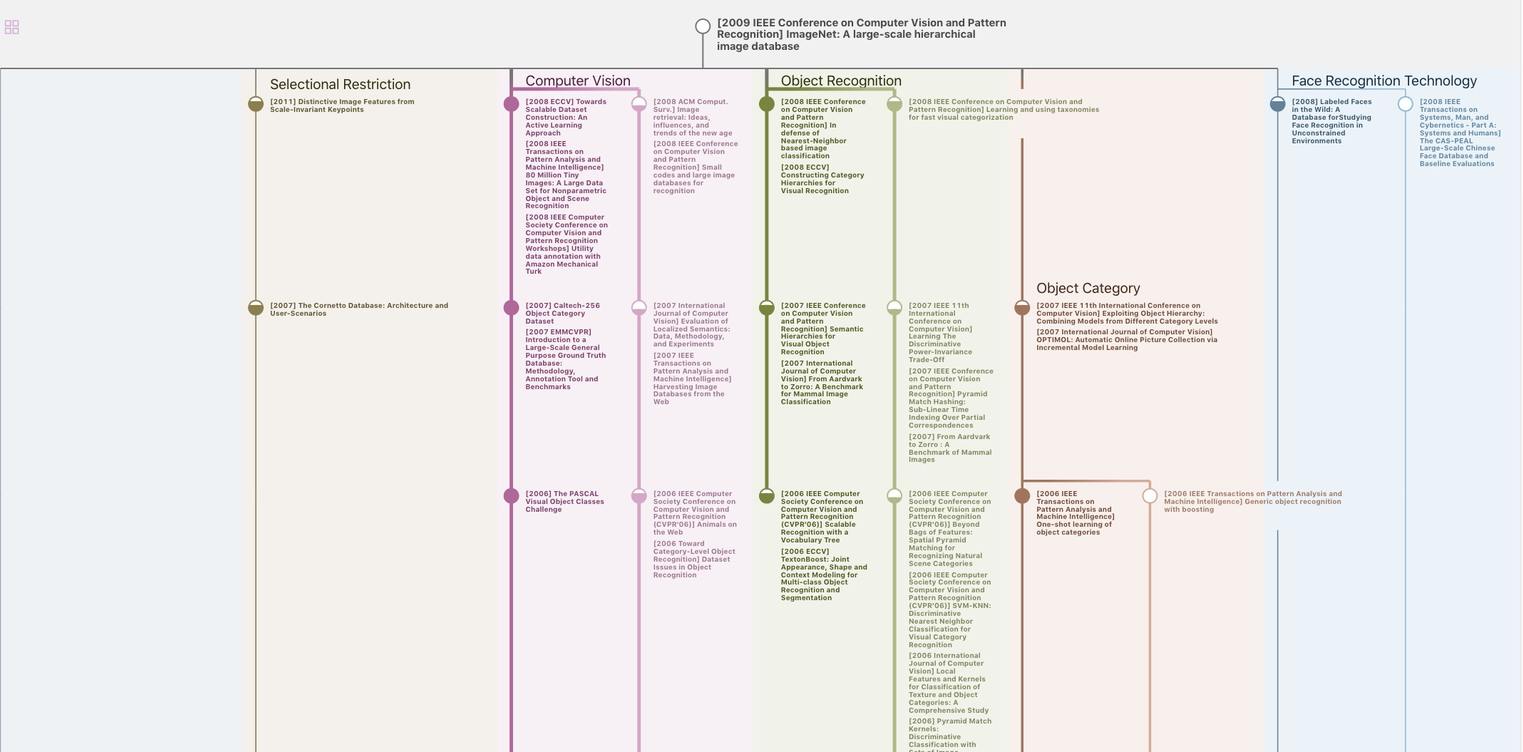
Generate MRT to find the research sequence of this paper
Chat Paper
Summary is being generated by the instructions you defined