A lightweight approach based on prompt for few-shot relation extraction
COMPUTER SPEECH AND LANGUAGE(2024)
摘要
Few-shot relation extraction (FSRE) aims to predict the relation between two entities in a sen-tence using a few annotated samples. Many works solve the FSRE problem by training complex models with a huge number of parameters, which results in longer processing times to obtain results. Some recent works focus on introducing relation information into Prototype Networks in various ways. However, most of these methods obtain entity and relation representations by fine-tuning large pre-trained language models. This implies that a copy of the complete pre-trained model needs to be saved after fine-tuning for each specific task, leading to a shortage of computing and space resources. To address this problem, in this paper, we introduce a light approach that utilizes prompt-learning to assist in fine-tuning model by adjusting fewer parameters. To obtain a better prototype of relation, we design a new enhanced fusion module to fuse relation information and original prototype. We conduct extensive experiments on the common FSRE datasets FewRel 1.0 and FewRel 2.0 to varify the advantages of our method, the results show that our model achieves state-of-the-art performance.
更多查看译文
关键词
Relation classification,Few-shot relation extraction,Prompt-tuning,Language model,Prototypical network
AI 理解论文
溯源树
样例
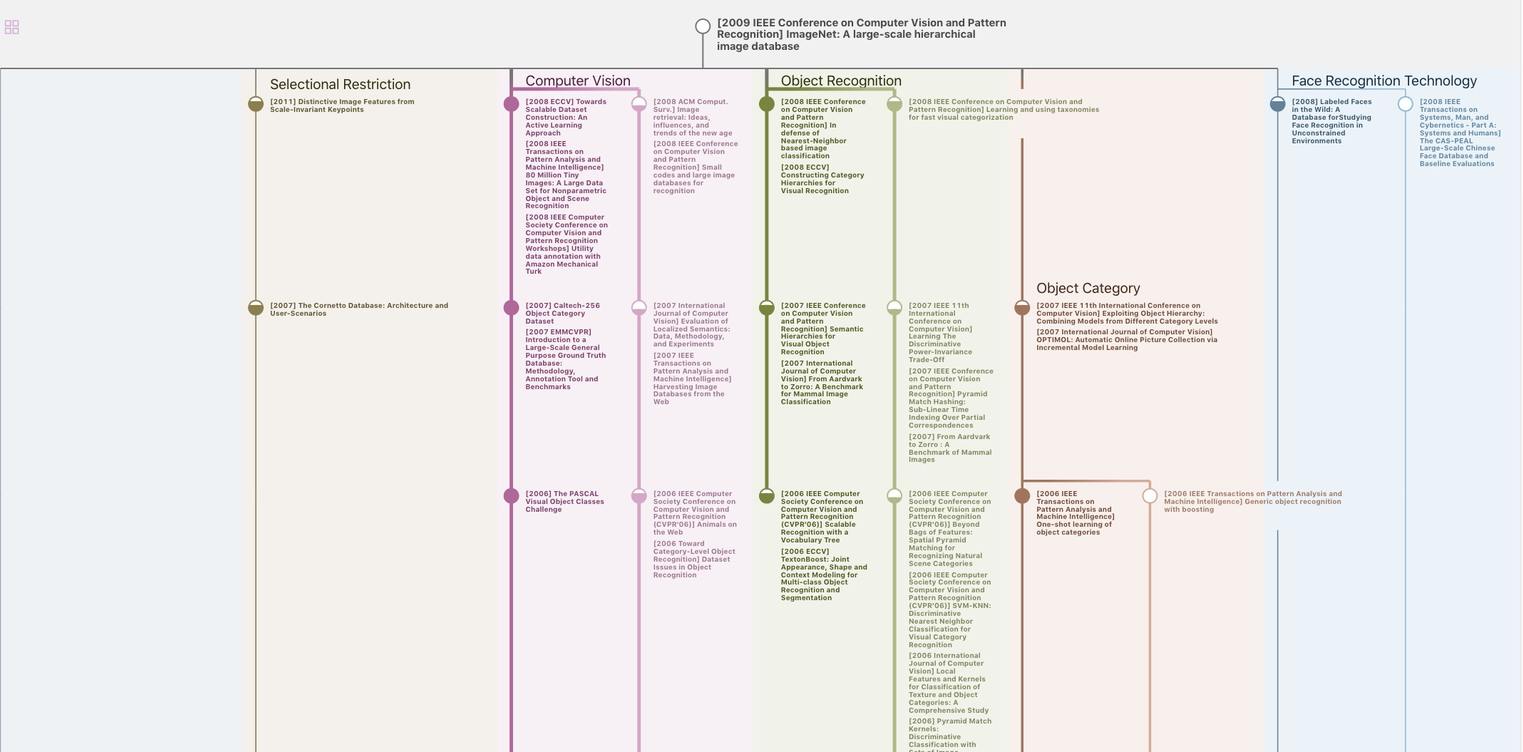
生成溯源树,研究论文发展脉络
Chat Paper
正在生成论文摘要