A new hybrid deep learning model for monthly oil prices forecasting
ENERGY ECONOMICS(2023)
摘要
The forecast of crude oil prices has always been important for investors and scholars and has drawn more attention to applying deep learning techniques in recent years. Under this circumstance, firstly, this paper proposes a novel hybrid deep learning forecasting model named Mod-VMD-BiLSTM based on the variational mode decomposition (VMD) and bidirectional long short-term memory (BiLSTM) algorithms. Next, several empirical studies and statistical evaluations are conducted to evaluate its forecasting performance. Our empirical results show that the preprocessing of decomposed series is beneficial to capture temporal general feature patterns hidden in sub-series, thereby helping to produce more accurate and robust forecasting results than the competing benchmark models among all scenarios. And all the evaluation metric values can pass the corresponding statistical tests, making the conclusions more convincing and comprehensive. Finally, the robustness tests confirm that the proposed forecasting framework is robust and superior for modeling and forecasting monthly oil prices time series.
更多查看译文
关键词
Long short-term memory,Empirical mode decomposition,Deep learning,Energy finance,Oil price forecasting
AI 理解论文
溯源树
样例
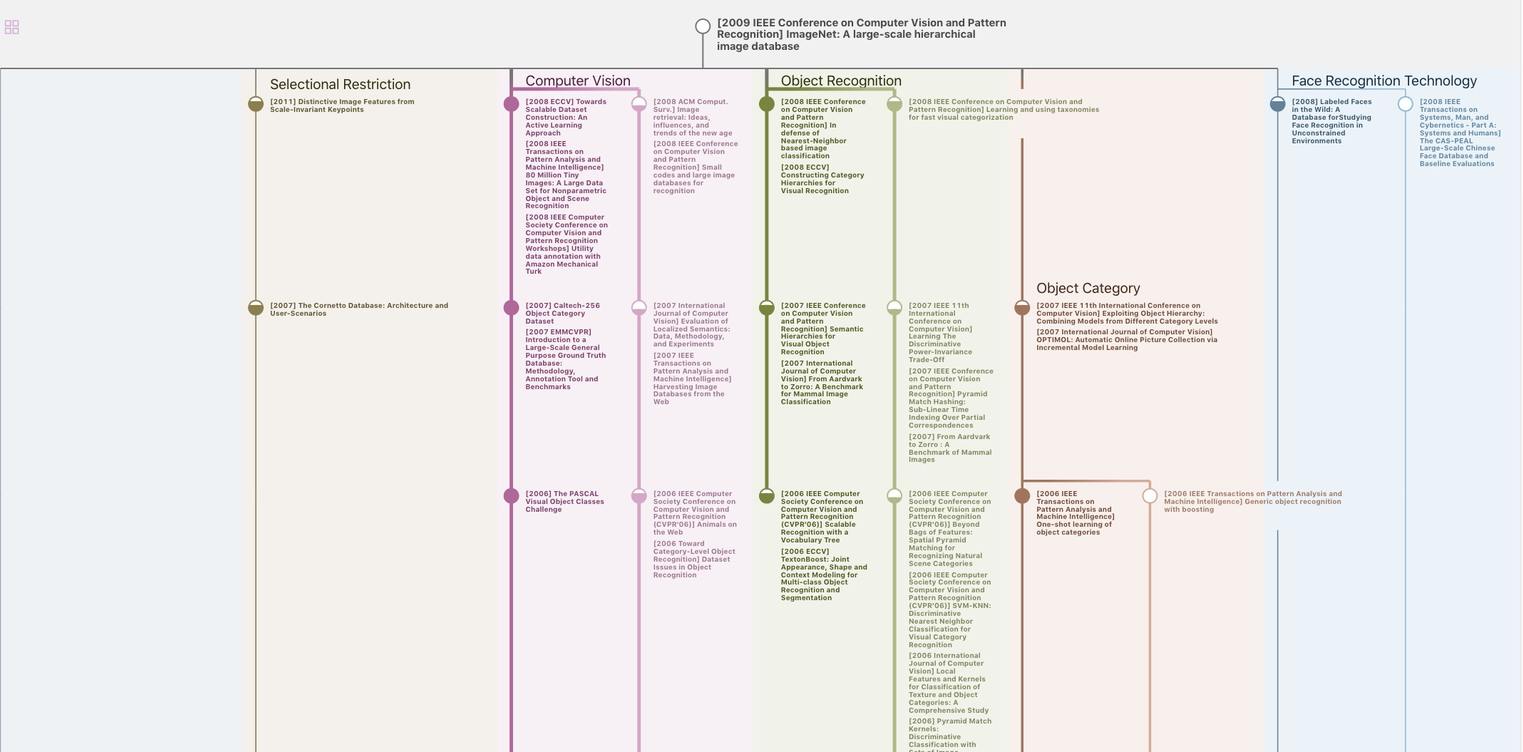
生成溯源树,研究论文发展脉络
Chat Paper
正在生成论文摘要