RUL prediction of rolling element bearings based on ACO-FELM
2023 CAA Symposium on Fault Detection, Supervision and Safety for Technical Processes (SAFEPROCESS)(2023)
摘要
Rolling element bearings are critical in rotating equipment. The condition of the bearings determines whether the entire mechanical equipment can function properly. If damaged bearings are not replaced promptly, this can lead to serious accidents or even to the scrapping of the entire equipment. Hence, accurately predicting the remaining operational lifespan of rolling bearings holds significant importance. The proposed method, which relies on the ant colony optimization algorithm, represents a feedback-driven optimization strategy for extreme learning machines. This approach allows for reliable estimation of the remaining functional duration of rolling bearings, even in scenarios with restricted vibration data. The method employed the relative root mean square evaluation that was not affected by individual variations of bearings, to assess their performance and degradation patterns. Furthermore, the variance of Relative Root Mean Square is utilized to ascertain whether the bearing is in the degradation stage. Once this stage is identified, it becomes feasible to predict the remaining useful life of the bearings. The validity of the proposed method was assessed in this study with a limited amount of experimental data on the acceleration life of bearings. The results demonstrate that the method enables proactive prediction of the remaining useful life and exhibits higher accuracy compared to other methods. Furthermore, the proposed method demonstrates good generalization capability across bearings under different operating conditions.
更多查看译文
关键词
Rolling element bearings,Feedback extreme learning machine,Remaining useful life prediction,Ant colony algorithm
AI 理解论文
溯源树
样例
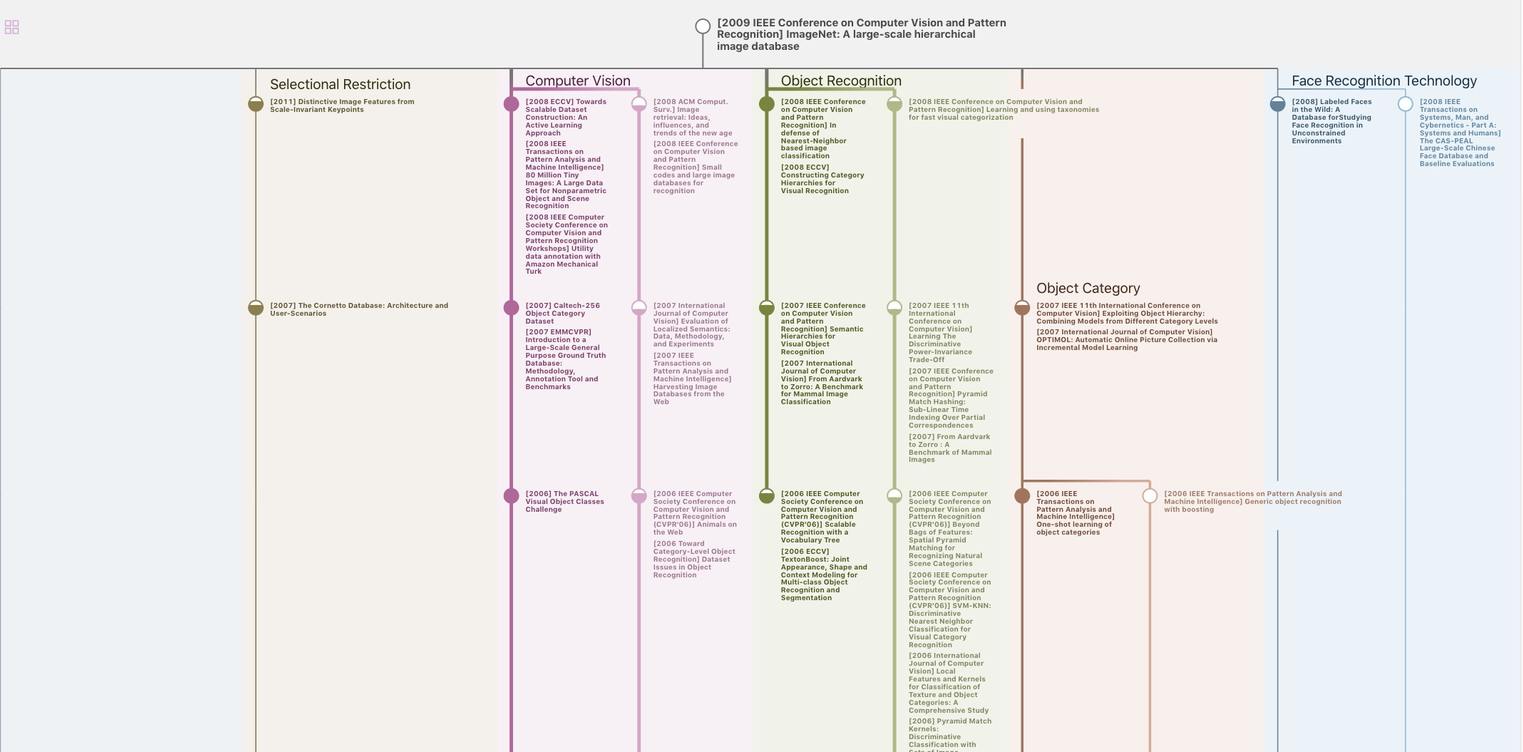
生成溯源树,研究论文发展脉络
Chat Paper
正在生成论文摘要