Self-Supervised Multi-LiDAR Object View Generation Using Single LiDAR
2023 IEEE 29th International Conference on Embedded and Real-Time Computing Systems and Applications (RTCSA)(2023)
摘要
3D object detection using point clouds has been widely used for self-driving vehicles, roadside vehicle detection, and tracking. However, the detection accuracy using single-LiDAR-scanned point clouds suffers from occlusion to have accurate bounding boxes. Several previous works proposed compensating for the point cloud to improve 3D detection. This work designed a self-labeling method that exploits the characteristics of multiple LiDAR-scanned point clouds collected on roadside units. This labeling method allows the point completion model to be trained without human-labeled bounding boxes. The point completion model uses the single-LiDAR-scanned point cloud as input and uses the extracted object points from the fused point cloud as the training target. The voxel IoU and recall of the point-completed point clouds increased by 17% and 22% compared to that of the raw point clouds. Additionally, the detection performance of DBSCAN, i.e., 3D AP@IoU=0.25 increased by 20% after applying the point completion algorithm.
更多查看译文
关键词
Self Supoervised,Point Clouds,Autonomous Driving
AI 理解论文
溯源树
样例
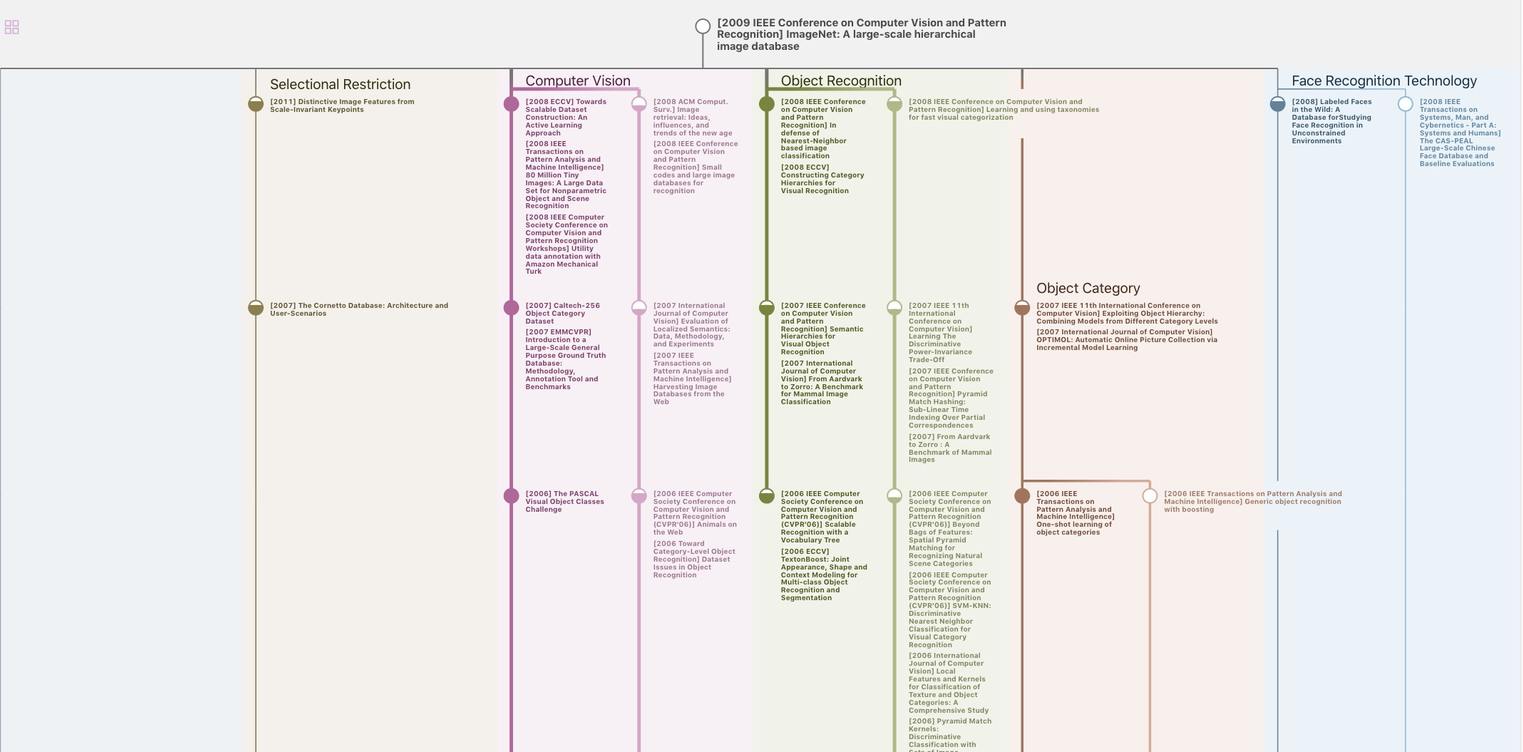
生成溯源树,研究论文发展脉络
Chat Paper
正在生成论文摘要