Missing multi-label learning based on the fusion of two-level nonlinear mappings
INFORMATION FUSION(2024)
摘要
The relationship between features and labels is an important factor affecting multi-label learning. Many existing multi-label learning approaches decompose label space into a low-rank latent label space and construct linear mappings between the feature space and the latent label space. This type of models may not depict the true data distribution well in high-dimensional spaces. In this work, a model called missing multi-label learning based on the fusion of two-level nonlinear mappings (MML-TLNM) is proposed. Specifically, in the second level mapping, the label matrix is nonlinearly decomposed into a low-rank latent label matrix and a correlation coefficient matrix to realize the nonlinear transformation from the latent label space to the actual label space. In the first-level mapping, a nonlinear relationship is constructed from the sample matrix to the latent label matrix to realize the nonlinear transformation between the original feature space and the latent label space. The two levels of nonlinear mappings are fused with each other in a nested manner and better depict the true distribution of multi-label data. In addition, the F-norm regularization constraints are imposed on the variables in the two-level nonlinear mappings. Extensive comparative experiments showed that the proposed learning model has good robustness and is superior to the state-of-the-art multi-label learning methods.
更多查看译文
关键词
Multi-label learning,Nonlinear mapping,Latent label,Missing label
AI 理解论文
溯源树
样例
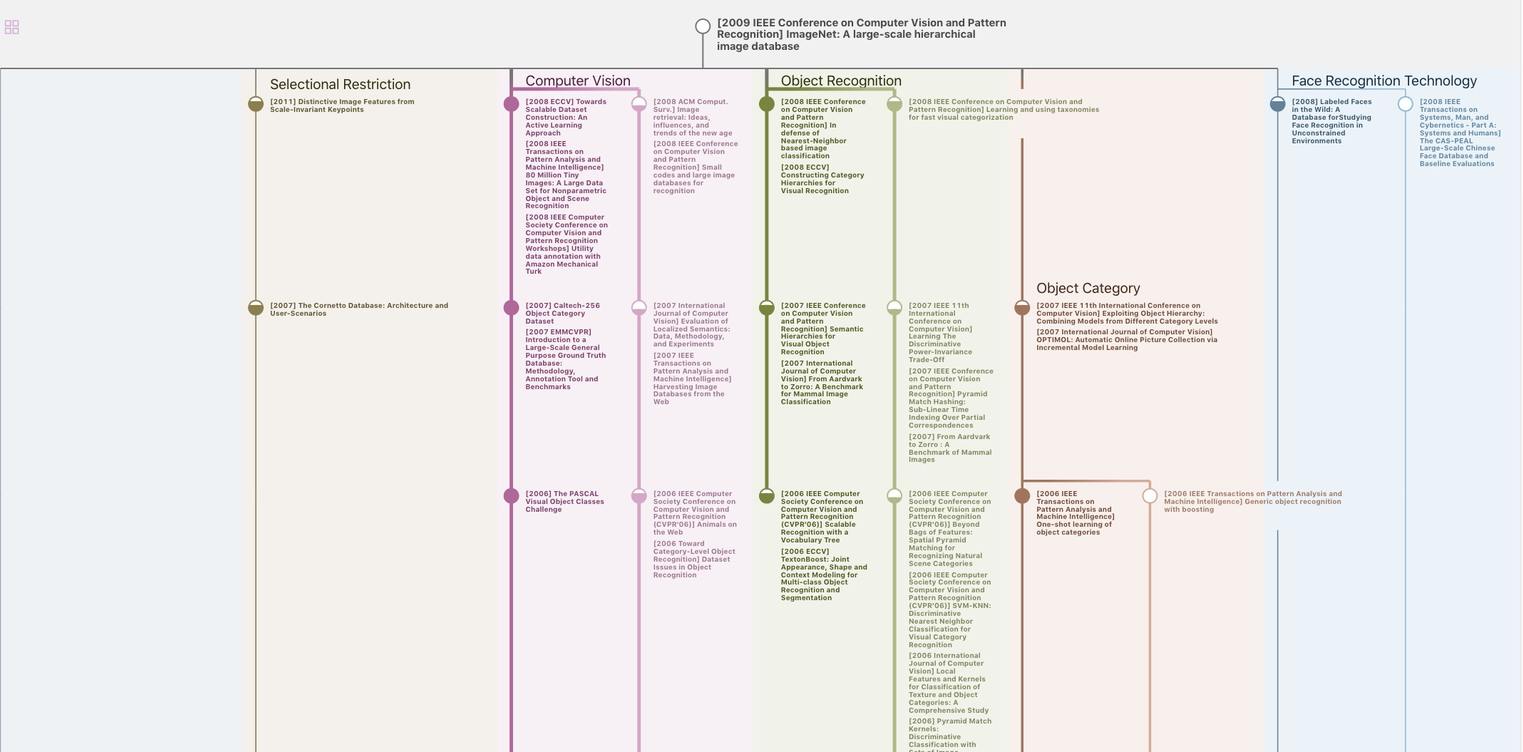
生成溯源树,研究论文发展脉络
Chat Paper
正在生成论文摘要