Using a Bayesian Belief Network to detect healthcare fraud
EXPERT SYSTEMS WITH APPLICATIONS(2024)
摘要
Healthcare fraud detection algorithms are mostly based on applying machine learning methods to payer-claims data transactions to detect fraudulent activities. However, claim transactions are often analyzed in isolation, disregarding the interconnections that naturally exist in the claims generated from a single unit such as the patient, payer, or provider. Fraud detection frameworks have untapped potential to aid downstream processes such as investigations and audits through transparent and interpretable machine learning models. In this study, we show how fraud detection frameworks can benefit from use of a graphical network model called Bayesian Belief Network (BBN) which exploits the relational structure of attributes in a transaction and has better interpretability properties than many competing machine learning methods. BBN model performance is found to be comparable to commonly utilized baseline models such as logistic regression and random forest after validation with a real-life insurance claims dataset. In particular, the F1 score of fraud class for the best performing BBN was 0.15. The proposed BBN model is also considered to be interpretable to auditors and investigators based on common sense qualitative evaluation.
更多查看译文
关键词
Medicaid,Fraud detection,Supervised learning,Probabilistic graphical models
AI 理解论文
溯源树
样例
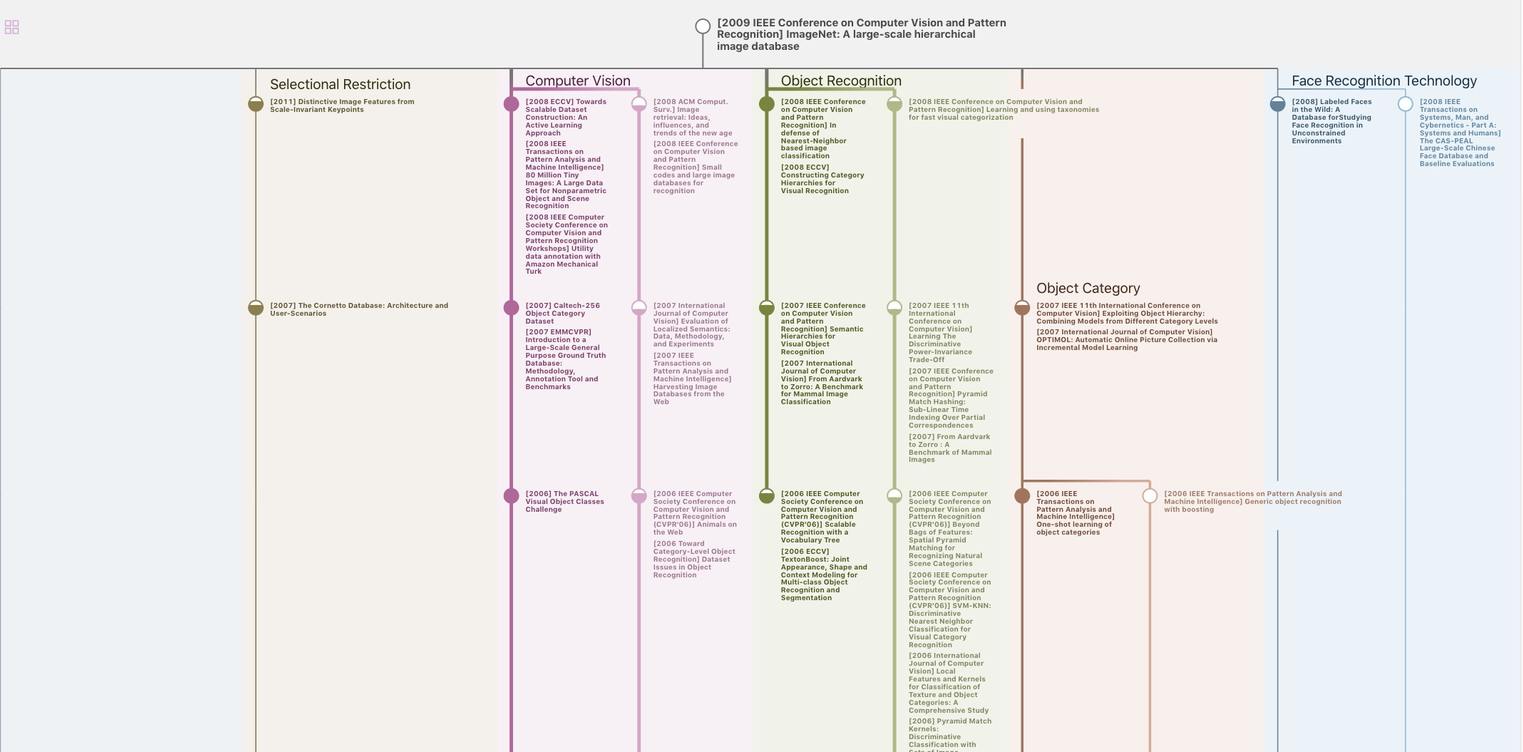
生成溯源树,研究论文发展脉络
Chat Paper
正在生成论文摘要