A high order fractal-based Kullback-Leibler divergence with application in classification
EXPERT SYSTEMS WITH APPLICATIONS(2024)
Abstract
Dempster-Shafer evidence theory (DSET) is extensively employed in multi-source data fusion applications. Nonetheless, when belief probability assignments (BPAs) exhibit considerable conflict, unexpected results can occur. To address this limitation, the high-order fractals are explored and a -order pound fractal-based Kullback- Leibler divergence (O-FKL) pound is introduced, which defines the -order pound as the optimal fractal epoch. This measure is employed to quantify the divergence between BPAs and demonstrates superior performance in assessing the conflict between two BPAs in numerical examples, compared to existing belief divergence methods. To utilize the O-FKL pound divergence measure to real-world problems, a novel O-FKL-based pound multi-source data fusion (O-FKL-MSDF) pound algorithm is designed. Through comparisons with well-known related methods, our proposed O-FKL-MSDF pound algorithm demonstrates superiority and enhanced robustness. Lastly, the O-FKL-MSDF pound algorithm is applied to real-world classification problems, underlining its high practical applicability.
MoreTranslated text
Key words
Dempster-Shafer evidence theory,High order KL divergence,Conflict management,Multi-source data fusion,Classification
AI Read Science
Must-Reading Tree
Example
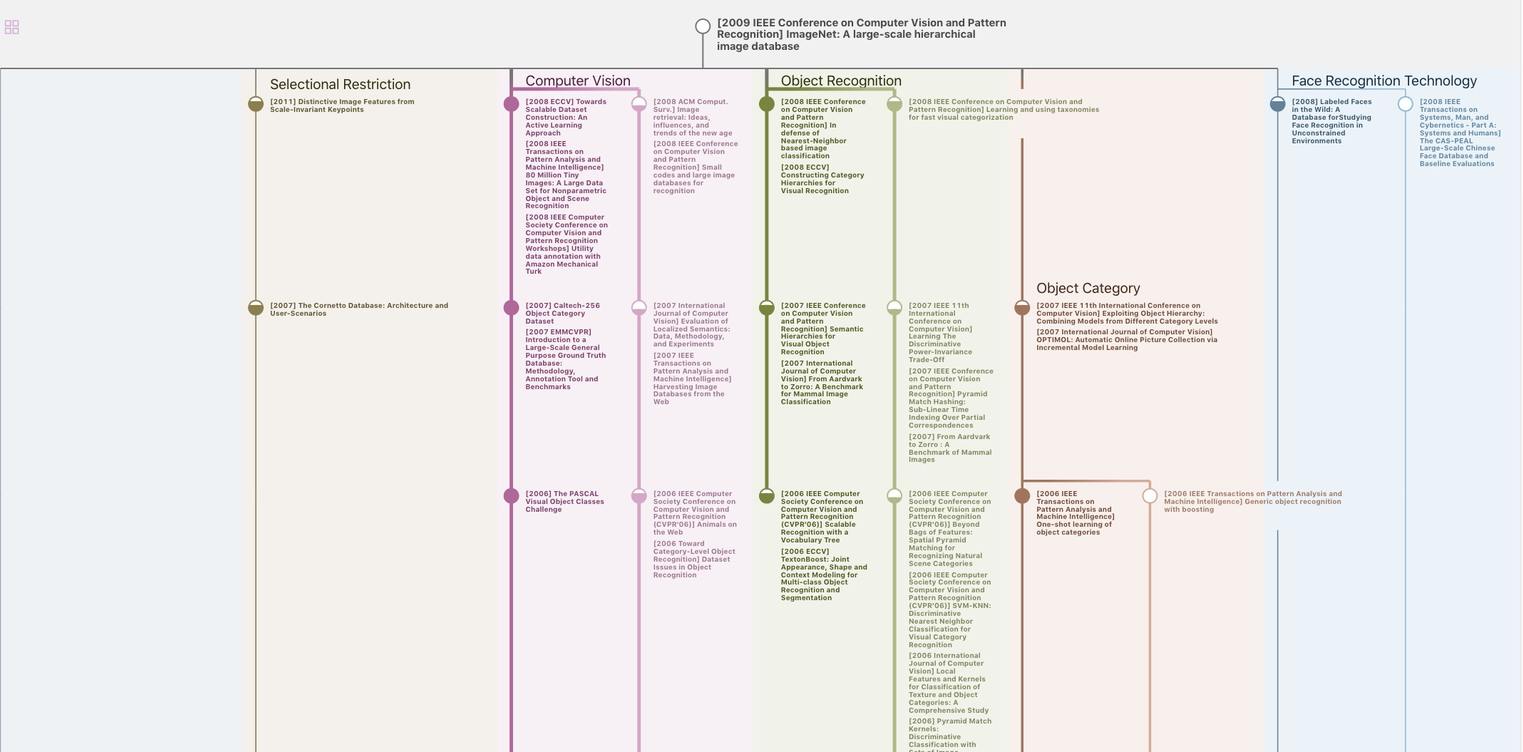
Generate MRT to find the research sequence of this paper
Chat Paper
Summary is being generated by the instructions you defined