Dynamic Object Classification of Low-Resolution Point Clouds: An LSTM-Based Ensemble Learning Approach
IEEE ROBOTICS AND AUTOMATION LETTERS(2023)
摘要
In unmanned vehicle perception, dynamic object classification is applied to classify objects accurately and timely, providing decision-making for obstacle avoidance and planning. Low-resolution LiDAR is one of the most important sensors for this task. Unfortunately, the existing approaches perform unsatisfactorily due to the huge domain gap between low-resolution and high-resolution point cloud classification. Some schemes try to reduce the gap by fusing multi-scan information through SLAM or completing single-scan point clouds. However, these methods rely on high positioning accuracy or the wholeness of object data. To this end, differently, we propose a dynamic object classification method of low-resolution data from the perspective of time-series fusion. By modeling time series of sparse data, we indicate change rules of separate classification models for object representation. Subsequently, based on ensemble learning, our method performs feature-level fusion on multiple networks to exploit their different expression capabilities. Finally, we utilize long short-term memory to gradually classify dynamic objects. Besides, we also propose a dataset of the low-resolution point clouds and manually annotate the ground truth, which contains abundant samples of cars, pedestrians, and motorcycles. Through testing actual low-resolution data, the accuracy of our method is verified to improve a lot than the state-of-the-art approaches.
更多查看译文
关键词
Recognition,computer vision for automation,low-resolution point clouds,ensemble learning,long short-term memory
AI 理解论文
溯源树
样例
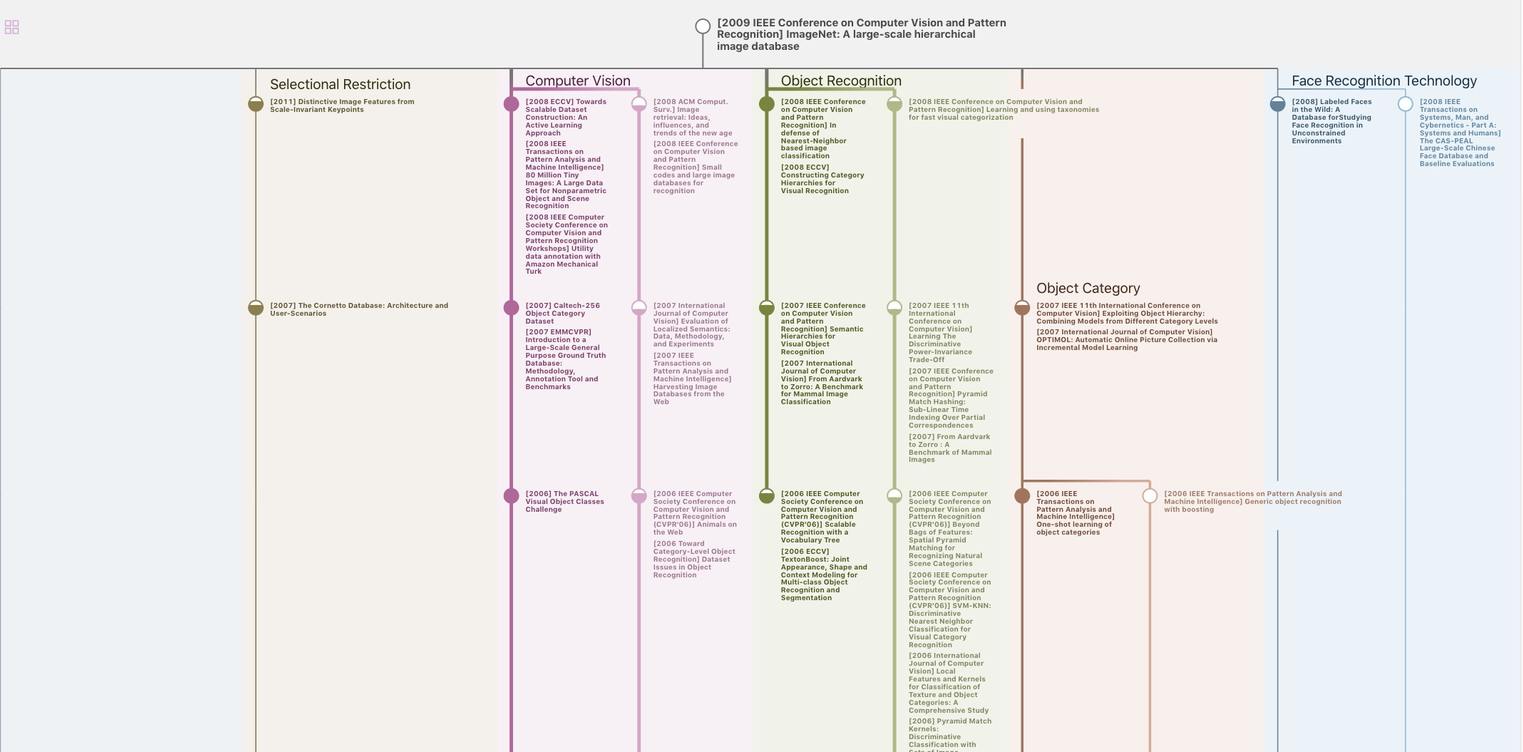
生成溯源树,研究论文发展脉络
Chat Paper
正在生成论文摘要