A Multifeature Combined Cloud-Snow Differentiation Algorithm for Gaofen-1 WFV Data Using Temperature, Temporal, and Spectral Characteristics
IEEE Journal of Selected Topics in Applied Earth Observations and Remote Sensing(2023)
摘要
Cloud detection plays a significant role in optical imagery, but snow has similar spectral characteristics to clouds in visible and near-infrared bands. The multifeature combined (MFC) algorithm was proposed for the Gaofen-1 (GF-1) wide-field (WFV) imaging system data, with only three visible bands and one near-infrared band. It shows great accuracy but performs poorly in snow-accumulated areas. Therefore, a multifeature combined cloud-snow differentiation (MFCSD) algorithm introducing temperature and temporal characteristics is proposed to further improve the overall accuracy of cloud detection for GF-1 WFV data. According to the different temperature, spatial, and temporal characteristics of clouds and snow, they can be distinguished by using land surface temperature (LST) data and multitemporal data. First, the cloud detection part of the MFC algorithm obtains cloud and snow mixed masks. Second, the Moderate Resolution Imaging Spectroradiometer (MODIS) LST product and time series of mixed masks are exploited to differentiate snow and clouds. When using the LST data to mark the potential snow cover areas, the average cloud detection accuracy of the verification data increases from 89.91% to 91.52% compared to MFC. After incorporating time series into the process, the average accuracy of cloud detection increases to 93.06%, and that of snow detection is 98.15% higher than MODIS snow cover. Thus, the MFCSD algorithm can well realize cloud detection and cloud-snow differentiation of GF-1 WFV data, and it can be applied to satellite data with similar limited band information with GF-1, such as SPOT-7, Quick Bird, and GF-6.
更多查看译文
关键词
Cloud detection, Gaofen-1 (GF-1), Nepal, snow detection
AI 理解论文
溯源树
样例
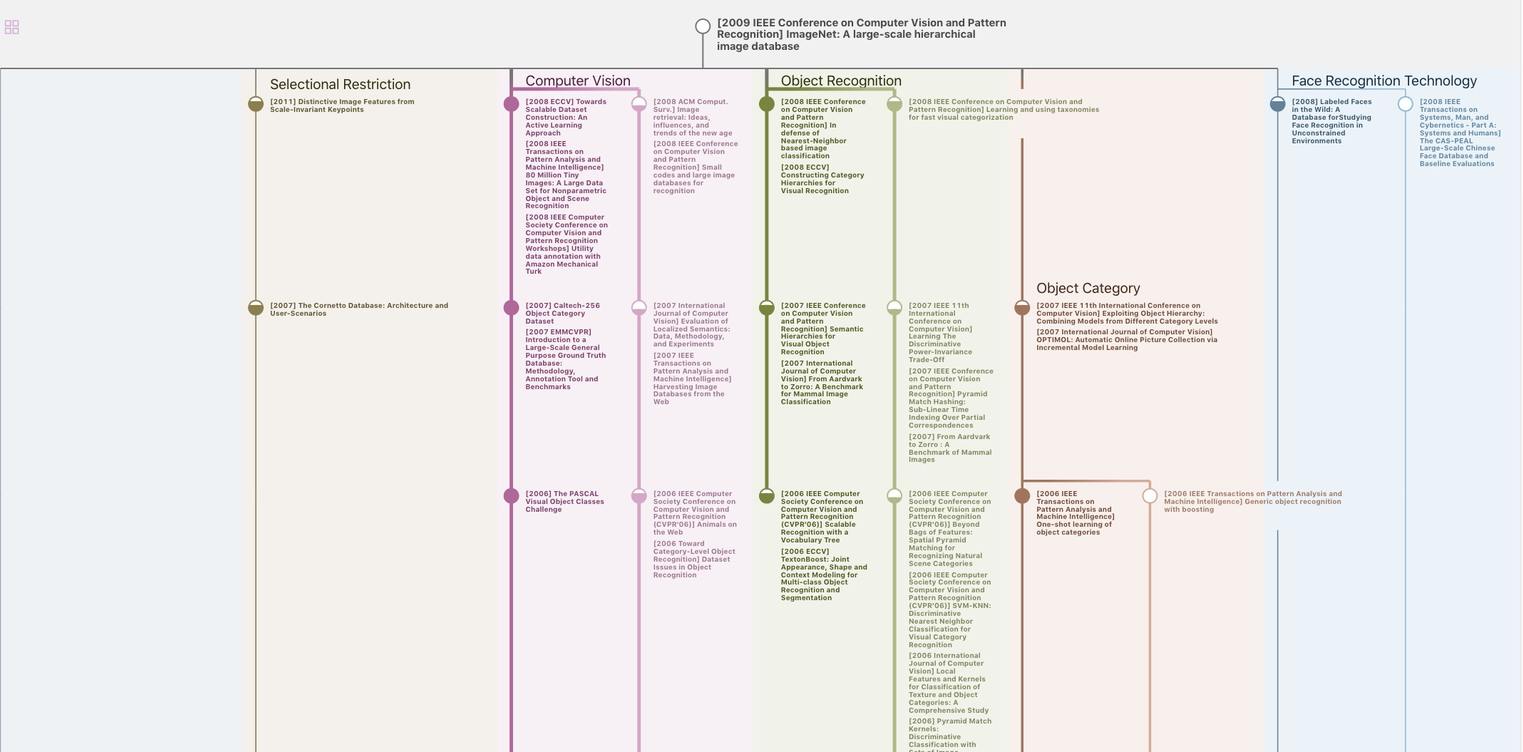
生成溯源树,研究论文发展脉络
Chat Paper
正在生成论文摘要