Sensing Indoor Lighting Environments and Analysing Dimension Reduction for Identification.
Workshop on Advances in Environmental Sensing Systems for Smart Cities(2023)
摘要
A generalized indoor light sensor can provide information to build and monitor indoor lighting arrangement that is aesthetically pleasing and conforming to the requirements set forth by the inhabitants. However, the identification of the surrounding lighting environment from the sensed parameters has some limitations and challenges. Till-to-date, classifiers are designed to identify only a single source, even in a multi-source environment. Classification based only on sensed values can be imperfect, as multi-type sources can share common parameters, or readings from a single source can fluctuate over time. The classification performances are mostly evaluated in controlled environments. In this work, we use a customised Bluetooth Low Energy (BLE) based light sensor that can sense and advertise major lighting parameters as instructed. Based on sensed parameters and adopting several Machine Learning (ML) and Neural Network (NN) based models off-board, we try to identify the singular and mixed presence of the four dissimilar types of sources: Incandescent, LED, CFL, and Sunlight in indoor surroundings. Off-board identification can get challenging where packet loss scenario is common. For that, we study how IoT devices with superior computational capability can utilise dimensional reduction techniques to minimize the required on-air traffic for classification. We then test classifiers with all those approaches both in controlled environments and real-world testbeds. The result shows that our best model can detect lighting environments with an accuracy of up to 98.22% in the controlled scenario and 83.33% in real-world testbeds.
更多查看译文
AI 理解论文
溯源树
样例
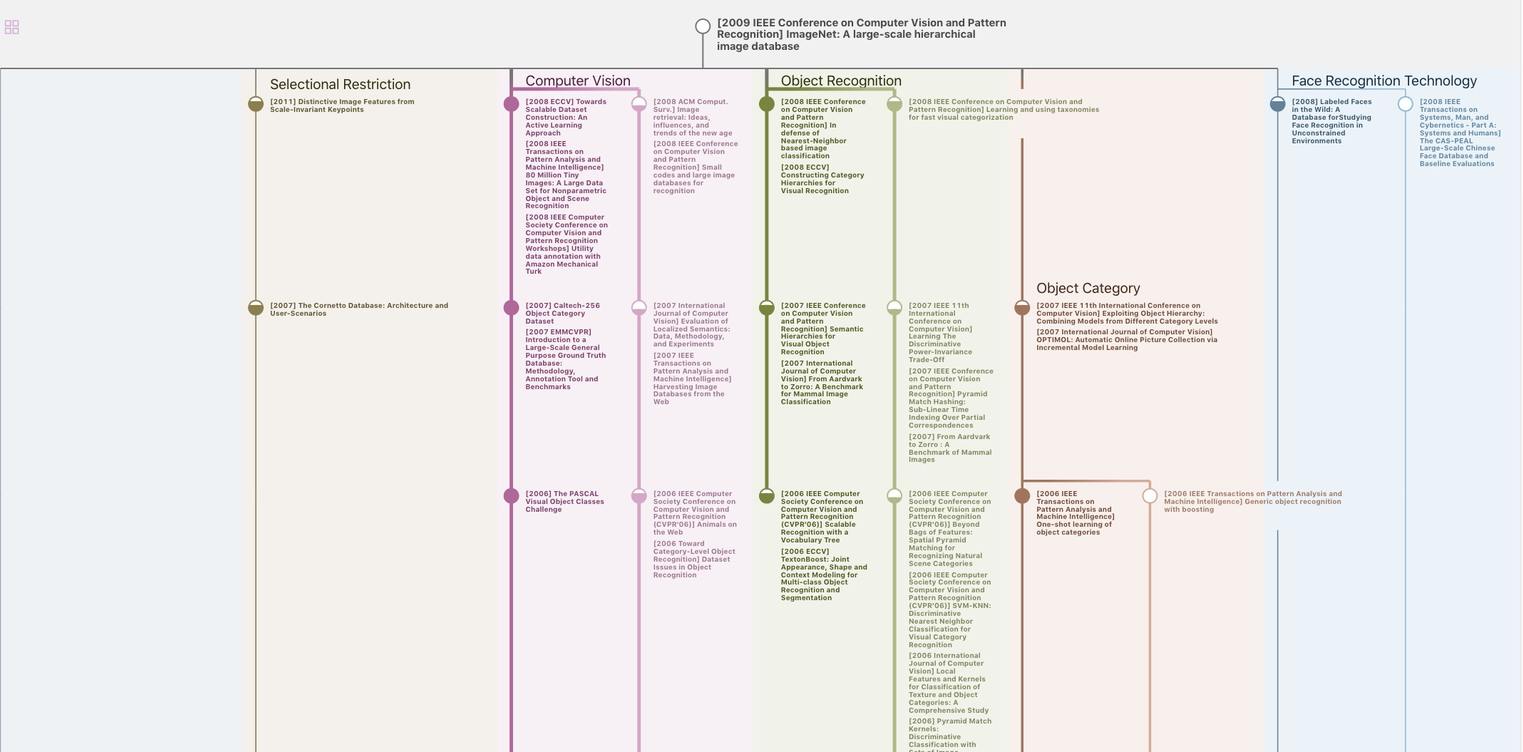
生成溯源树,研究论文发展脉络
Chat Paper
正在生成论文摘要