Multi-Scale Similarity Aggregation for Dynamic Metric Learning
MM '23: Proceedings of the 31st ACM International Conference on Multimedia(2023)
摘要
In this paper, we propose a new multi-scale similarity aggregation method (MSA) for dynamic metric learning (DyML), which adopts a pretraining-finetuning scheme and efficiently learns the similarity relationship for each semantic level. In particular, building upon the framework of self-supervised pretraining, the output embedding layer is divided into three learners to learn the similarity relations in each level individually. Then for training these learners, the hierarchical prior information is fully considered. Specifically, in light of the class hierarchy that each class in a coarse level corresponds to a set of subclasses in a finer level, multi-proxy learning is employed to facilitate the single-level similarity learning of each learner. On the other hand, following the hierarchical consistency property, a cross-level similarity constraint is further presented to encourage the estimated similarities of the three learners to be hierarchically consistent. Extensive experiments on three DyML datasets show that MSA significantly outperforms the existing state-of-the-art methods and allows for a better generalization for different semantic scales.
更多查看译文
AI 理解论文
溯源树
样例
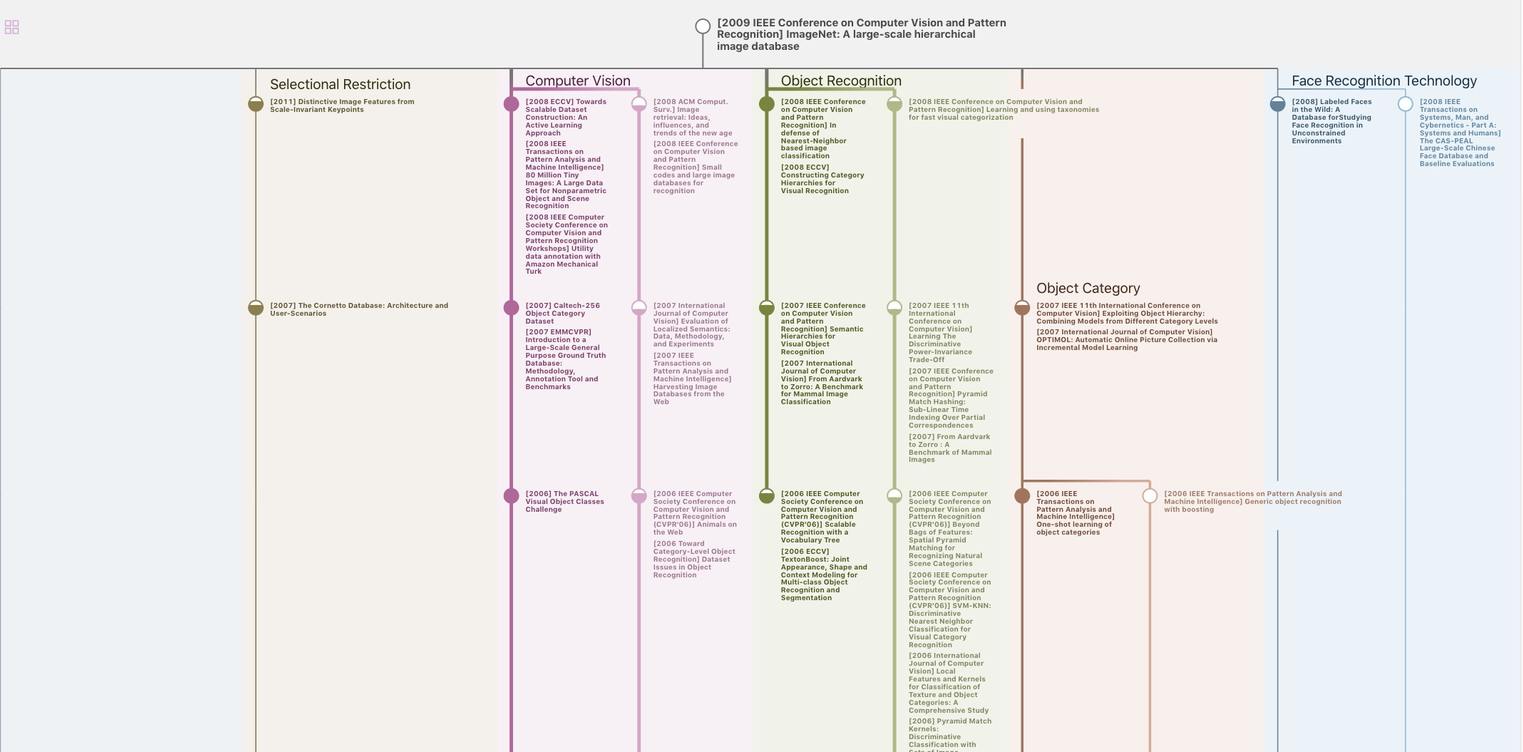
生成溯源树,研究论文发展脉络
Chat Paper
正在生成论文摘要