TIVA-KG: A Multimodal Knowledge Graph with Text, Image, Video and Audio
MM '23: Proceedings of the 31st ACM International Conference on Multimedia(2023)
摘要
Knowledge graphs serve as a powerful tool to boost model performances for various applications covering computer vision, natural language processing, multimedia data mining, etc. The process of knowledge acquisition for human is multimodal in essence, covering text, image, video and audio modalities. However, existing multimodal knowledge graphs fail to cover all these four elements simultaneously, severely limiting their expressive powers in performance improvement for downstream tasks. In this paper, we propose TIVA-KG, a multimodal Knowledge Graph covering Text, Image, Video and Audio, which can benefit various downstream tasks. Our proposed TIVA-KG has two significant advantages over existing knowledge graphs in i) coverage of up to four modalities including text, image, video, audio, and ii) capability of triplet grounding which grounds multimodal relations to triples instead of entities. We further design a Quadruple Embedding Baseline (QEB) model to validate the necessity and efficacy of considering four modalities in KG. We conduct extensive experiments to test the proposed TIVA-KG with various knowledge graph representation approaches over link prediction task, demonstrating the benefits and necessity of introducing multiple modalities and triplet grounding. TIVA-KG is expected to promote further research on mining multimodal knowledge graph as well as the relevant downstream tasks in the community. TIVA-KG is now available at our website: http://mn.cs.tsinghua.edu.cn/tivakg.
更多查看译文
AI 理解论文
溯源树
样例
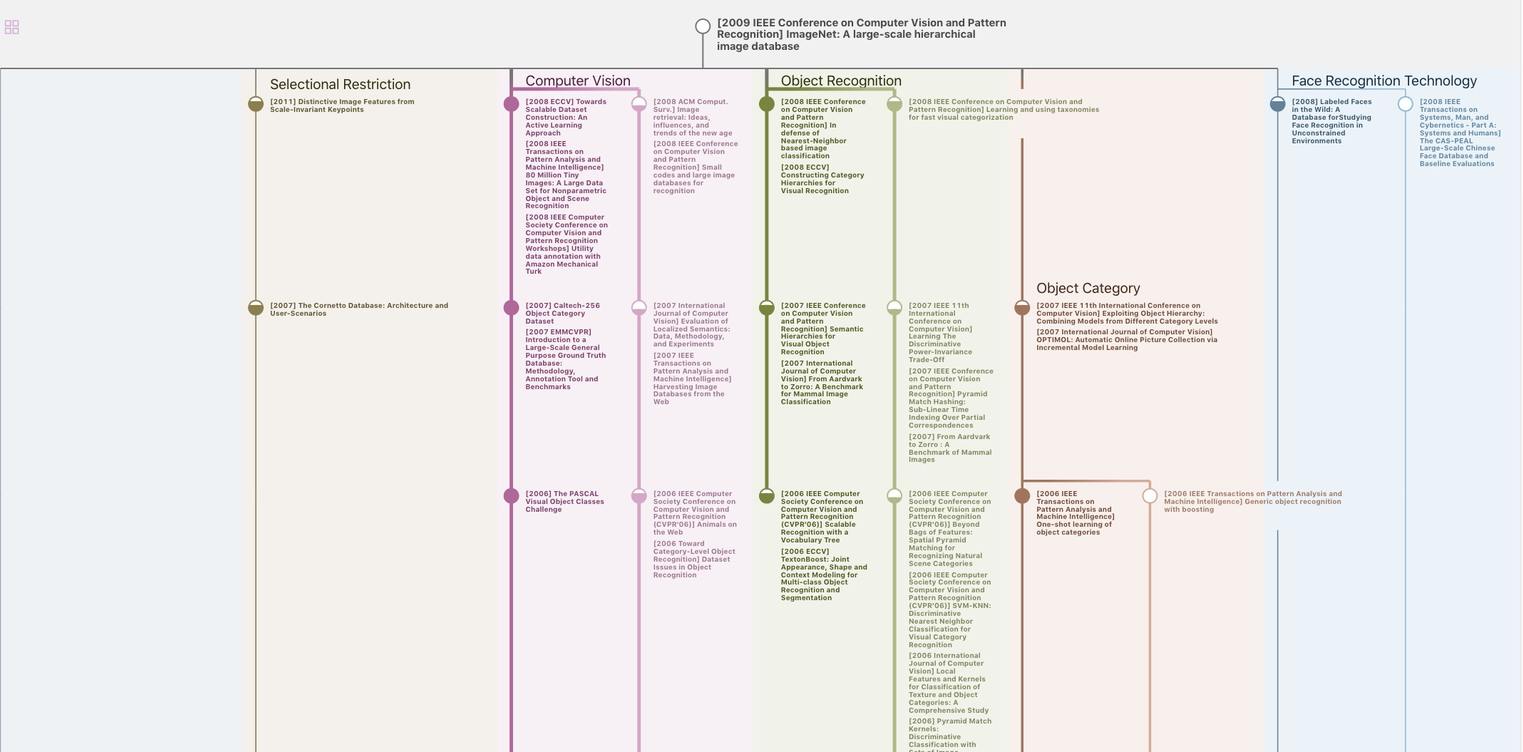
生成溯源树,研究论文发展脉络
Chat Paper
正在生成论文摘要