Client-Adaptive Cross-Model Reconstruction Network for Modality-Incomplete Multimodal Federated Learning
PROCEEDINGS OF THE 31ST ACM INTERNATIONAL CONFERENCE ON MULTIMEDIA, MM 2023(2023)
Abstract
Multimodal federated learning (MFL) is an emerging field that allows many distributed clients, each with multimodal data, to work together to train models targeting multimodal tasks without sharing local data. Whereas, existing methods assume that all modalities for each sample are complete, which limits their practicality. In this paper, we propose a Client-Adaptive Cross-Modal Reconstruction Network (CACMRN) to solve the modality-incomplete multimodal federated learning (MI-MFL). Compared to existing centralized methods for reconstructing missing modality, the local client data in federated learning is typically much less, which makes it challenging to train a reliable reconstruction model that can accurately predict missing data. We propose a cross-modal reconstruction transformer, which can prevent the model overfitting on the local client by exploring instance-instance relationships within the local client and utilizing normalized self-attention to conduct data-depended partial updating. Using federated optimization with alternative local updating and global aggregation, our method can not only collaboratively utilize the distributed data on different local clients to learn the cross-modal reconstruction transformer, but also prevent the reconstruction model from overfitting the data on the local client. Extensive experimental results on three datasets demonstrate the effectiveness of our method.
MoreTranslated text
Key words
Federated Learning,Multimodal,Missing Modality
AI Read Science
Must-Reading Tree
Example
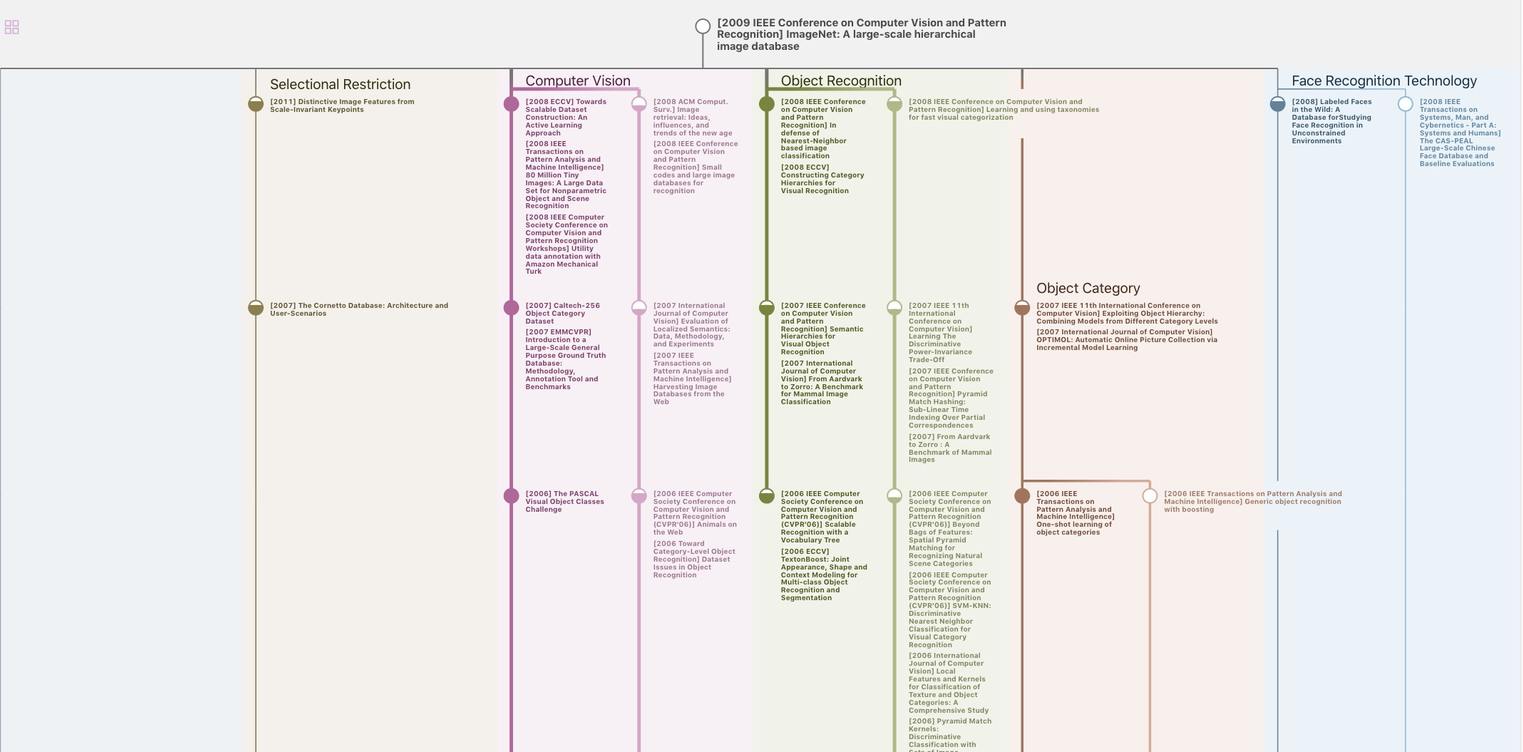
Generate MRT to find the research sequence of this paper
Chat Paper
Summary is being generated by the instructions you defined