IFS-SED: Incremental Few-Shot Sound Event Detection Using Explicit Learning and Calibration
MM '23: Proceedings of the 31st ACM International Conference on Multimedia(2023)
摘要
Sound event detection (SED) refers to recognizing the sound events in a continuous audio signal, which has drawn increasing interest during recent decades. The applications of SED seem to be evident in many fields, ranging from surveillance to monitoring applications. Despite the sustainable efforts that have been made, most of the previous attempts are performed on the closed-set, as only fixed and known sound event classes can be employed during the training. In this paper, we present our incremental few-shot SED framework under the open-set settings, as a practical machine listening system should be able to address unknown sound events. Specifically, an explicit learning and calibration-based multi-stage learning framework is utilized to address the challenges of catastrophic forgetting, and aim to achieve a better trade-off between stability and plasticity. To compress the model efficiently, the model prune and self-distillation paradigm are combined used for the model compression, thus our system can be deployed for the resource-limited devices. Our framework can also provide an uncertain estimation for the inference. Lastly, an interactive interface is presented to demonstrate the functions of our system.
更多查看译文
AI 理解论文
溯源树
样例
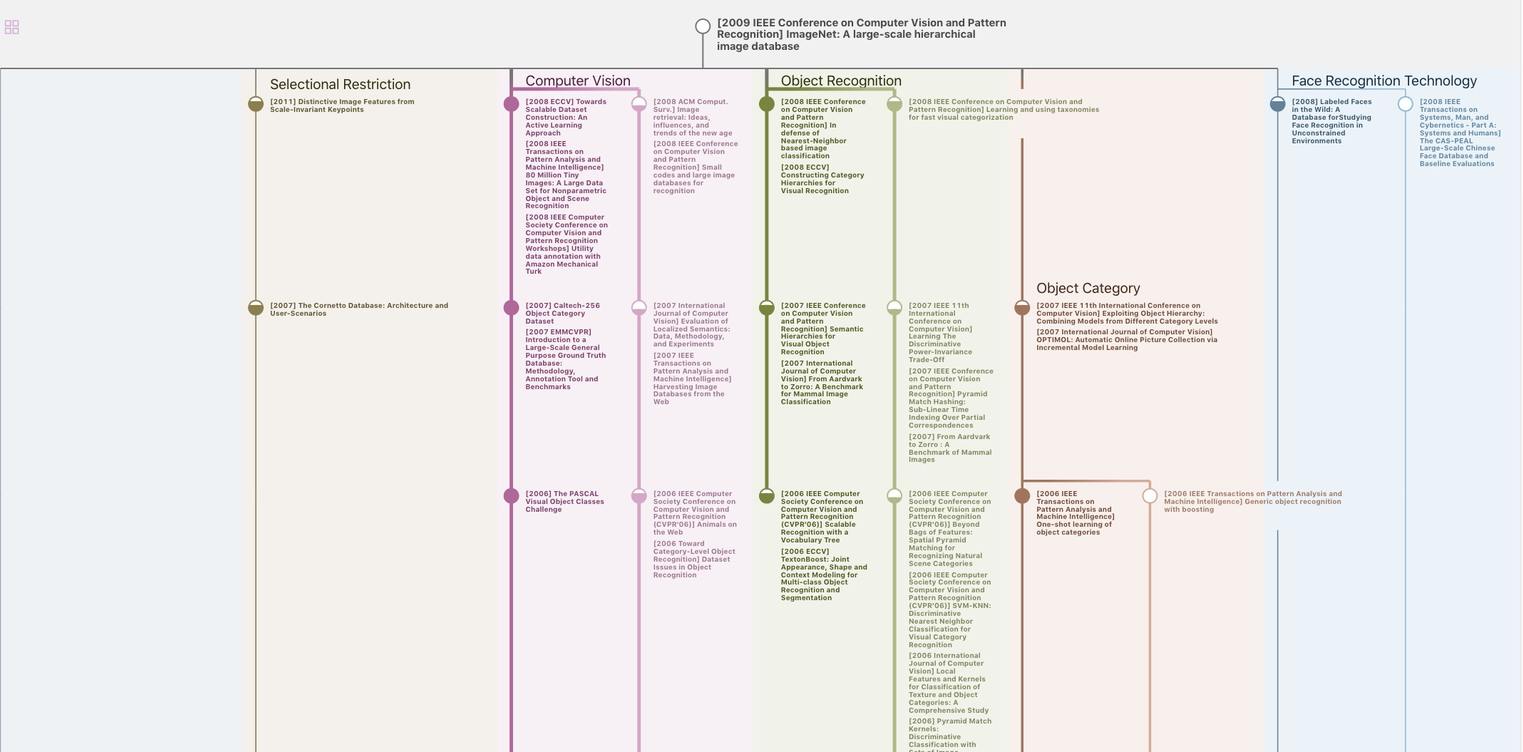
生成溯源树,研究论文发展脉络
Chat Paper
正在生成论文摘要