Towards robust and generalizable representations of extracellular data using contrastive learning.
NeurIPS(2023)
摘要
Contrastive learning is quickly becoming an essential tool in neuroscience for extracting robust and meaningful representations of neural activity. Despite numerous applications to neuronal population data, there has been little exploration of how these methods can be adapted to key primary data analysis tasks such as spike sorting or cell-type classification. In this work, we propose a novel contrastive learning framework, CEED (Contrastive Embeddings for Extracellular Data), for high-density extracellular recordings. We demonstrate that through careful design of the network architecture and data augmentations, it is possible to generically extract representations that far outperform current specialized approaches. We validate our method across multiple high-density extracellular recordings. All code used to run CEED can be found at https://github.com/ankitvishnu23/CEED.
### Competing Interest Statement
The authors have declared no competing interest.
更多查看译文
关键词
extracellular data,contrastive learning,generalizable representations
AI 理解论文
溯源树
样例
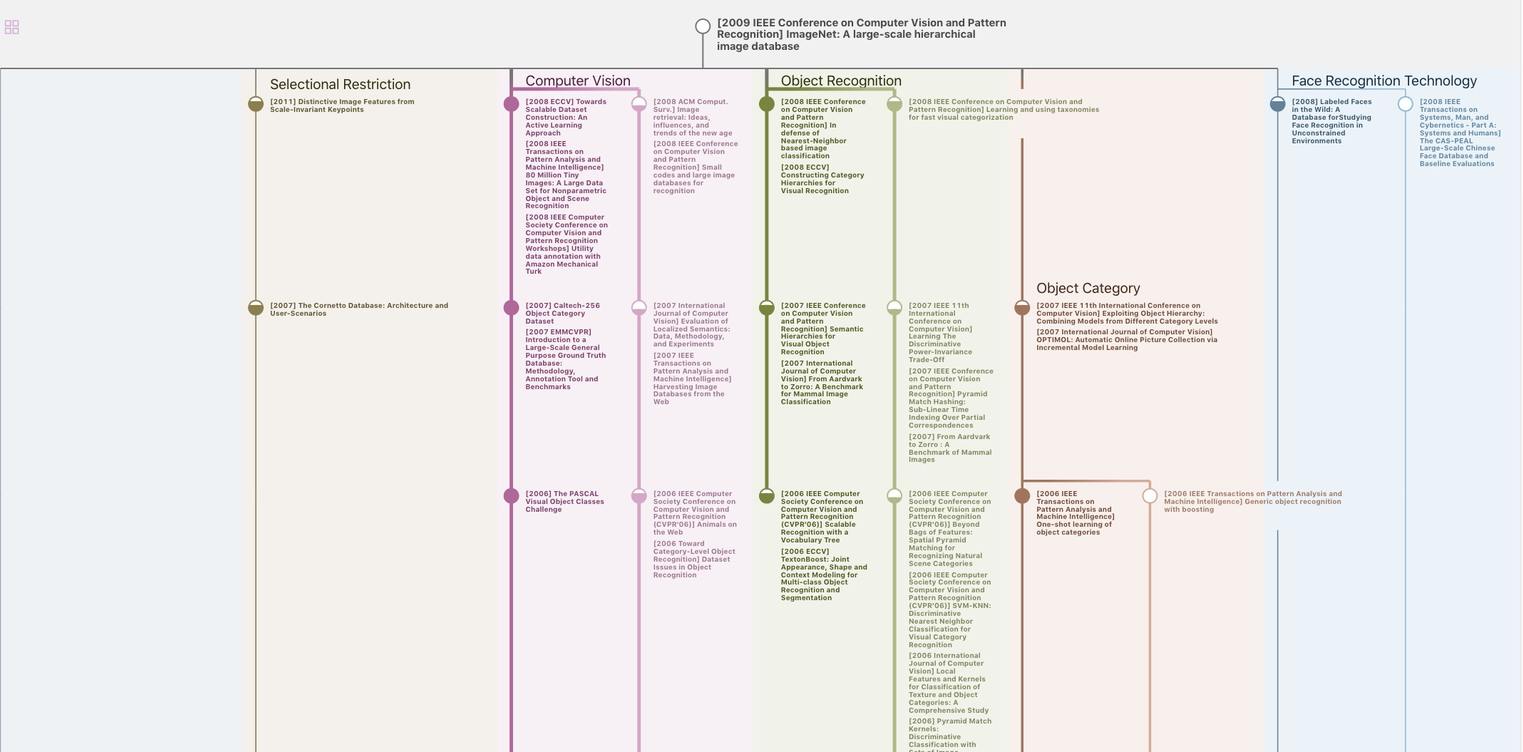
生成溯源树,研究论文发展脉络
Chat Paper
正在生成论文摘要