Evaluating the Effect of Common Annotation Faults on Object Detection Techniques.
IEEE International Symposium on Software Reliability Engineering(2023)
摘要
Machine learning (ML) is applied in many safety-critical domains such as autonomous driving and medical diagnosis. Many ML applications in such domains require object detection, which includes both classification and localization, to provide additional context. To ensure high accuracy, state-of-the-art object detection (OD) systems require large quantities of correctly annotated images for training. However, creating such datasets is non-trivial, may involve significant human effort, and is hence inevitably prone to annotation faults. We evaluate the effect of such faults on OD applications. We present ODFI, which can inject five different types of common annotation faults into any COCO-formatted dataset. We then use ODFI to inject these faults into two road traffic and one medical X-ray imaging datasets. Finally, using these faulty datasets, we systematically evaluate and compare the efficacy of existing OD techniques that are designed to be robust against such faults. To do so, we introduce a new metric that evaluates the robustness of OD models in the presence of faults. We find that (1) single-stage detectors trained with faulty annotations perform better in scenes with more objects, (2) redundant bounding boxes have the least impact on robustness, and (3) ensembles have the highest overall robustness among the robust OD techniques considered.
更多查看译文
关键词
Error resilience,Machine learning,Training
AI 理解论文
溯源树
样例
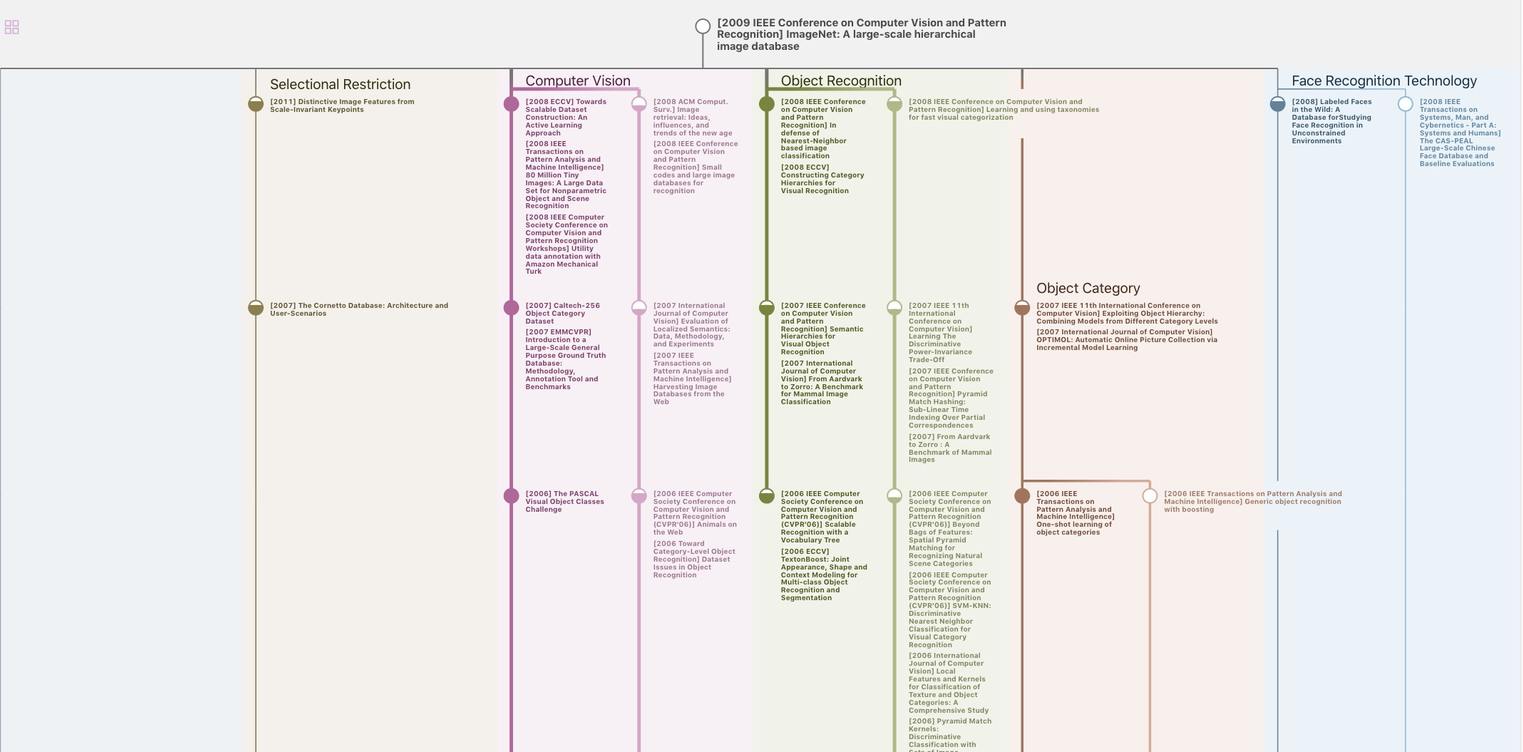
生成溯源树,研究论文发展脉络
Chat Paper
正在生成论文摘要