AutoKAD: Empowering KPI Anomaly Detection with Label-Free Deployment
2023 IEEE 34th International Symposium on Software Reliability Engineering (ISSRE)(2023)
摘要
Monitoring Key Performance Indicators (KPIs) and detecting anomalies in online service systems is critical. However, choosing the right KPI anomaly detection algorithm and appropriate hyperparameters presents a challenge. Conventional Automated Machine Learning (AutoML) struggles to address this because the hold-out dataset lacks labels and its loss doesn’t reliably reflect anomaly detection accuracy. To address the above challenges, this paper introduces AutoKAD, an AutoML framework designed to solve the combined algorithm selection and hyperparameter optimization problem for unsupervised KPI Anomaly Detection. We propose a label-free universal objective function, inspired by the Local Outlier Factor (LOF), for evaluating AutoML trials. Additionally, we improve the acquisition function and designs a cluster-based warm start strategy to enhance exploration effectiveness and efficiency. The experimental results on three real-world datasets show that our approach outperforms the SOTA model selection algorithm by 11% in F1-score and achieves comparable performance (99%) with theoretically optimal results. We believe that AutoKAD can greatly improve the deployment feasibility of existing anomaly detection algorithms in real-world systems. Our code is anonymously released at https://github.com/NetManAIOps/AutoKAD.
更多查看译文
关键词
Automated Machine Learning,Time Series Anomaly Detection,AIOps
AI 理解论文
溯源树
样例
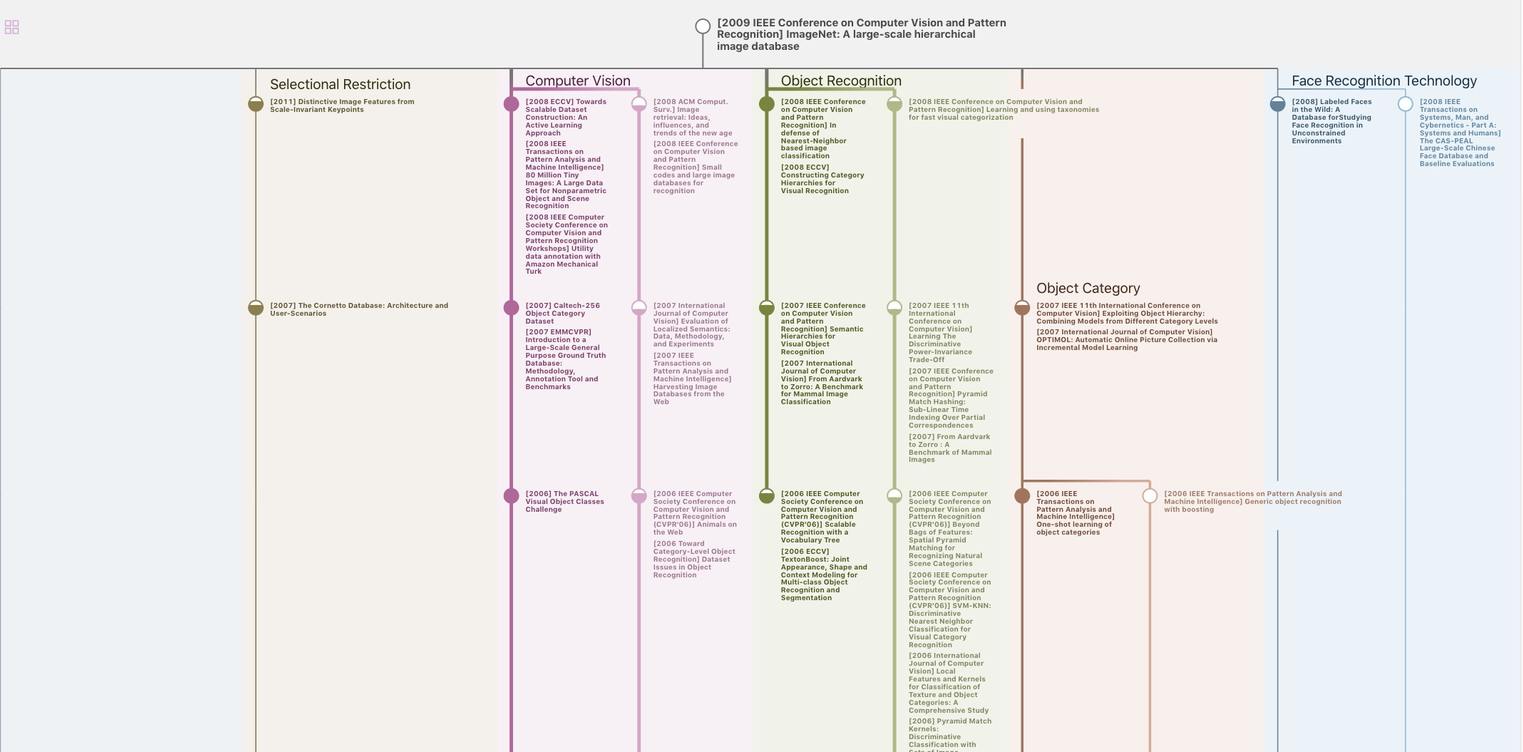
生成溯源树,研究论文发展脉络
Chat Paper
正在生成论文摘要