A Comparative Study of Deterministic and Stochastic Policies for Q-learning
2023 4th International Conference on Artificial Intelligence, Robotics and Control (AIRC)(2023)
Abstract
Q-learning is a form of reinforcement learning that employs agents to perform actions in an environment under a policy to reach ultimate goals. Q-learning is also thought as a goal-directed learning to maximize the expected value of the cumulative rewards via optimizing policies. Deterministic and scholastic policies are commonly used in reinforcement learning. However, they perform quite different in Markov decision processes. In this study, we conduct a comparative study on these two policies in the context of a grid world problem with Q-learning and provide an insight into the superiority of the deterministic policy over the scholastic one.
MoreTranslated text
Key words
Reinforcement Learning,Q-Learning,Markov Decision Process,Deterministic and stochastic policies,GridWorld
AI Read Science
Must-Reading Tree
Example
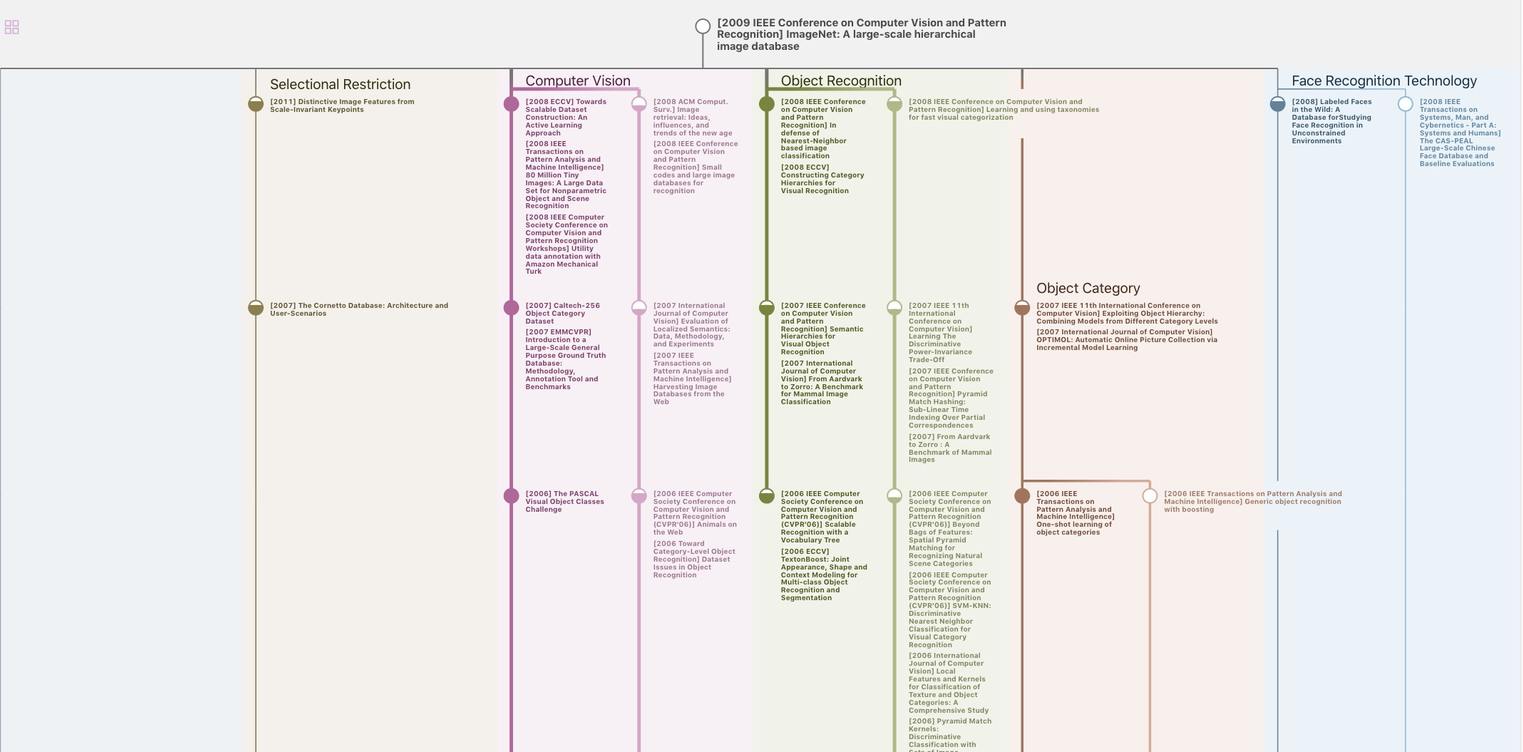
Generate MRT to find the research sequence of this paper
Chat Paper
Summary is being generated by the instructions you defined