Life prediction of electronic equipment based on the fusion of simulation data and measurement data
2023 IEEE AUTOTESTCON(2023)
摘要
The remaining useful life (RUL) prediction is one of the core technologies in the field of reliability engineering. In recent years, the emergence of long-life and high-reliability electronic equipment has posed a challenge to the life prediction of such equipment. Reliability data obtained alone by experimental tests or field observations have limitations. When we use data fusion methods, we can make full use of different types of data, save test cost, and improve prediction accuracy. At present, the commonly used data fusion methods are to merge multi-source data such as failure time information and degradation data under multi-stress conditions. Electronic devices have the characteristics of high cost of test execution and extensive sources of reliability data. For such characteristics, this paper presents a method that combines simulation data with measured data, The simulation data can be used as a priori information for reliability assessment of electronic equipment. When reliability tests are not conducted. In the absence of reliability test, the life distribution of the equipment can be approximated by simulation information, and the life distribution of the device can be updated according to the measurement data after a small amount of measurement information is obtained. The above idea can be realized by Bayes method. Firstly, we need to obtain the degradation/failure information of functional parameters of electronic equipment based on the degradation of key components in them. The linear Wiener process is used to model the degradation curve and inverse Gaussian distribution to model the failure-time data, then, we can get the prior distribution of model parameters under different simulation conditions. Secondly, to get some data in reality, we need to carry out reliability tests (ALT, ADT). Finally, the MCMC method is used to obtain the parameter estimation and confidence intervals, the correlation between parameters and the smoothness of the martingale chain need to test. After all these work, we give the remaining life prediction of the equipment and its reliability function. Sallen-Key bandpass filter is used to verify the practicality of the method and compare it with the extant models: (1) Self-contrast with the present model using only a small amount of field information or only simulation information, and the results show that the confidence interval for predicting the remaining life by combining the two sources of information is the narrowest at the same confidence level. The accuracy of prediction is higher than those two models.(2) Compared with the time series prediction models ARIMA and recurrent neural networks RNN, LSTM, GRU, the outstanding advantage of this method is that it can give an explicit expression of the degradation process of electronic equipment affected by stress-time, which can better solve the problems of overfitting of the training set and accumulation of multi-step prediction errors of the above-mentioned models, besides the sacrificing part of the training set fitting accuracy can be exchanged for higher test set accuracy, which can be better used in engineering practice.
更多查看译文
关键词
Multi-source data fusion,Bayes Method,Markov chain Monte Carlo(MCMC)
AI 理解论文
溯源树
样例
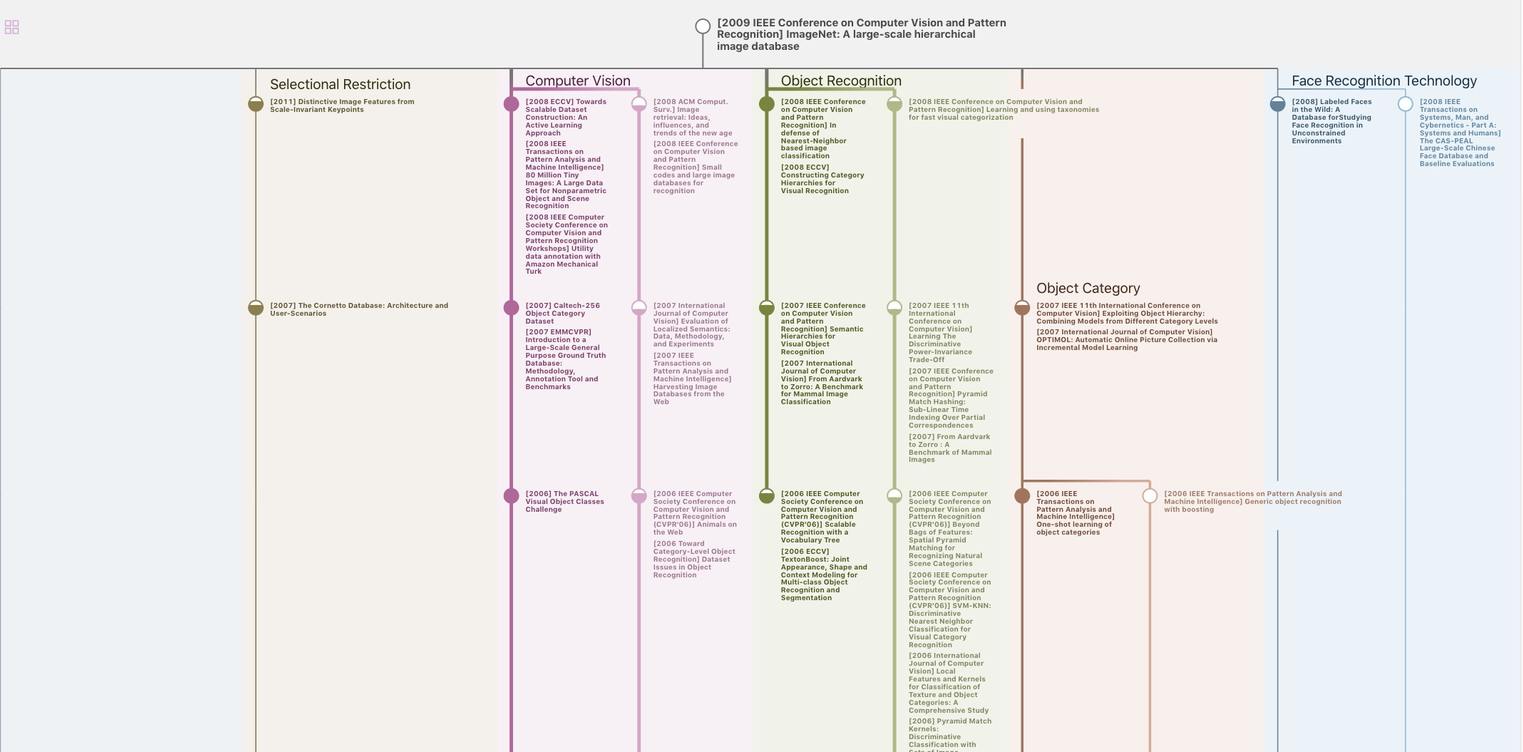
生成溯源树,研究论文发展脉络
Chat Paper
正在生成论文摘要