A Contrastive Learning Framework for Dual-Target Cross-Domain Recommendation
MM '23: Proceedings of the 31st ACM International Conference on Multimedia(2023)
摘要
Cross-Domain Recommendation (CDR) is proposed to address the long-standing data sparsity problem in recommender systems (RSs). Traditional CDR only leverages relatively richer information from an auxiliary domain to improve the performance in a sparser domain, which is also called single-target CDR. In recent years, dual-target CDR has been proposed to improve recommendation performance in both domains simultaneously. The existing dual-target CDR methods are based on common users to achieve knowledge transfer between domains. We argue that the existing methods face two challenges: (1) how to learn more representative user and item embeddings in each domain, and (2) in the case of a small number of common users in real-world datasets, how to achieve better knowledge transfer. To address these challenges, in this paper, we propose a contrastive learning (CL) framework, called CL-DTCDR. In CL-DTCDR, we first design a CL task in each domain to learn more representative user and item embeddings. Then, we further construct positive pairs of the user and her/his most similar user between domains to optimize user embeddings. By two CL tasks, CL-DTCDR effectively improves performance in both domains. Extensive experiments conducted on three real-world datasets demonstrate that CL-DTCDR significantly outperforms the state-of-the-art approaches.
更多查看译文
AI 理解论文
溯源树
样例
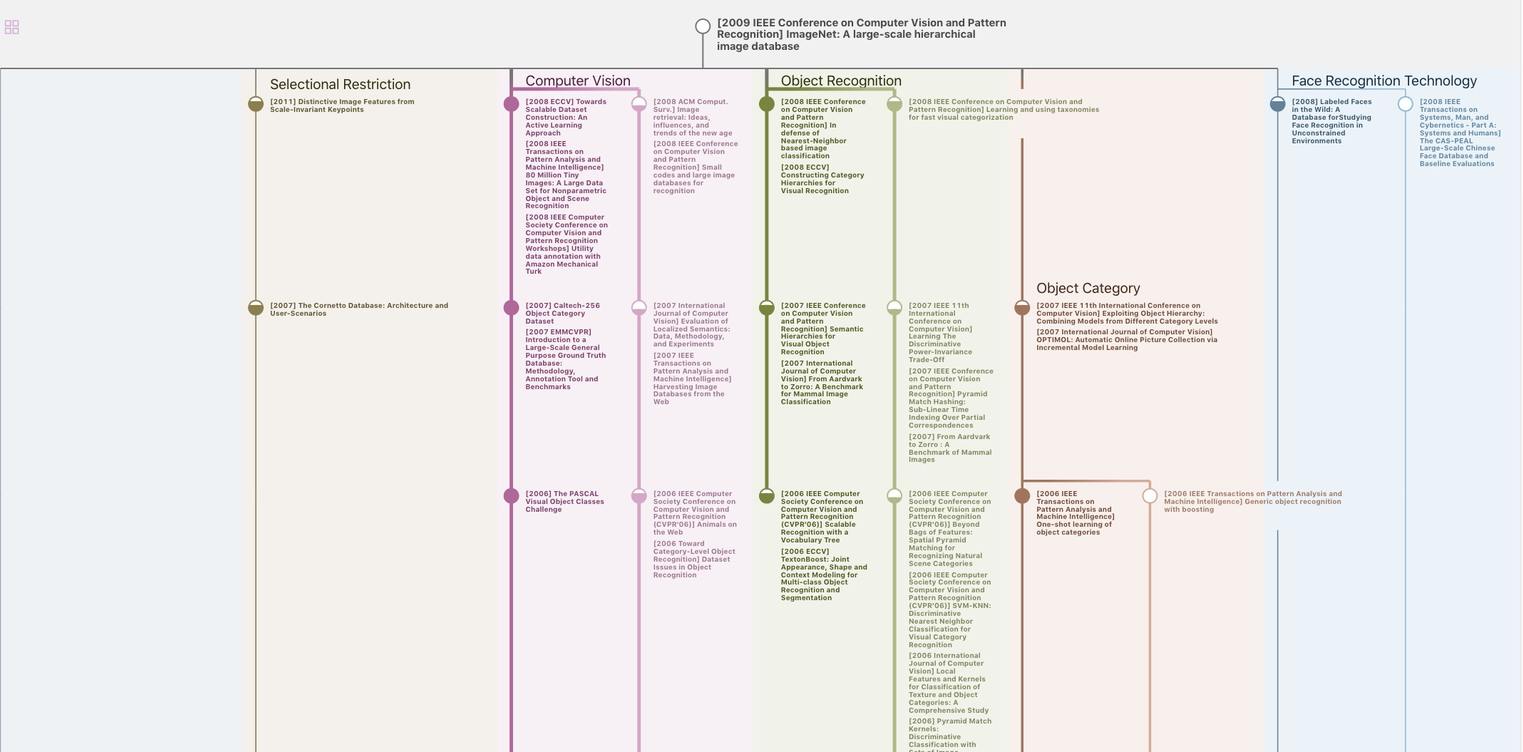
生成溯源树,研究论文发展脉络
Chat Paper
正在生成论文摘要