The Silent Manipulator: A Practical and Inaudible Backdoor Attack against Speech Recognition Systems
MM '23: Proceedings of the 31st ACM International Conference on Multimedia(2023)
摘要
Backdoor Attacks have been shown to pose significant threats to automatic speech recognition systems (ASRs). Existing success largely assumes backdoor triggering in the digital domain, or the victim will not notice the presence of triggering sounds in the physical domain. However, in practical victim-present scenarios, the over-the-air distortion of the backdoor trigger and the victim awareness raised by its audibility may invalidate such attacks. In this paper, we propose SMA, an inaudible grey-box backdoor attack that can be generalized to real-world scenarios where victims are present by exploiting both the vulnerability of microphones and neural networks. Specifically, we utilize the nonlinear effects of microphones to inject an inaudible ultrasonic trigger. To accurately characterize the microphone response to the crafted ultrasound, we construct a novel nonlinear transfer function for effective optimization. We also design optimization objectives to ensure triggers' robustness in the physical world and transferability on unseen ASR models. In practice, SMA can bypass the microphone's built-in filters and human perception, activating the implanted trigger in the ASRs inaudibly, regardless of whether the user is speaking. Extensive experiments show that the attack success rate of SMA can reach nearly 100% in the digital domain and over 85% against most microphones in the physical domains by only poisoning about 0.5% of the training audio dataset. Moreover, our attack can resist typical defense countermeasures to backdoor attacks.
更多查看译文
AI 理解论文
溯源树
样例
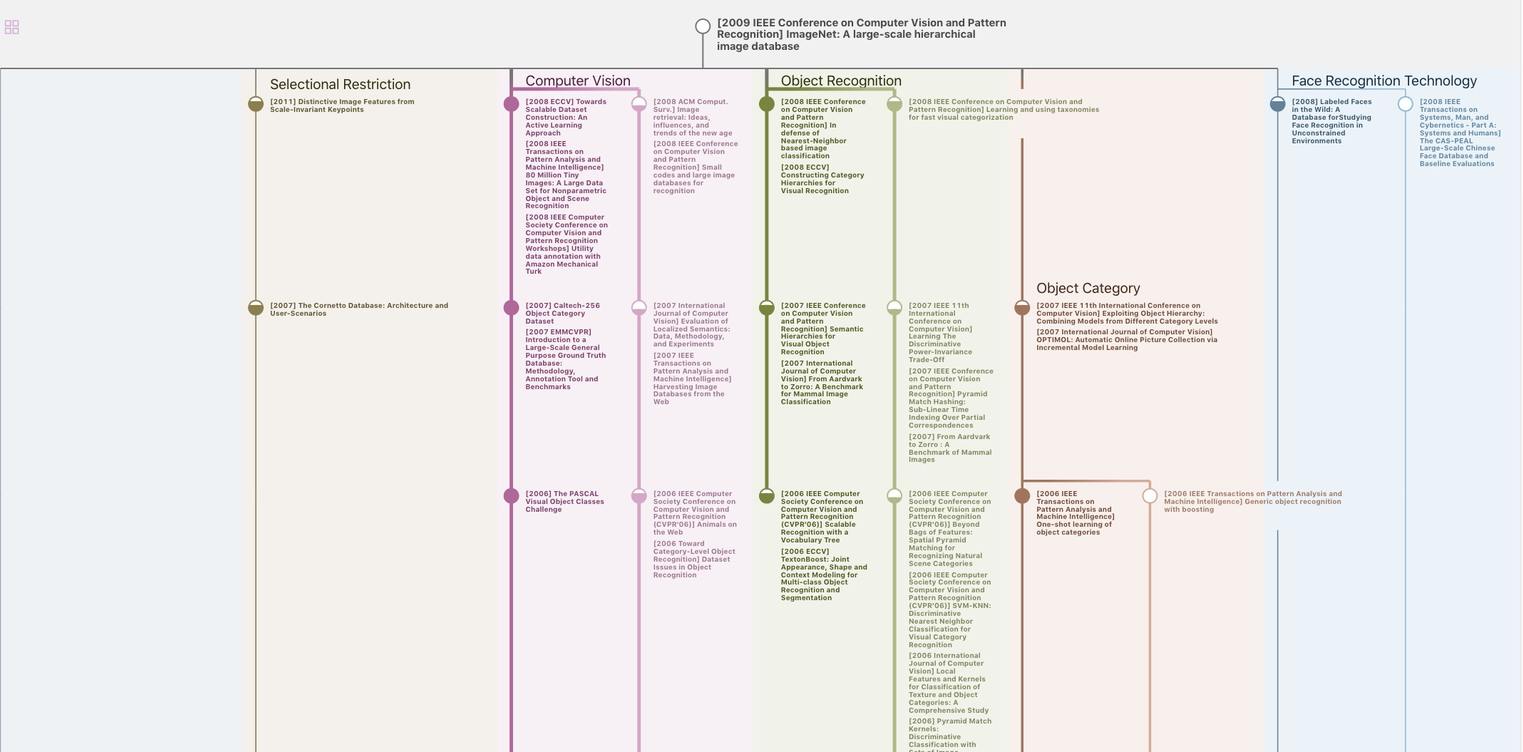
生成溯源树,研究论文发展脉络
Chat Paper
正在生成论文摘要