Separable Modulation Network for Efficient Image Super-Resolution
MM '23: Proceedings of the 31st ACM International Conference on Multimedia(2023)
摘要
Deep learning-based models have demonstrated unprecedented success in image super-resolution (SR) tasks. However, more attention has been paid to lightweight SR models lately, due to the increasing demand for on-device inference. In this paper, we propose a novel Separable Modulation Network (SMN) for efficient image SR. The key parts of the SMN are the Separable Modulation Unit (SMU) and the Locality Self-enhanced Network (LSN). SMU enables global relational interactions but significantly eases the process by separating spatial modulation from channel aggregation, hence making the long-range interaction efficient. Specifically, spatial modulation extracts global contexts from spatial, and channel aggregation condenses all global context features into the channel modulator, ultimately the aggregated contexts are fused into the final features. In addition, LSN allows guiding the network to focus on more refined image attributes by encoding local contextual information. By coupling two complementary components, SMN can capture both short- and long-range contexts for accurate image reconstruction. Extensive experimental results demonstrate that our SMN achieves state-of-the-art performance among the existing efficient SR methods with less complexity.
更多查看译文
AI 理解论文
溯源树
样例
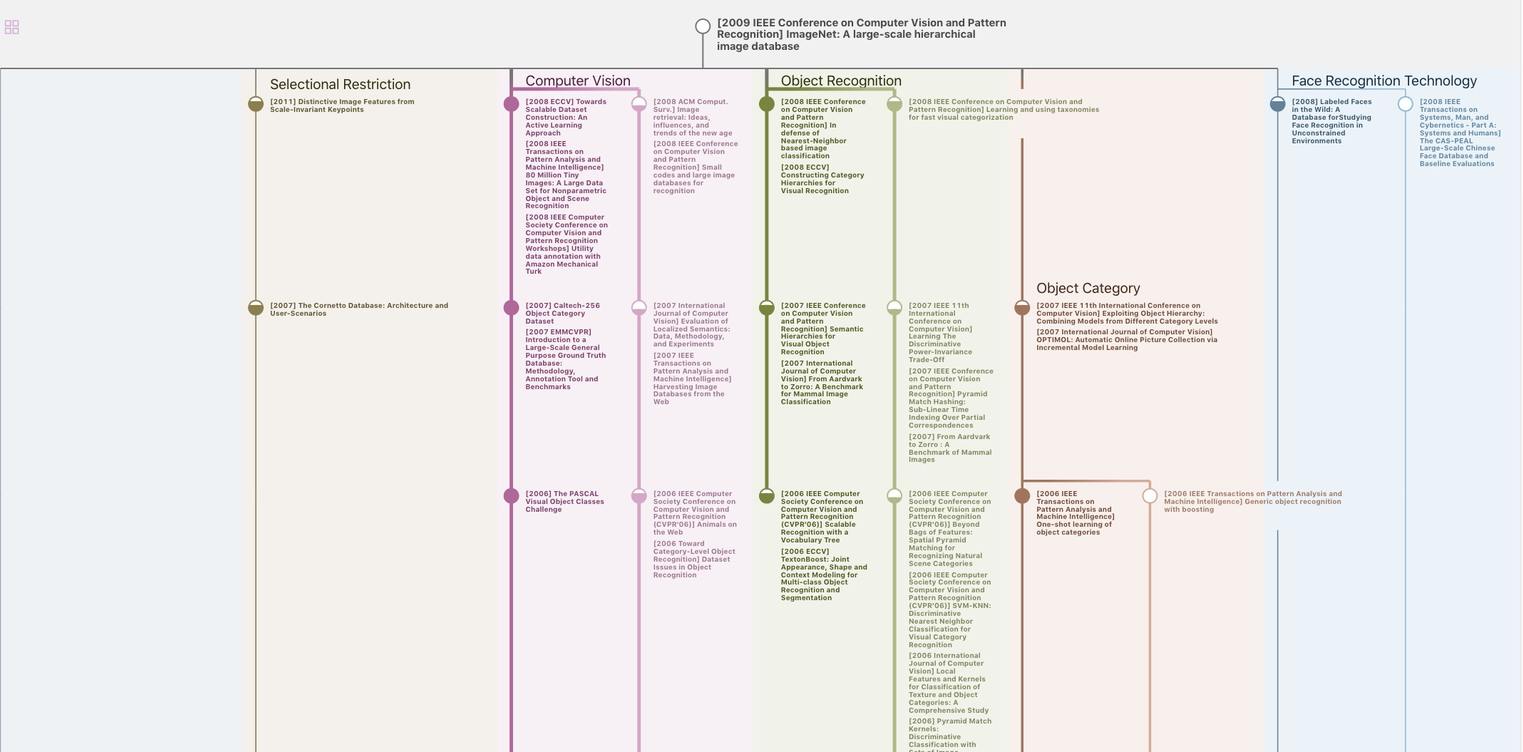
生成溯源树,研究论文发展脉络
Chat Paper
正在生成论文摘要