Learning from Easy to Hard Pairs: Multi-step Reasoning Network for Human-Object Interaction Detection
MM '23: Proceedings of the 31st ACM International Conference on Multimedia(2023)
摘要
Human-object interaction (HOI) detection aims to interpret the interactions of human-object pairs. Existing methods adopt a one-step reasoning paradigm that simultaneously outputs multi-label results for all HOI pairs without distinguishing difficulties. However, there are significant variations among HOI pairs in the same image, making their performance degrade in challenging situations. In this paper, we argue that the model should prioritize hard samples after inferring easy ones, and hard samples can benefit from easy ones. To this end, we propose a novel Multi-step Reasoning Network that progressively learns from easy to hard samples. In particular, an Easy-to-Hard Learning Block is introduced to enhance the representation of hard HOI pairs by prior associations. Additionally, we propose a Multi-step Reasoning Probability Transfer mechanism to enhance multi-label interaction classifications, which leverages cognitive associations and semantic dependencies. Extensive experiments demonstrate that our method outperforms other state-of-the-art on two challenging benchmark datasets.
更多查看译文
AI 理解论文
溯源树
样例
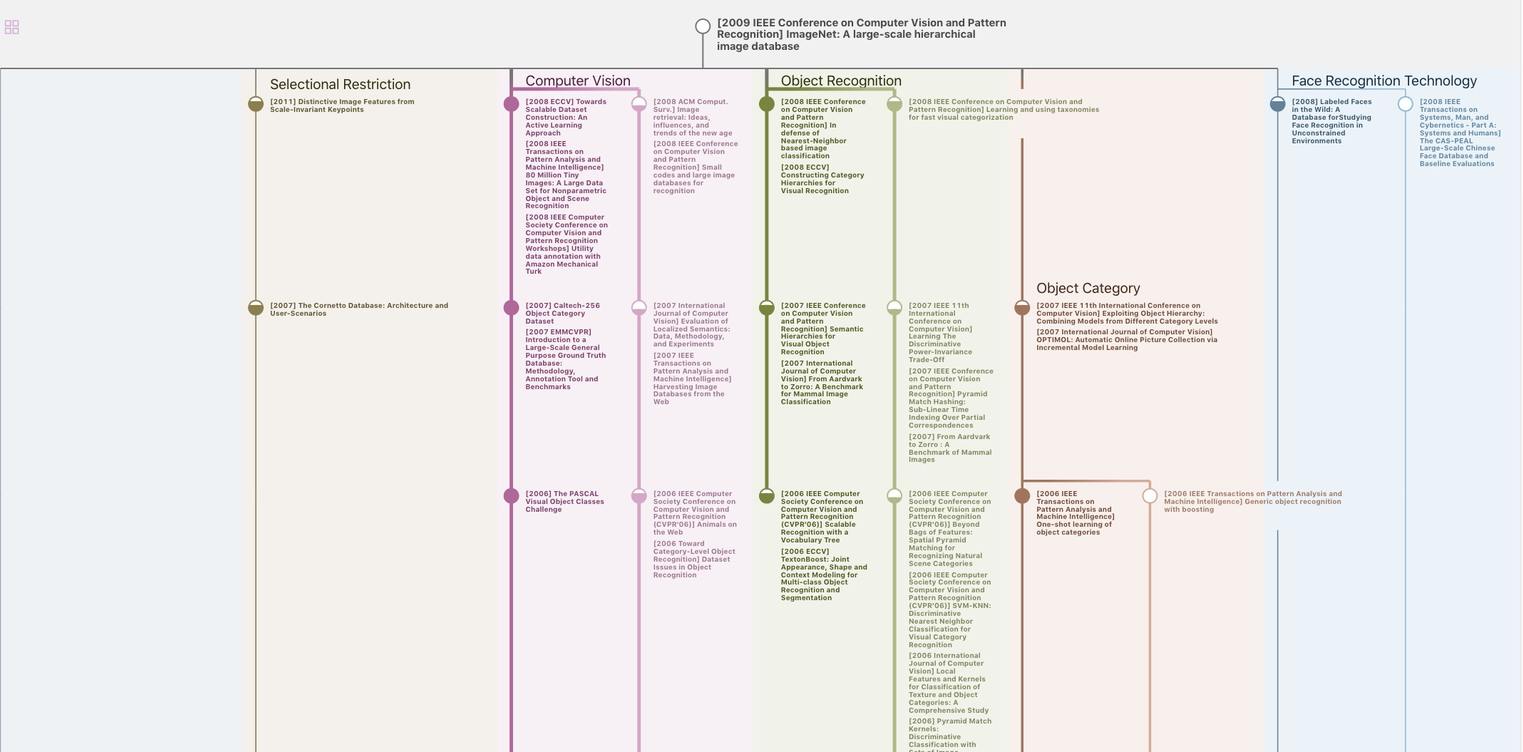
生成溯源树,研究论文发展脉络
Chat Paper
正在生成论文摘要