FedCD: A Classifier Debiased Federated Learning Framework for Non-IID Data
MM '23: Proceedings of the 31st ACM International Conference on Multimedia(2023)
摘要
One big challenge to federated learning is the non-IID data distribution caused by imbalanced classes. Existing federated learning approaches tend to bias towards classes containing a larger number of samples during local updates, which causes unwanted drift in the local classifiers. To address this issue, we propose a classifier debiased federated learning framework named FedCD for non-IID data. We introduce a novel hierarchical prototype contrastive learning strategy to learn fine-grained prototypes for each class. The prototypes characterize the sample distribution within each class, which helps align the features learned in the representation layer of every client's local model. At the representation layer, we use fine-grained prototypes to rebalance the class distribution on each client and rectify the classification layer of each local model. To alleviate the bias of the classification layer of the local models, we incorporate a global information distillation method to enable the local classifier to learn decoupled global classification information. We also adaptively aggregate the class-level classifiers based on their quality to reduce the impact of unreliable classes in each aggregated classifier. This mitigates the impact of client-side classifier bias on the global classifier. Comprehensive experiments conducted on various datasets show that our method, FedCD, effectively corrects classifier bias and outperforms state-of-the-art federated learning methods.
更多查看译文
AI 理解论文
溯源树
样例
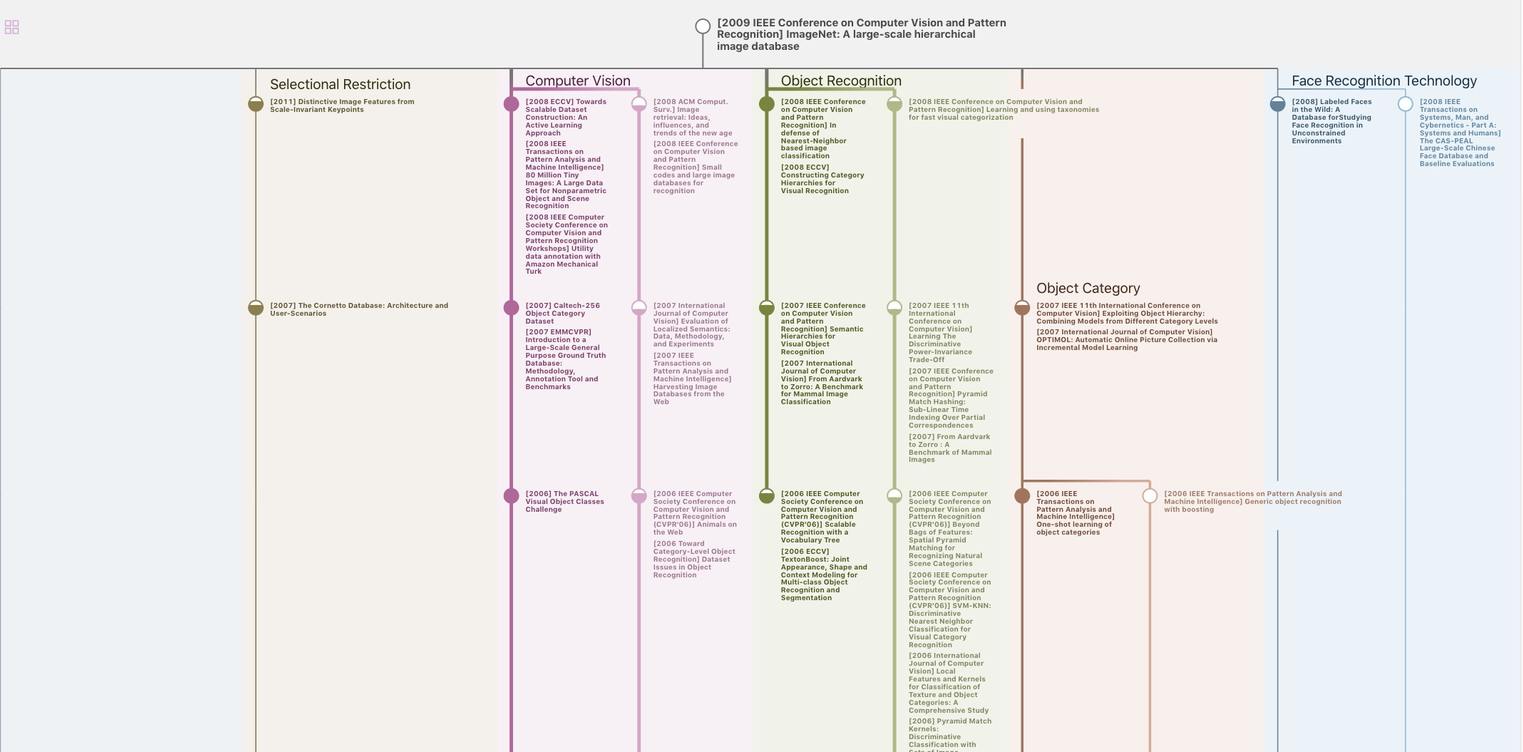
生成溯源树,研究论文发展脉络
Chat Paper
正在生成论文摘要