HELIOS: Hyper-Relational Schema Modeling from Knowledge Graphs
MM '23: Proceedings of the 31st ACM International Conference on Multimedia(2023)
摘要
Knowledge graph (KG) schema, which prescribes a high-level structure and semantics of a KG, is significantly helpful for KG completion and reasoning problems. Despite its usefulness, open-domain KGs do not practically have a unified and fixed schema. Existing approaches usually extract schema information using entity types from a KG where each entity e can be associated with a set of types {Te, by either heuristically taking one type for each entity or exhaustively combining the types of all entities in a fact (to get entity-typed tuples, (h_type, r, t_type) for example). However, these two approaches either overlook the role of multiple types of a single entity across different facts or introduce non-negligible noise as not all the type combinations actually support the fact, thus failing to capture the sophisticated schema information. Against this background, we study the problem of modeling hyper-relational schema, which is formulated as mixed hyper-relational tuples ({Th}, r, {Tt}, k, {Tv1},...) with two-fold hyper-relations: each type set T may contain multiple types and each schema tuple may contain multiple key-type set pairs (k, Tv). To address this problem, we propose HELIOS, a hyper-relational schema model designed to subtly learn from such hyper-relational schema tuples by capturing not only the correlation between multiple types of a single entity, but also the correlation between types of different entities and relations in a schema tuple. We evaluate HELIOS on three real-world KG datasets in different schema prediction tasks. Results show that HELIOS consistently outperforms state-of-the-art hyper-relational link prediction techniques by 20.0-29.7%, and is also much more robust than baselines in predicting types and relations across different positions in a hyper-relational schema tuple.
更多查看译文
AI 理解论文
溯源树
样例
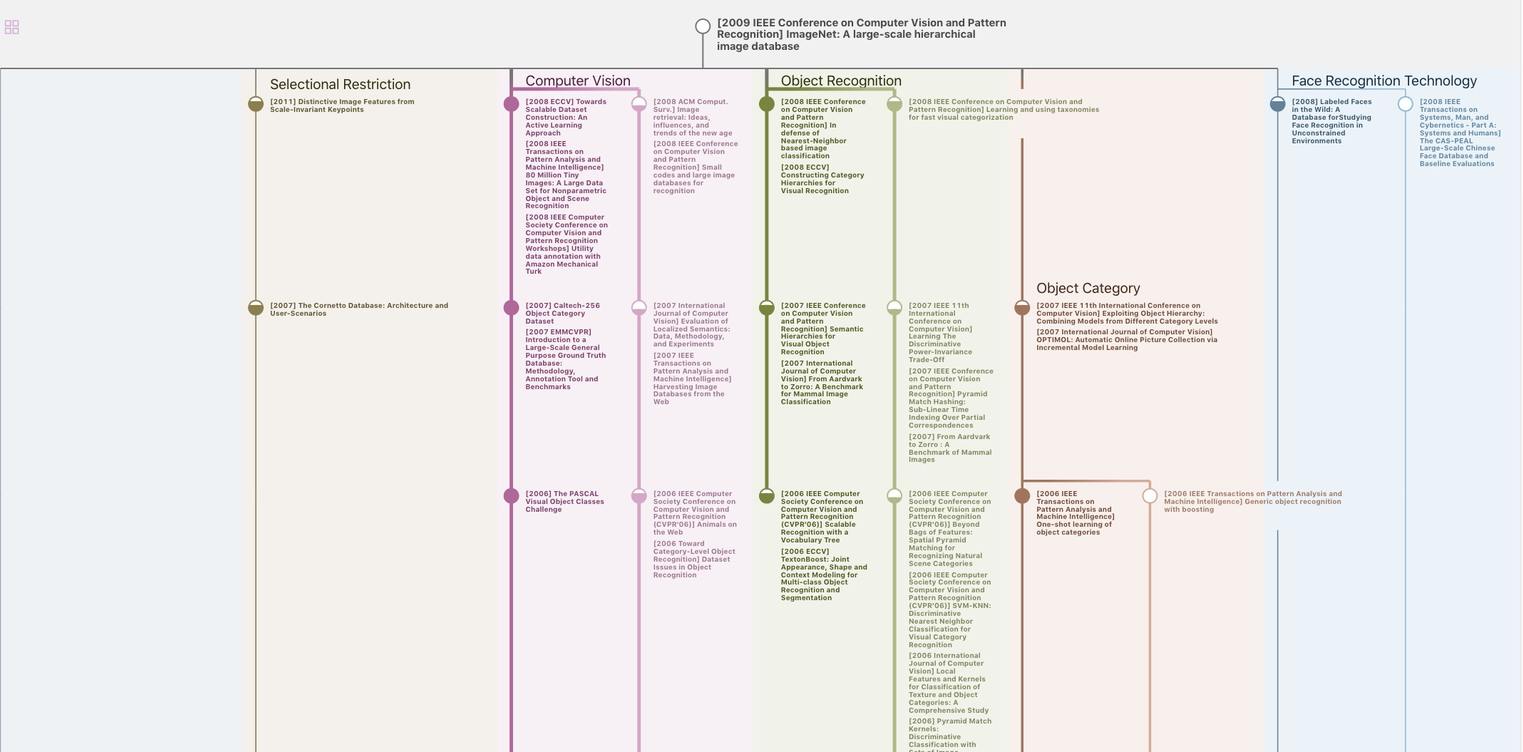
生成溯源树,研究论文发展脉络
Chat Paper
正在生成论文摘要