Preserving Local and Global Information: An Effective Metric-based Subspace Clustering
MM '23: Proceedings of the 31st ACM International Conference on Multimedia(2023)
摘要
Subspace clustering, which recoveries the subspace representation in the form of an affinity graph, has drawn tons of attention due to its effectiveness in various clustering tasks. However, existing subspace clustering methods are usually fed with raw data, which may lead to a suboptimal result since it is difficult to directly and accurately depict the inherent relation between data points. In this paper, we propose a novel subspace clustering method by holistically utilizing the pairwise similarity and graph geometric structure. Our model first constructs an initial subspace representation by means of self-expression, which is able to depict the global structure of data. Then, we use an effective metric to recover an intrinsic matrix with pairwise similarity based on the obtained representation, which further preserves the local structure. Besides, we propose to facilitate the downstream subspace learning task by searching for a smooth representation of the original data, which is obtained by applying a low-pass filter to retain the graph geometric features. By leveraging the subtasks of learning the smooth representation, performing the subspace learning, and recovering the intrinsic similarity matrix in a unified learning framework, each subtask can be alternately boosted. Experiments on several benchmark data sets have been conducted to verify the proposed method.
更多查看译文
AI 理解论文
溯源树
样例
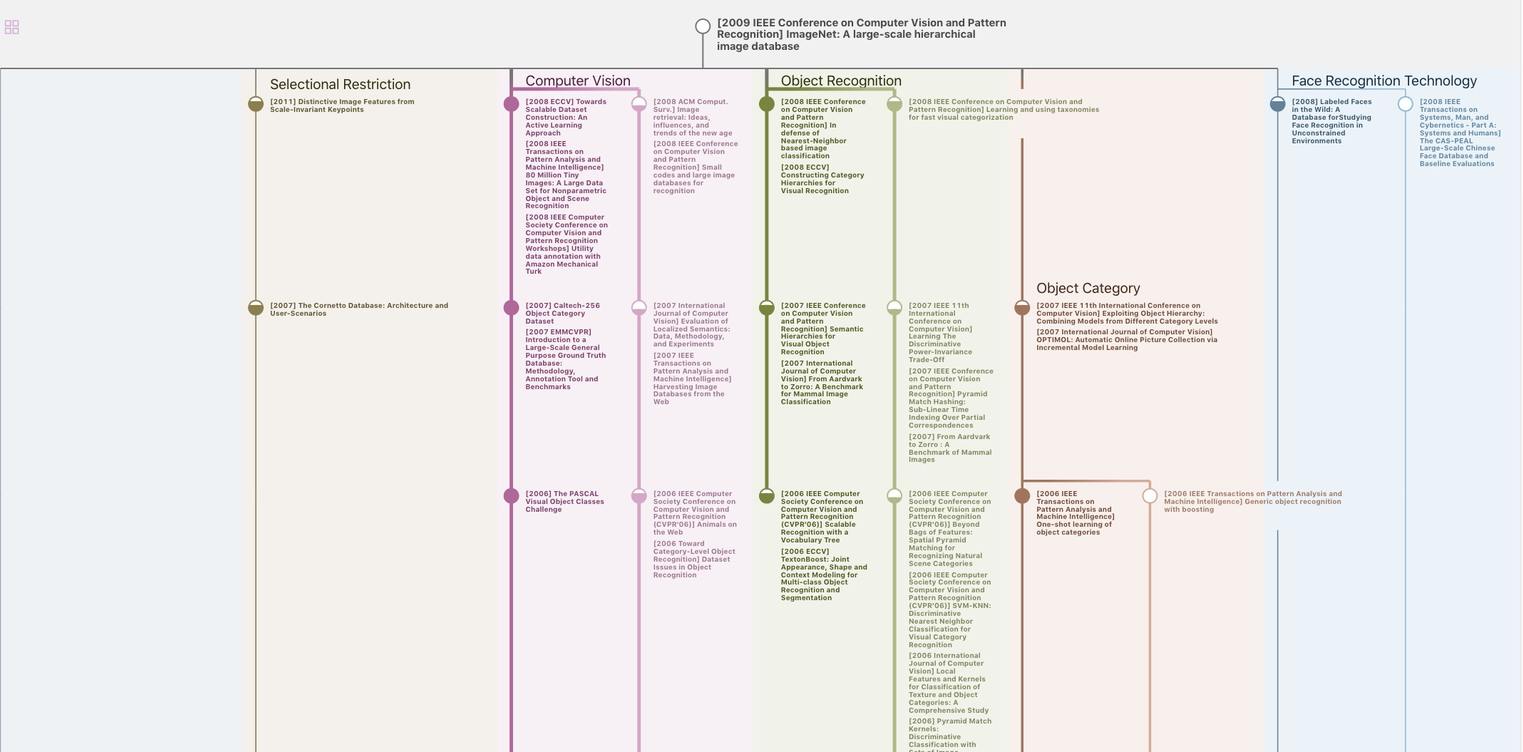
生成溯源树,研究论文发展脉络
Chat Paper
正在生成论文摘要