CARIS: Context-Aware Referring Image Segmentation
MM '23: Proceedings of the 31st ACM International Conference on Multimedia(2023)
Abstract
Referring image segmentation aims to segment the target object described by a natural-language utterance. Recent approaches typically distinguish pixels by aligning pixel-wise visual features with linguistic features extracted from the referring description. Nevertheless, such a free-form description only specifies certain discriminative attributes of the target object or its relations to a limited number of objects, which fails to represent the rich visual context adequately. The stand-alone linguistic features are therefore unable to align with all visual concepts, resulting in inaccurate segmentation. In this paper, we propose to address this issue by incorporating rich visual context into linguistic features for sufficient vision-language alignment. Specifically, we present Context-Aware Referring Image Segmentation (CARIS), a novel architecture that enhances the contextual awareness of linguistic features via sequential vision-language attention and learnable prompts. Technically, CARIS develops a context-aware mask decoder with sequential bidirectional cross-modal attention to integrate the linguistic features with visual context, which are then aligned with pixel-wise visual features. Furthermore, two groups of learnable prompts are employed to delve into additional contextual information from the input image and facilitate the alignment with non-target pixels, respectively. Extensive experiments demonstrate that CARIS achieves new state-of-the-art performances on three public benchmarks. Code is available at https://github.com/lsa1997/CARIS.
MoreTranslated text
AI Read Science
Must-Reading Tree
Example
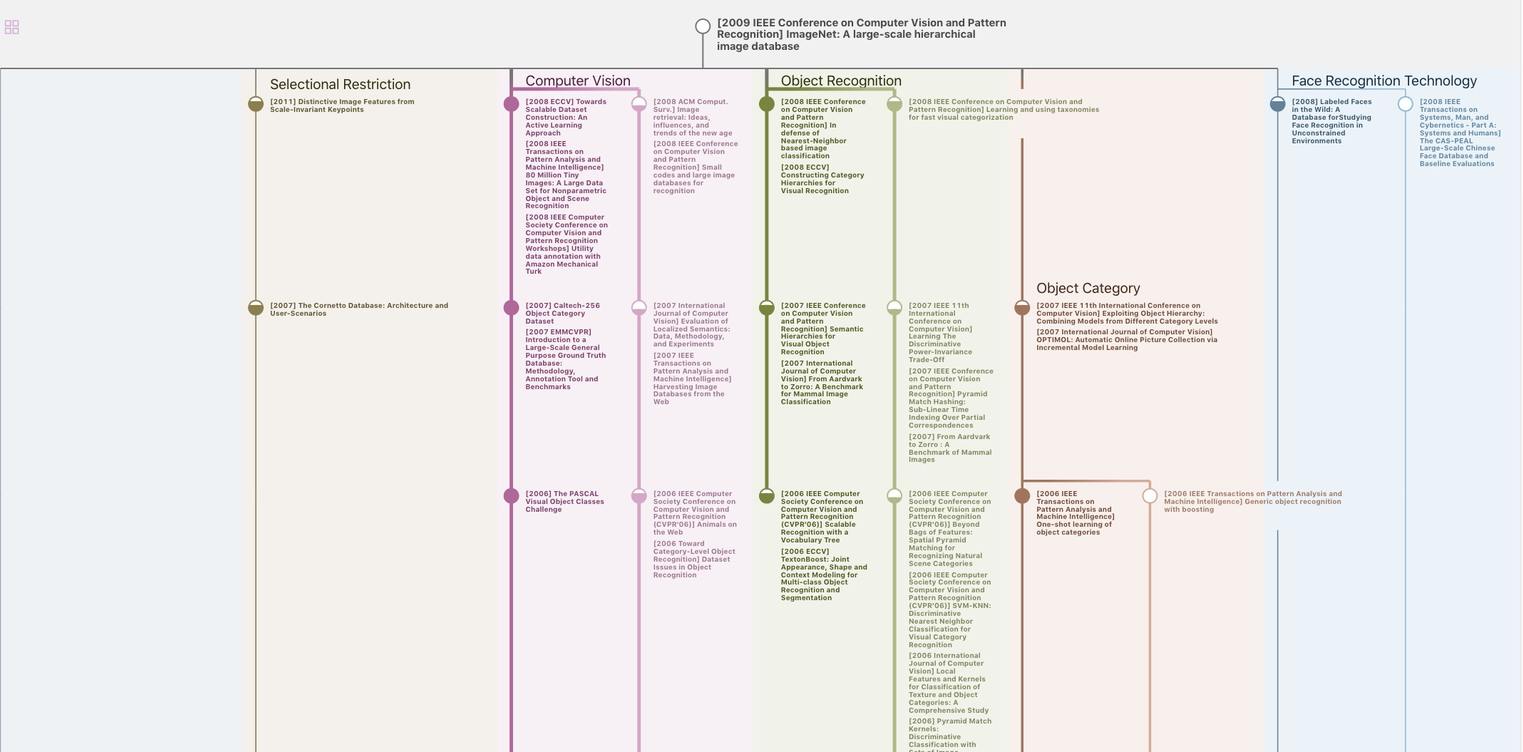
Generate MRT to find the research sequence of this paper
Chat Paper
Summary is being generated by the instructions you defined