Unknown Fault Detection of Rolling Bearing Based on Similarity Mining of Stationary and Non-stationary Features
PROCEEDINGS OF THE 4TH INTERNATIONAL WORKSHOP ON HUMAN-CENTRIC MULTIMEDIA ANALYSIS, HCMA 2023(2023)
摘要
Rolling bearings are essential and critical components in rotating machinery, and their condition is vital for ensuring the reliable operation of the equipment. Currently, numerous deep learning methods have been investigated for diagnosing the condition of bearings. However, these methods can only recognize fault categories that were included in the training of the model, and they are unable to detect unknown fault categories. In this paper, we propose a method for unknown fault detection of rolling bearings based on stationary - non-stationary feature similarity mining. This method analyzes the features of fault signals and explores the relationships between various fault categories, considering both stationary and non-stationary perspectives. In particular, we propose a framework for bearing unknown fault detection based on stationary and non-stationary feature mining. The framework comprises two stages: pre-training and the discovery of unknown fault categories. Siamese networks are employed during the pre-training stage to acquire the similarity relationship between stationary and non-stationary features. During the stage of discovering unknown fault categories, the similarity information is utilized to facilitate the identification of new fault categories. Additionally, we introduce a discriminative feature time-frequency focusing module to prioritize the model's attention towards non-stationary time-frequency fluctuations. The performance of our model is evaluated on the CWRU dataset and the PU dataset, and the experimental results demonstrate its superiority over other state-of-the-art methods, resulting in a significant enhancement in accuracy.
更多查看译文
关键词
fault diagnosis,novel class discovery,similarity mining,attention mechanism
AI 理解论文
溯源树
样例
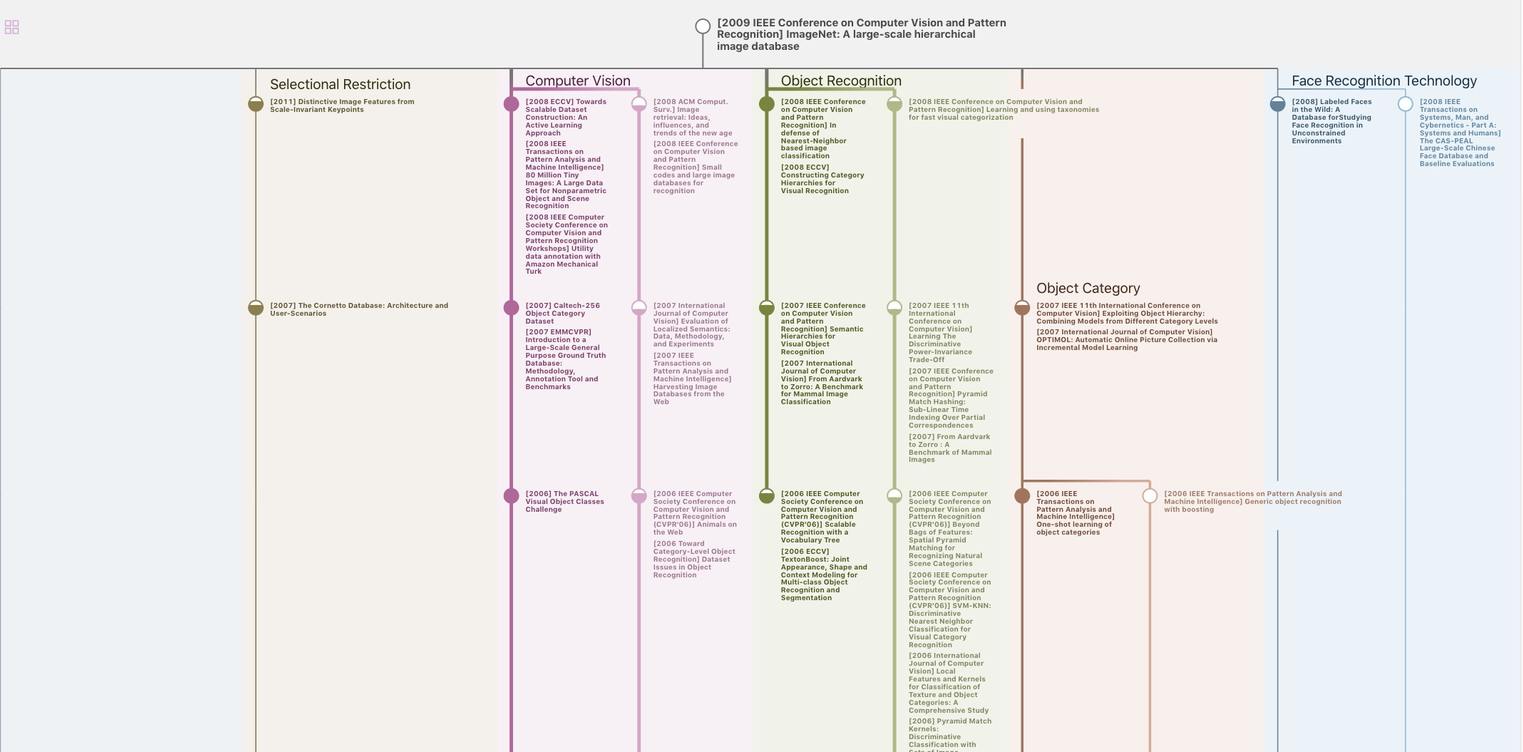
生成溯源树,研究论文发展脉络
Chat Paper
正在生成论文摘要