An intelligent computer-aided diagnosis method for paroxysmal atrial fibrillation patients with nondiagnostic ECG signals
BIOMEDICAL SIGNAL PROCESSING AND CONTROL(2024)
摘要
Classification of ECG signals plays an important role in the field of medical diagnosis. Despite that much progress has been made in ECG classification in recent years, most of them focus on single-lead cardiac feature extraction. In this paper, we propose a new classification method based on twelve-lead surface ECG signal by fusing improved hand-crafted ECG features and deep learning classifiers for paroxysmal atrial fibrillation (PAF) detection. Three-dimensional dominated components are extracted without ECG signal segmentation operations, and continuous wavelet transform (CWT) is adopted for time-frequency representation to reflect essential characteristics of multi-resolution frequency domain at different scales. This kind of time-frequency representation has high discriminative power to detect PAF even before pathologic changes in surface twelve-lead ECG signals. Five pre-trained deep neural networks models are used to perform transfer learning and feature fusion. ECG signals are finally classified by using a feature fusion method based on multiple deep networks.
更多查看译文
关键词
ECG classification,KPCA-CWT,Feature fusion,Multiple deep networks
AI 理解论文
溯源树
样例
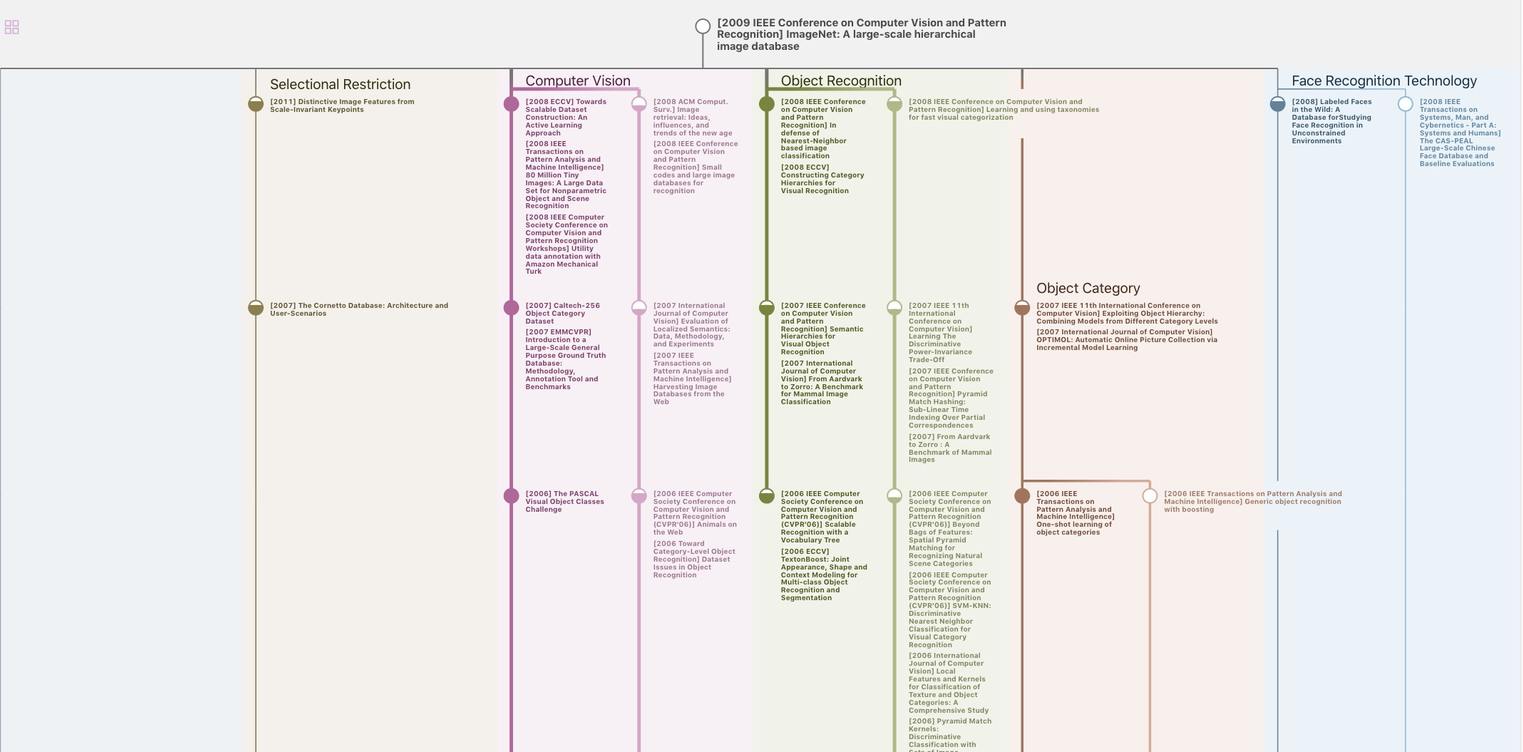
生成溯源树,研究论文发展脉络
Chat Paper
正在生成论文摘要