Continual Learning Under Language Shift
CoRR(2023)
摘要
The recent increase in data and model scale for language model pre-training
has led to huge training costs. In scenarios where new data become available
over time, updating a model instead of fully retraining it would therefore
provide significant gains. We study the pros and cons of updating a language
model when new data comes from new languages – the case of continual learning
under language shift. Starting from a monolingual English language model, we
incrementally add data from Danish, Icelandic, and Norwegian to investigate how
forward and backward transfer effects depend on pre-training order and
characteristics of languages, for three different model sizes. Our results show
that, while forward transfer is largely positive and independent of language
order, backward transfer can be positive or negative depending on the order and
characteristics of new languages. We explore a number of potentially
explanatory factors and find that a combination of language contamination and
syntactic similarity best fits our results.
更多查看译文
关键词
continual learning,language shift
AI 理解论文
溯源树
样例
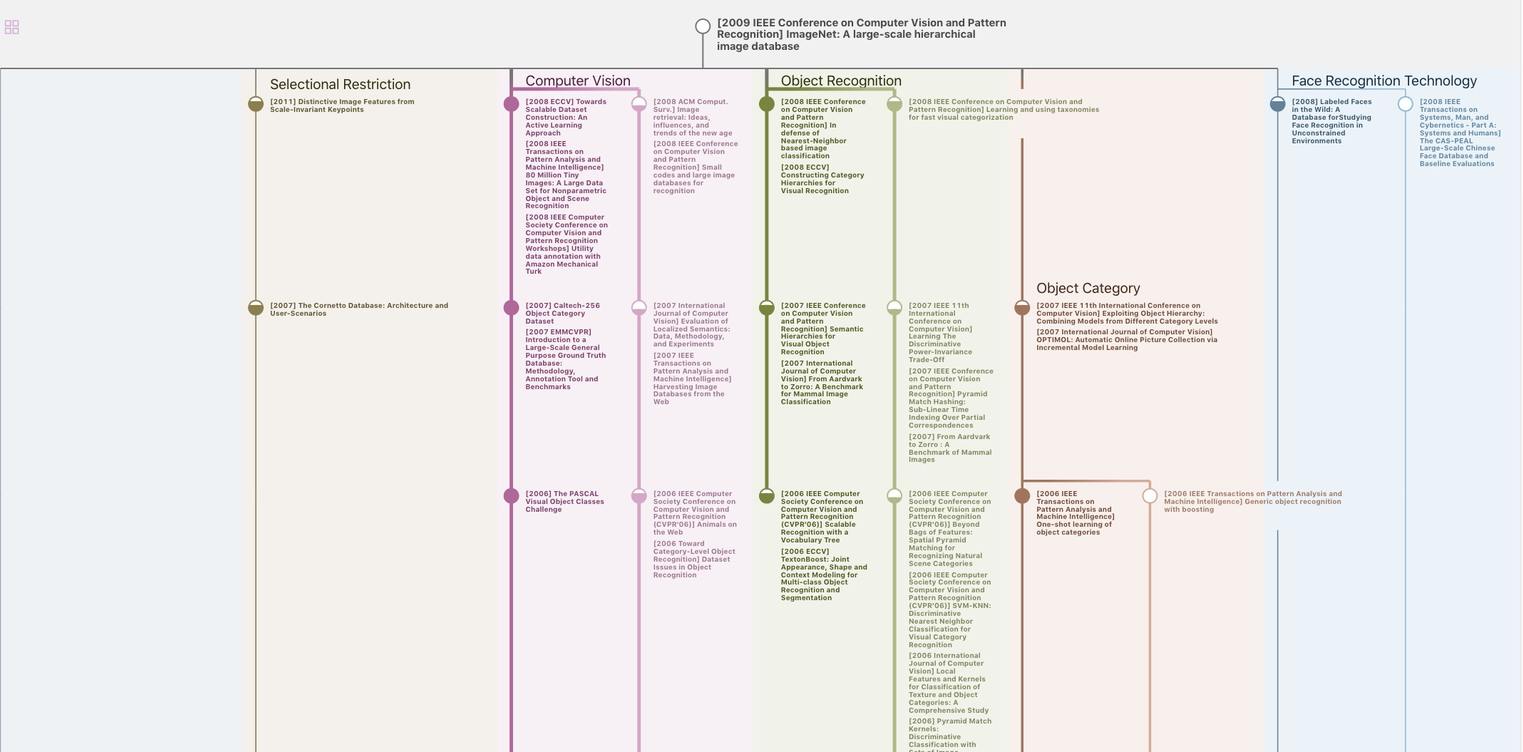
生成溯源树,研究论文发展脉络
Chat Paper
正在生成论文摘要