ResTran: Long Distance Relationship on Image Forgery Detection
IEEE Access(2023)
摘要
Image tampering detection is becoming more and more important in image forensics, especially in today's society where advanced image editing tools are becoming more and more popular. At the same time, with the rapid growth of digital image technology and multimedia data, it is very important to ensure the authenticity and integrity of visual information. Therefore, to address this issue, we propose a novel image tampering detection method using a deep convolutional network (ResNet) combined with a Transformer decoding layer. While traditional algorithms only solve a single tamper detection problem, our method can detect multiple tampering means. We use ResNet as the backbone of feature extraction to obtain rich and hierarchical features from input images. We then employ Transformer's decoding layer to process these features, enabling the model to capture long-distance dependencies and complex patterns, which we believe can further improve the accuracy of image tampering detection. In the experiments, we conduct experiments on three datasets (CASIA, NIST, and IMD2020) to verify the performance of the model. According to the experimental results, our model performed well on the CASIA and IMD2020 datasets, and also achieved good results in the NIST dataset. Furthermore, we also test two main types of image tampering: Copy-move and Splicing. Our model performs very well in detecting the Splicing type of image tampering. The experimental results show that this study fully demonstrates the potential and effectiveness of deep convolutional networks (such as ResNet) and Transformer decoding layers in image tampering detection, and also verifies the high performance and excellent generalization ability of the model.
更多查看译文
关键词
Transformer,image forgery detection,ResNet,splicing,copy-move,deep learning
AI 理解论文
溯源树
样例
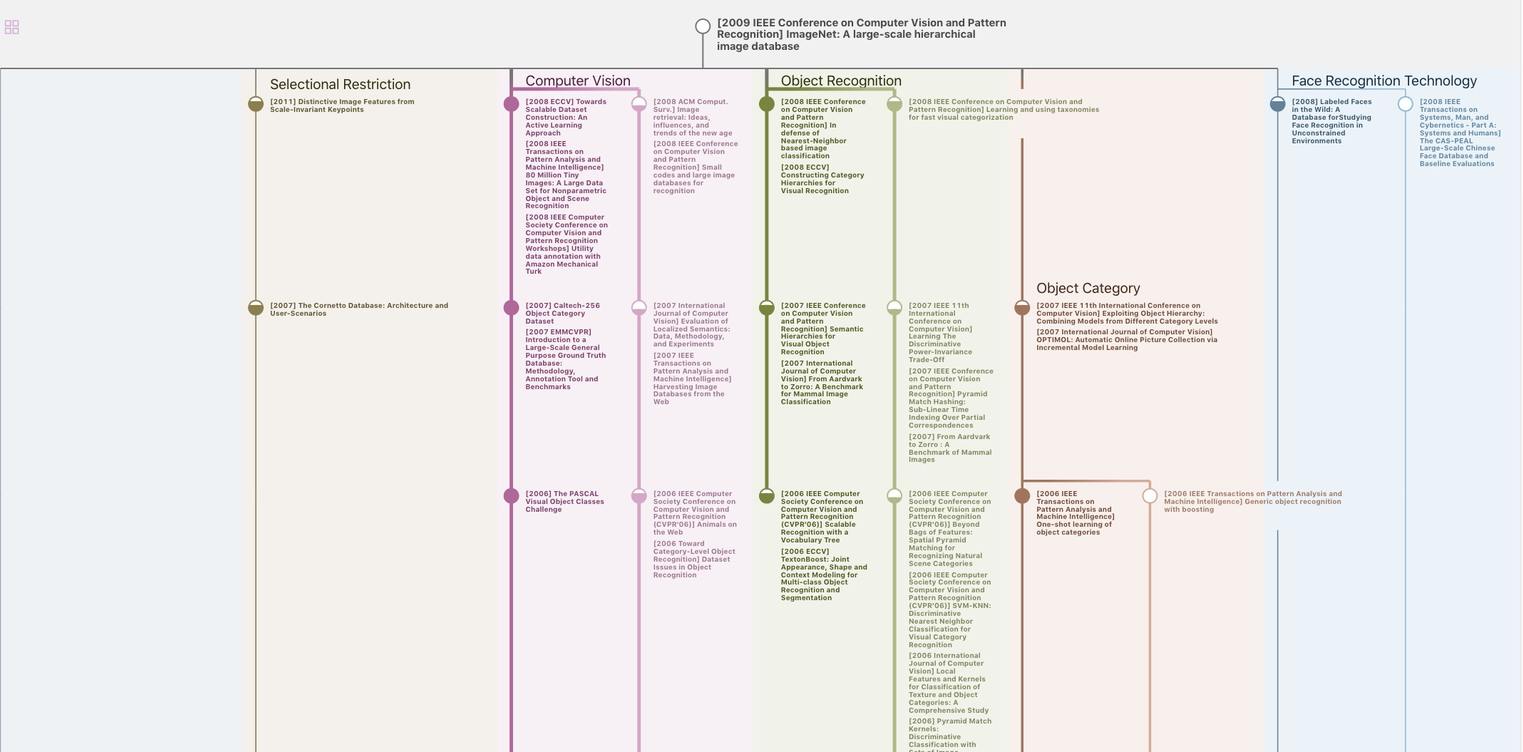
生成溯源树,研究论文发展脉络
Chat Paper
正在生成论文摘要