Mapping Exploit Code on Paste Sites to the MITRE ATT&CK Framework: A Multi-label Transformer Approach
2023 IEEE International Conference on Intelligence and Security Informatics (ISI)(2023)
摘要
Cyber-criminals often use information-sharing platforms such as paste sites (e.g., Pastebin) to share vast amounts of malicious text content, such as exploit source code. Careful analysis of malicious paste site content can provide Cyber Threat Intelligence (CTI) about potential threats. In this research, we propose a Convolutional BiLSTM Transformer multi-label classification method that automatically maps paste site exploit source code to the MITRE ATT&CK framework to identify adversarial techniques in support of proactive CTI. The Convolutional BiLSTM Transformer combines a convolutional neural network layer placed before a Transformer block, a concatenated pooling from a global max pooling and global average, and a BiLSTM pair-wise function within the Transformer to capture word and sequence orders. We conducted an multi-label classification experiment where our proposed Convolutional BiLSTM Transformer model achieved state-of-the-art results in terms of accuracy, recall, F1-score, and hamming loss. The results of a case study showed the tactics and tools that are used by malicious actors on paste sites.
更多查看译文
关键词
Cyber threat intelligence,paste sites,exploit linking,convolutional,BiLSTM,transformer model,exploits
AI 理解论文
溯源树
样例
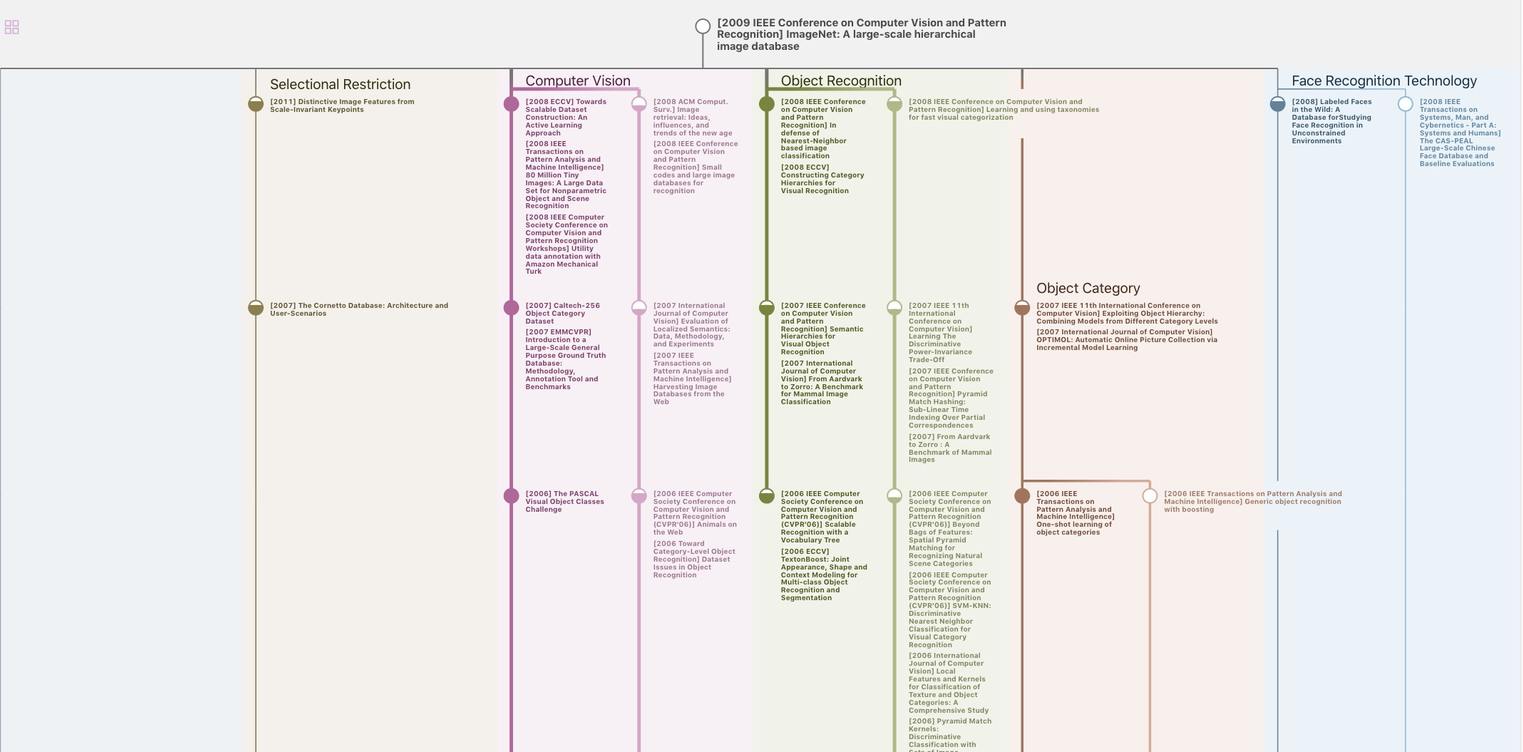
生成溯源树,研究论文发展脉络
Chat Paper
正在生成论文摘要