A Deep Reinforcement Learning Perspective on Adaptive Federated Dropout
2023 IEEE 20th International Conference on Mobile Ad Hoc and Smart Systems (MASS)(2023)
摘要
Federated learning is a distributed learning solution that achieves high-quality machine learning models while ensuring privacy and collaboration among various end devices. However, different kinds of end devices can lead to an unstable training process due to limited and dynamic communication and computation resources. Federated Dropout is an important technique for mitigating such resource bottlenecks by randomly removing a fixed percentage of activations of model components. However, most of the existing FL dropout methods cannot effectively utilize the distinguished characteristics of different clients or fail to automatically adapt to end device heterogeneity. In this paper, we thus propose Adaptive Federated Dropout with Reinforcement Learning (AFD-RL). AFD-RL employs reinforcement learning technology to adapt to the clients’ unbalanced computation and communication resources and provide a personalized dropout strategy for each client. By automatically selecting suitable model components for each client in each communication round, AFD-RL achieves better accuracy with fewer communication rounds. Extensive experiments validate the effectiveness of the proposed approach in improving training efficiency and inference accuracy.
更多查看译文
关键词
federated learning,dropout,reinforcement learning,DDQN
AI 理解论文
溯源树
样例
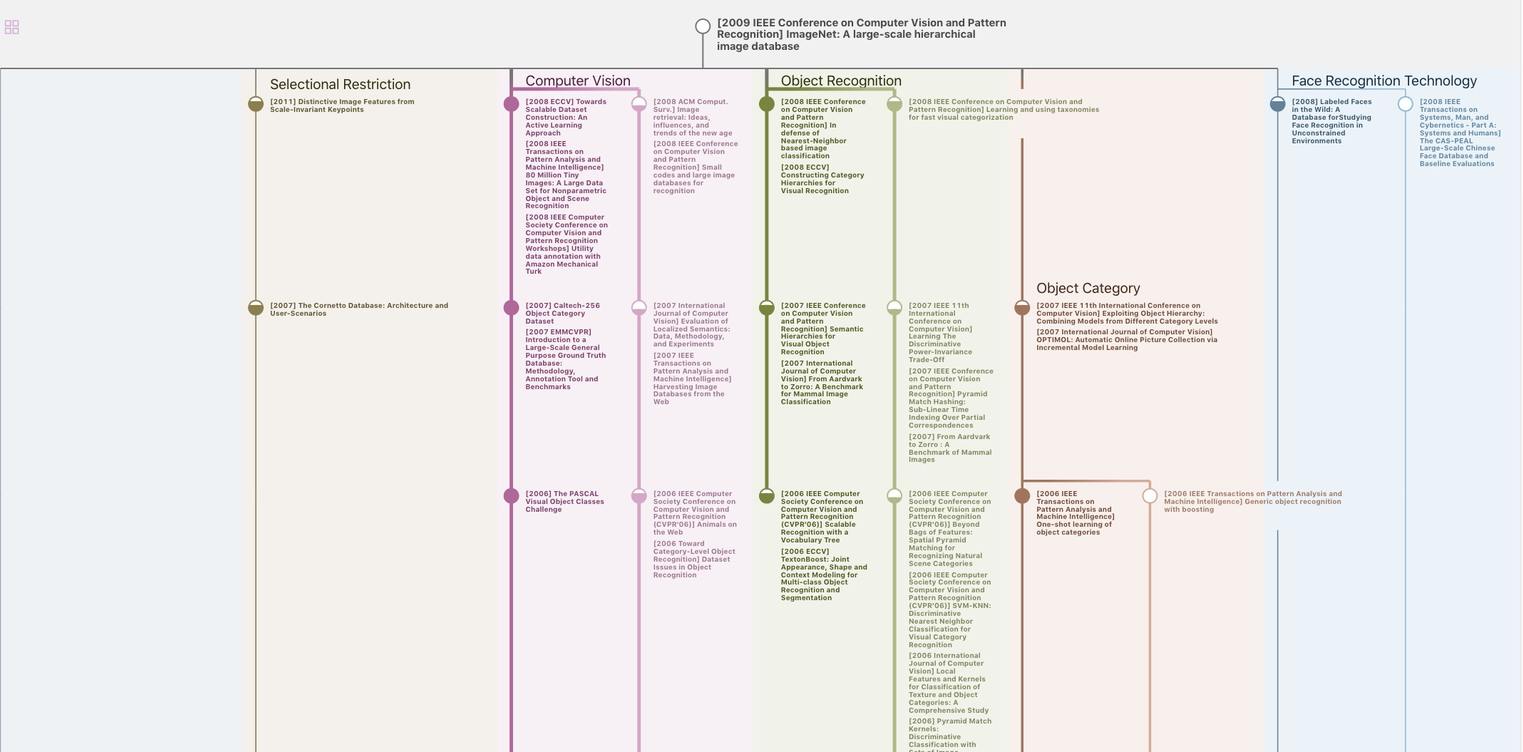
生成溯源树,研究论文发展脉络
Chat Paper
正在生成论文摘要