Time-Series Misalignment Aware DNN Adversarial Attacks for Connected Autonomous Vehicles
2023 IEEE 20th International Conference on Mobile Ad Hoc and Smart Systems (MASS)(2023)
Abstract
Connected Autonomous Vehicle (CAV) technique allows each autonomous vehicle to analyze time-series driving signals (e.g., speed, steering wheel angle) sent by its nearby vehicle using the Deep Neural Network (DNN). This scenario could attract adversarial attacks on the DNNs. To fool the victim's DNN model to make misclassification in the black-box scenario, an attacker generates a small perturbation series on time-series signals to generate an adversarial input. However, as the attacker does not know the start time stamp of each input to the DNN, the start point of the perturbation may not match that of real target input and the misalignment can make the attack unsuccessful. To address this problem, we propose three time-series Misalignment Aware DNN Adversarial Attacks (MA
3
) for CAVs. The first two methods estimate the start point of the target input, while the third method creates a perturbation for each maneuver that misclassifies all inputs with all time stamps as the start point. Both of our experimental evaluations based on real driving data and real experiments show that MA
3
produces up to 63.49% higher success rate, 56% less perturbation generation time, and 63.16% less perturbation amount compared with previous attack method. Our source code is publicly available.
MoreTranslated text
AI Read Science
Must-Reading Tree
Example
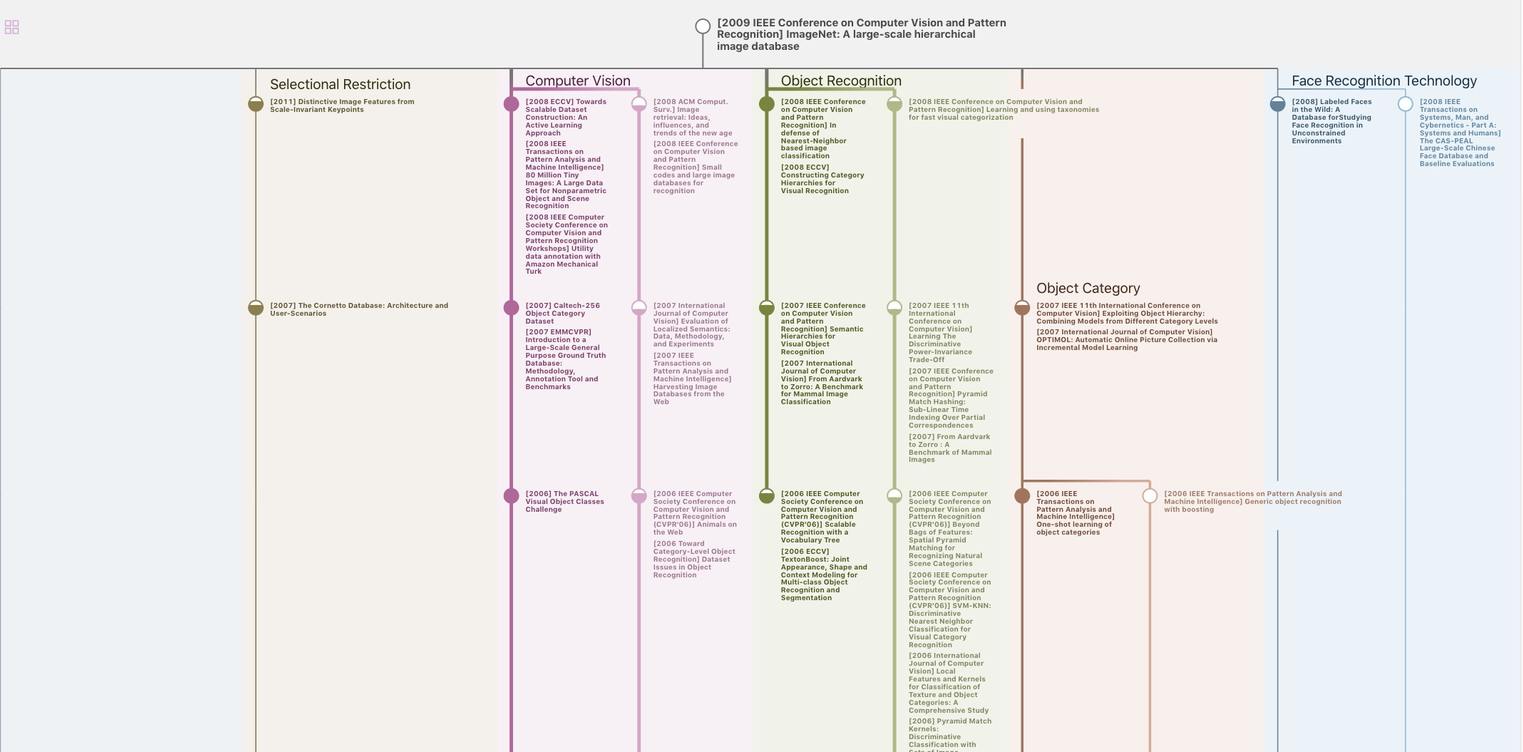
Generate MRT to find the research sequence of this paper
Chat Paper
Summary is being generated by the instructions you defined