GraphFed: A Personalized Subgraph Federated Learning Framework for Non-IID Graphs
2023 IEEE 20th International Conference on Mobile Ad Hoc and Smart Systems (MASS)(2023)
摘要
Recently, federated graph learning has attracted significant attention, as subgraphs of a global graph may often distribute across different institutions and are subject to privacy restrictions. However, inevitable data heterogeneity among sub-graphs can lead to poor performance of Graph Neural Networks (GNNs) trained by vanilla federated learning algorithm, which has been neglected before. To address this problem we look at subgraphs and find that there are usually overlapping nodes among them. Inspired by the common overlapping nodes among subgraphs, we propose GraphFed, a subgraph federated learning framework which solve the challenge in terms of both data augmentation and personalized federated learning. GraphFed uses common overlapping nodes among subgraphs to locate lost node information and applies data augmentation by retrieving lost nodes and forming common node sets that mitigates data distribution difference. Furthermore, GraphFed treats the overlapping nodes as bridges to calculate the distance among different subgraphs, which implies the similarity of the graph data distribution. Then, we use the distance to perform personalized federated learning. Empirical results and analysis on three real-world graph datasets with graph federated learning settings demonstrate the effectiveness of our proposed framework.
更多查看译文
关键词
federated learning,graph neural network,graph data heterogeneity
AI 理解论文
溯源树
样例
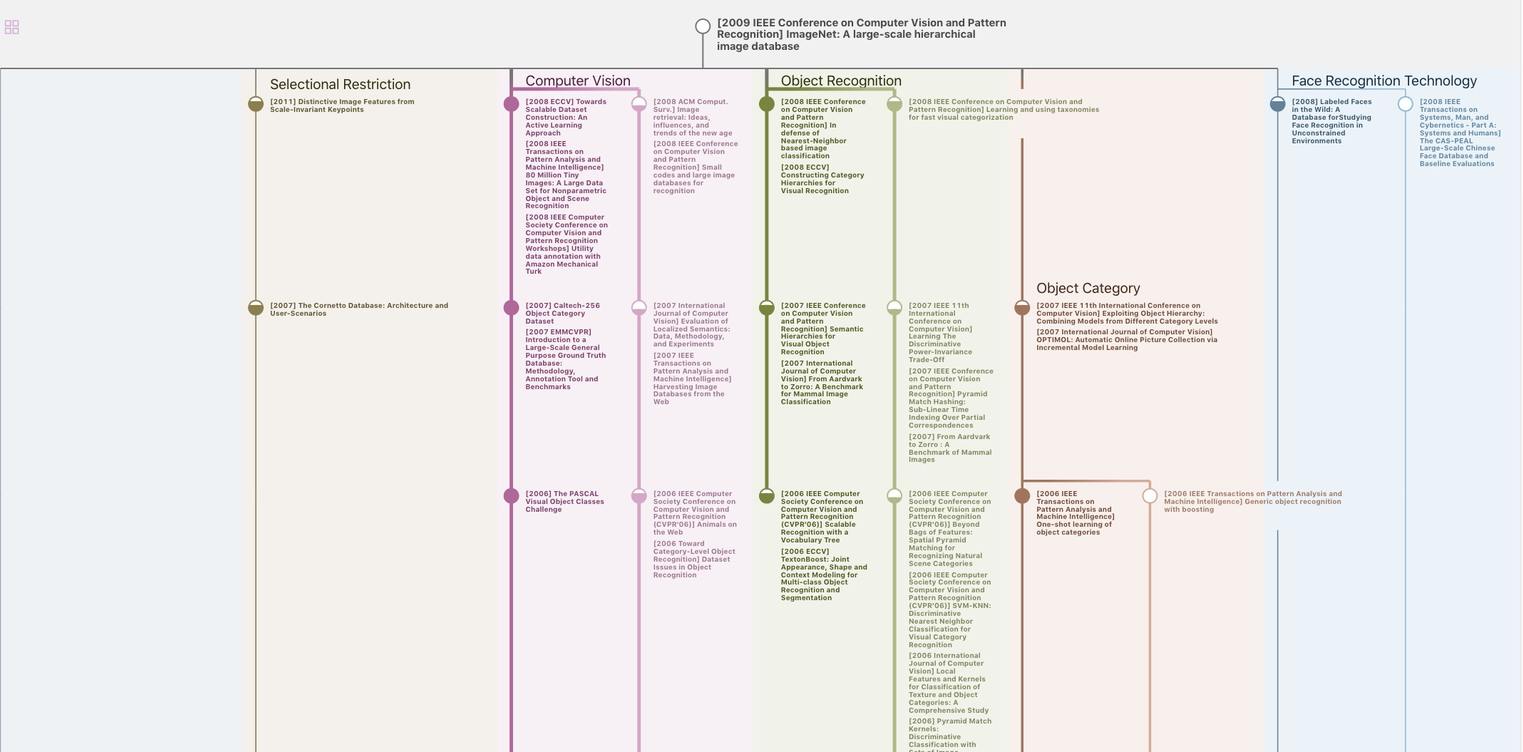
生成溯源树,研究论文发展脉络
Chat Paper
正在生成论文摘要